Title: Enhancing Geophysics Data Interpretation through a Human-in-the-Loop Framework
Time: Friday, Feb 17th, 3:00 PM
Location: CSIP library (room 5126), 5th floor, Centergy one building
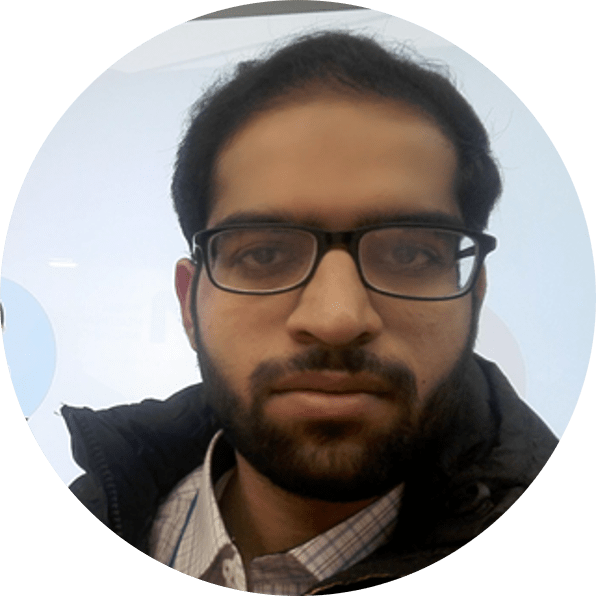
Bio
Ahmad is a 5th year PhD candidate at the Omni Lab for Intelligent Visual Engineering and Science (OLIVES) supervised by Professor Ghassan AlRegib. His work lies at the intersection of advanced machine/deep learning theory and practical applications in the domain of earth science. His work has received wide acclaim from peers in academia and industry alike and has been featured in various press releases at Georgia Tech. He has also made a name for himself for his teaching contributions and has been awarded the Outstanding ECE GTA Award and the Outstanding Online Head TA Award.
Abstract
Accurate and fast interpretation of geophysics data forms an important cornerstone of meeting the energy needs of our modern world. Over the years, researchers have devised various machine learning-based computational learning algorithms to improve turn-around times and accuracy of mapping energy deposits (i.e., oil and gas). However, geophysics data is beset with multiple problems including limited generalization capability, label uncertainty, feature redundancy, etc. Likewise, machine learning models trained on such data lack sufficient transparency to be able to induce user trust and do not fully exploit the unique characteristics of geophysics data with respect to certain critical applications. Additionally, the machine learning practitioner (i.e., the geophysicist) is usually relegated to a side role with little to no input to the data-driven model workflows. The presence of these limitations on multiple fronts (i.e., data, model, and user) hampers the realization of an integrated human-in-the-loop computational interpretation framework that allows for a smooth flow of information to and from the various pipeline components to extract the best value from the data. Our work has served to address these challenges in a systematic fashion to enable the possibility of a user-centric, data-driven, model-based interpretation framework for geophysics data.