DETAILS
61st Annual Meeting of the Association for Computational Linguistics
Toronto, Canada
July 9 – 14, 2023
Number of Authors by Subject ↓
Sorted by # of Georgia Tech Authors then Partners
Interactive Chart 📊
Resources and Evaluation
• • • • • • • • • • • • •
Theme Track: Reality Check
• • • • • • • • • • •
Multilingualism and Cross-Lingual NLP
• • • • • • • • • • • • • • • • • • • • • •
NLP Applications
• • • • • • • • • • • •
Generation
• • • • • • • •
Dialogue and Interactive Systems
• • • •
Speech and Multimodality
• • • • • • • • •
Information Retrieval and Text Mining
• • • • • •
Computational Social Science and Cultural Analytics
• • • • • • • • •
Machine Learning for NLP
• • • • • •
Interpretability and Analysis of Models for NLP
• • • •
Information Extraction
• • • •
Question Answering
• • • • • • • •
Ethics and NLP
• • • • •
Summarization
• • • • •
RESEARCH
Oral Papers
DiffusionDB: A Large-scale Prompt Gallery Dataset for Text-to-Image Generative ModelsHonorable Mention
Zijie J. Wang, Evan Montoya, David Munechika, Haoyang Yang, Benjamin Hoover and Duen Horng Chau
Towards Zero-Shot Multilingual Transfer for Code-Switched ResponsesOutstanding Paper
Ting-Wei Wu, Changsheng Zhao, Ernie Chang, Yangyang Shi, Pierce Chuang, Vikas Chandra and Biing Juang
Main Papers
Cold-Start Data Selection for Better Few-shot Language Model Fine-tuning: A Prompt-based Uncertainty Propagation Approach
Yue Yu, Rongzhi Zhang, Ran Xu, Jieyu Zhang, Jiaming Shen and Chao Zhang
Compositional Data Augmentation for Abstractive Conversation Summarization
Siru Ouyang, Jiaao Chen, Jiawei Han and Diyi Yang
Cross-Modal Attribute Insertions for Assessing the Robustness of Vision-and-Language Learning
Shivaen Ramshetty, Gaurav Verma and Srijan Kumar
DAMP: Doubly Aligned Multilingual Parser for Task-Oriented Dialogue
William Held, Christopher Hidey, Fei Liu, Eric Zhu, Rahul Goel, Diyi Yang and Rushin Shah
Distill or Annotate? Cost-Efficient Fine-Tuning of Compact Models
Junmo Kang, Wei Xu and Alan Ritter
Do CoNLL-2003 Named Entity Taggers Still Work Well in 2023?Reproduction Award
Shuheng Liu and Alan Ritter
Forgotten Knowledge: Examining the Citational Amnesia in NLP
Janvijay Singh, Mukund Rungta, Diyi Yang and Saif Mohammad
Human-in-the-loop Evaluation for Early Misinformation Detection: A Case Study of COVID-19 Treatments
Ethan Mendes, Yang Chen, Wei Xu and Alan Ritter
Improved Instruction Ordering in Recipe-Grounded Conversation
Duong Le, Ruohao Guo, Wei Xu and Alan Ritter
LENS: A Learnable Evaluation Metric for Text Simplification
Mounica Maddela, Yao Dou, David Heineman and Wei Xu
Multi-VALUE: A Framework for Cross-Dialectal English NLP
Caleb Ziems, William Held, Jingfeng Yang, Jwala Dhamala, Rahul Gupta and Diyi Yang
NormBank: A Knowledge Bank of Situational Social Norms
Caleb Ziems, Jane Dwivedi-Yu, Yi-Chia Wang, Alon Halevy and Diyi Yang
On Second Thought, Let’s Not Think Step by Step! Bias and Toxicity in Zero-Shot Reasoning
Omar Shaikh, Hongxin Zhang, William Held, Michael Bernstein and Diyi Yang
Revisiting non-English Text Simplification: A Unified Multilingual BenchmarkHonorable Mention
Michael Ryan, Tarek Naous and Wei Xu
Training Models to Generate, Recognize, and Reframe Unhelpful Thoughts
Mounica Maddela, Megan Ung, Jing Xu, Andrea Madotto, Heather Foran and Y-Lan Boureau
Trillion Dollar Words: A New Financial Dataset, Task & Market Analysis
Agam Shah, Suvan Paturi and Sudheer Chava
Findings Papers
Adversarial Robustness of Prompt-based Few-Shot Learning for Natural Language Understanding
Venkata Prabhakara Sarath Nookala, Gaurav Verma, Subhabrata Mukherjee and Srijan Kumar
Controllable Conversation Generation with Conversation Structures via Diffusion Models
Jiaao Chen and Diyi Yang
Extracting Shopping Interest-Related Product Types from the Web
Yinghao Li, Colin Lockard, Prashant Shiralkar and Chao Zhang
Frustratingly Easy Label Projection for Cross-lingual Transfer
Yang Chen, Chao Jiang, Alan Ritter and Wei Xu
Graph Reasoning for Question Answering with Triplet Retrieval
Shiyang Li, Yifan Gao, Haoming Jiang, Qingyu Yin, Zheng Li, Xifeng Yan, Chao Zhang and Bing Yin
Human-in-the-loop Abstractive Dialogue Summarization
Jiaao Chen, Mohan Dodda and Diyi Yang
Modeling Cross-Cultural Pragmatic Inference with Codenames Duet
Omar Shaikh, Caleb Ziems, William Held, Aryan Pariani, Fred Morstatter and Diyi Yang
Numeric Magnitude Comparison Effects in Large Language Models
Raj Shah, Vijay Marupudi, Reba Koenen, Khushi Bhardwaj and Sashank Varma
ReGen: Zero-Shot Text Classification via Training Data Generation with Progressive Dense Retrieval
Yue Yu, Yuchen Zhuang, Rongzhi Zhang, Yu Meng, Jiaming Shen and Chao Zhang
TADA : Task Agnostic Dialect Adapters for English
William Held, Caleb Ziems and Diyi Yang
Teaching the Pre-trained Model to Generate Simple Texts for Text Simplification
Renliang Sun, Wei Xu and Xiaojun Wan
Werewolf Among Us: Multimodal Resources for Modeling Persuasion Behaviors in Social Deduction Games
Bolin Lai, Hongxin Zhang, Miao Liu, Aryan Pariani, Fiona Ryan, Wenqi Jia, Shirley Anugrah Hayati, James Rehg and Diyi Yang
Industry Track
Context-Aware Query Rewriting for Improving Users’ Search Experience on E-commerce Websites
Simiao Zuo, Qingyu Yin, Haoming Jiang, Shaohui Xi, Bing Yin, Chao Zhang and Tuo Zhao
Workshops
- cTBL: Augmenting Large Language Models for Conversational Tables Anirudh Sundar, Larry Heck
- Keynote speakers: Larry Heck, Diyi Yang
- Keynote speaker: Alan Ritter
People
Main Program Paper Authors
Graduate Students
Jiaao Chen • Yang Chen • Yao Dou • Ruohao Guo • William Held • Wenqi Jia • Chao Jiang • Junmo Kang • Reba Koenen • Bolin Lai • Duong Le • Yinghao Li • Shuheng Liu • Mounica Maddela • Vijay Marupudi • Tarek Naous • Mukund Rungta • Fiona Ryan • Agam Shah • Raj Shah • Janvijay Singh • Gaurav Verma • Zijie J. Wang • Ting•Wei Wu • Haoyang Yang • Yue Yu • Rongzhi Zhang • Yuchen Zhuang • Caleb Ziems
Undergrads
Khushi Bhardwaj • David Heineman • Ethan Mendes • Evan Montoya • David Munechika • Aryan Pariani • Suvan Paturi • Michael Ryan
Alumni
Venkata Prabhakara Sarath Nookala • Shivaen Ramshetty
AI Sous Chef
Lorem ipsum dolor sit, amet consectetur adipisicing elit. Consequatur, ipsam enim vitae ratione illum nulla voluptatem quam ea nobis iste veniam. Veniam assumenda exercitationem et consequatur aliquam corporis, at eum?
Voluptatum nam, reiciendis aut dignissimos minima voluptatibus vel necessitatibus quas, aspernatur quo dolorum dolore mollitia dolor explicabo consectetur adipisci illo quisquam dolores cum libero quidem earum tempore alias facere. Voluptatem!
Aliquid maiores modi architecto asperiores cupiditate, fugit tempore alias delectus quisquam aperiam autem rerum accusamus voluptatibus enim eveniet magni illum aspernatur. Laudantium atque minus ullam, eligendi facilis quam voluptatem rerum!
Lorem, ipsum dolor sit amet consectetur adipisicing elit. Mollitia aut magnam, sit voluptas aperiam iusto unde possimus ipsam accusantium officia numquam cumque vel ex et animi ipsum facilis adipisci velit?

Lorem ipsum
Lorem ipsum dolor sit.
Lorem ipsum dolor sit amet consectetur adipisicing elit. Minus eligendi rerum recusandae temporibus nesciunt dicta saepe incidunt nostrum expedita ipsum.
Id, in maiores cumque repellat fugit, iusto at provident ullam illo incidunt quam quia iste sequi iure! Eveniet, sunt vero?
Lorem, ipsum dolor sit amet consectetur adipisicing elit. Minima, pariatur? Lorem, ipsum dolor sit amet consectetur adipisicing elit. Minima, pariatur.
Jane Doe

RESEARCH TEAM: Duong Le, Ruohao Guo, Wei Xu and Alan Ritter
Featured Research
Robustness: Making Progress by Poking Holes in Artificial Intelligence Modelsđź”—
By Bryant Wine
Every few weeks, a new consumer application powered by artificial intelligence (AI) hits the market. During this frenzy that is a gold rush of AI, many have come to question the technology because they feel it is untested and vulnerable to ailments ranging from buggy performance to adversarial surveillance.
That is one of many reasons the Association for Computational Linguistics (ACL) hosts an annual meeting, this year being the 61st to be held July 9-14 in Toronto. Here, the venue brings together experts from around the world to discuss advances, issues, and opportunities in natural language processing (NLP) and AI.
Two of these experts are Assistant Professor Srijan Kumar and Ph.D. student Gaurav Verma, both researchers at Georgia Tech’s School of Computational Science and Engineering (CSE). Kumar, Verma, and their group are slated to present two papers at ACL 2023, sharing their research about robustness of AI models that can lead to improved AI performance and security.
“Security of AI models is paramount. Reliable and responsible development of AI models are important discussion topics at the national and international levels,” Kumar said. “As Large Language Models become part of the backbone of many products and tools with which users will interact, it is important to understand when, how, and why these AI models will fail.”
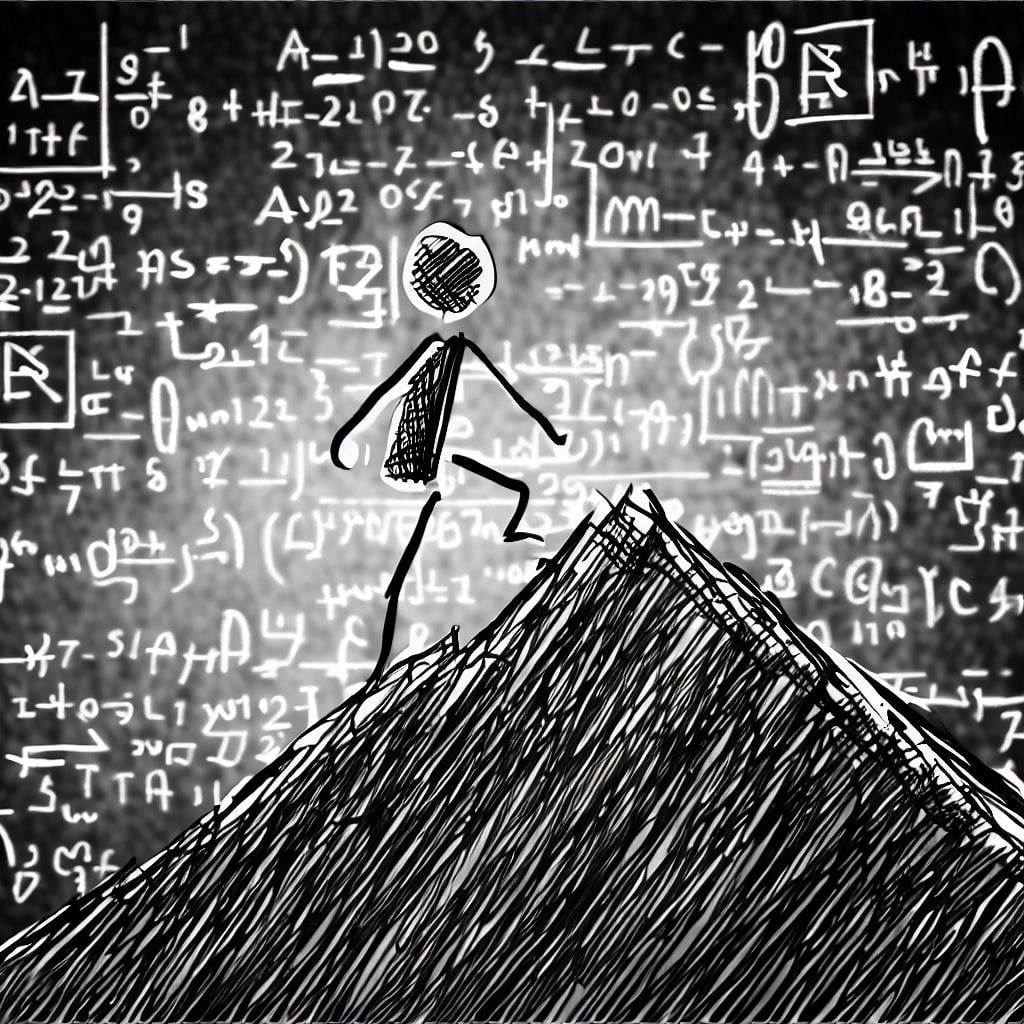
Robustness refers to the degree to which an AI model’s performance changes when using new data versus training data. To ensure that a model is performing reliably to its intended purpose, it is critical to understand their robustness.
Trust is the essential value within robustness, both for researchers that work in AI and consumers that use it.
AI models that perform unpredictably or in unintended way diminish trust in the technology, an issue relevant in today’s discourse and debate over AI security. Investigating robustness can prevent, or at least highlight, performance issues arising due to unmodeled behavior and malicious attacks.
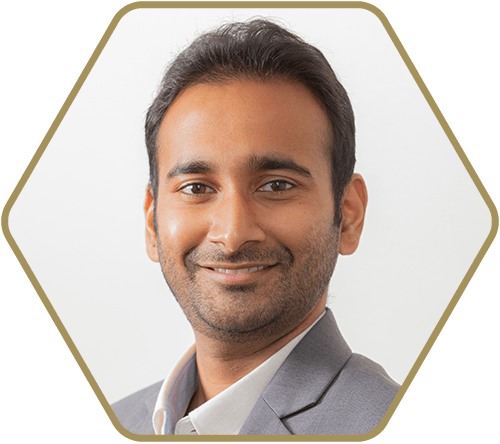
“Security of AI models is paramount. Reliable and responsible development of AI models are important discussion topics at the national and international levels. As Large Language Models become part of the backbone of many products and tools with which users will interact, it is important to understand when, how, and why these AI models will fail.”
Srijan Kumar
Assistant Professor
Computational Science and Engineering
Deep Learning for Every Kind of Media
The group proposes in their paper an approach to evaluate the robustness of multimodal learning methods called Cross-Modal Attribute Insertions (XMAI). XMAI obtains meaningful text augmentations using cross-modal associations in multimodal data, which they found methods using text-only information cannot do.
The revealed vulnerability, the group explains, is that augmenting input text leads to poorer performance of the multimodal model. For example, adding more descriptive wording in search text for an image, like from “girl on a chair” to “little girl on a wooden chair,” caused the correct image to be retrieved at a lower rank.
While this finding show that models fail, it is important to keep in mind the group’s research is part of the engineering process to evaluate and improve robustness in vision-and-language models. By discussing these flaws at conferences like ACL 2023, researchers can use shared information to build the next generation of more robust multimodal systems.
Similar to multimodal learning, another class of AI models that uses text as input is prompt-based few-shot learning (FSL). While FSL is a useful framework for AI to improve task performance where the availability of labeled data is limited, Kumar’s group points out in their ACL findings paper that there is limited understanding of the methods’ adversarial robustness.
“Our findings shine a light on a significant vulnerability in FSL models – a marked lack of adversarial robustness,” Verma explained. “Our work highlights a non-trivial balancing act between accuracy and adversarial robustness of few-shot learning for NLP.”
Kumar’s team ran tests on six GLUE benchmark tasks, comparing FSL models with fully fine-tuned models. Here, they found a notable, greater drop in task performance of FSL models treated with adversarial perturbations than that of fully fine-tuned models.
In the same study, Kumar’s group found and proposed few ways to improve FSL robustness.
These include using unlabeled data for prompt-based FSLs and expanding to an ensemble of models trained with different prompts. The group also demonstrated that increasing the number of few-shot examples and model size led to increased adversarial robustness of FSL methods.
Co-authors joining Kumar and Verma in their two ACL 2023 papers include School of CSE alumni Shivaen Ramshetty and Venkata Prabhakara Sarath Nookala, as well as Subhabrata Mukherjee, a principal researcher at Microsoft Research.
Kumar’s lab, the Computational Data Science Lab for the Web and Social Media (CLAWS), lies at the intersection of AI and security, where they develop solutions to improve the security of AI models and AI solutions to improve internet security.
Kumar is like many today in that he wants to realize the potential good of AI. Here, measuring and enhancing the trustworthiness and robustness of AI models are cornerstones of the CLAWS group, ensuring the security of AI and the safety of those that use it.
“My vision for future AI models is for them to not only be accurate, but also robust, reliable, trustworthy, unbiased, and fair,” said Kumar. “These two ACL research papers, along with our other recent papers, push the scientific boundaries in the development of the next-generation of AI models.”

More News
The Big Picture

ACL’s global community in the 2023 technical program includes 6,600+ experts from more than 60 countries.
Explore this interactive data dashboard showing which countries are in the different research tracks at ACL.
This analysis was developed by the Machine Learning Center at Georgia Tech in conjunction with the ACL organizing committee.
Project Lead and Web Development: Joshua Preston
Writers: Tess Malone, Bryant Wine
Data Interactives: Joshua Preston
Special Thanks to: Yang Liu