Learning @ Scale 2024
ACM Learning at Scale Conference | July 18 – 20, 2024 | ATLANTA

Rapid advances in AI have created new opportunities but also challenges for the Learning@Scale community. The advances in generative AI show potential to enhance pedagogical practices and the efficacy of learning at scale. This has led to an unprecedented level of interest in employing generative AI for scaling tutoring and feedback. The prevalence of such tools calls for new practices and understanding on how AI-based methods should be designed and developed to enhance the experiences and outcomes of teachers and learners.
Learn how Georgia Tech experts are tackling this challenge with research at the ACM Learning at Scale Conference 2024.
Robotics research is part of the next wave of computing innovation and is key to shaping how artificial intelligence will become part of our lives. Meet the Georgia Tech experts who are charting a path forward. #ICRA2024
____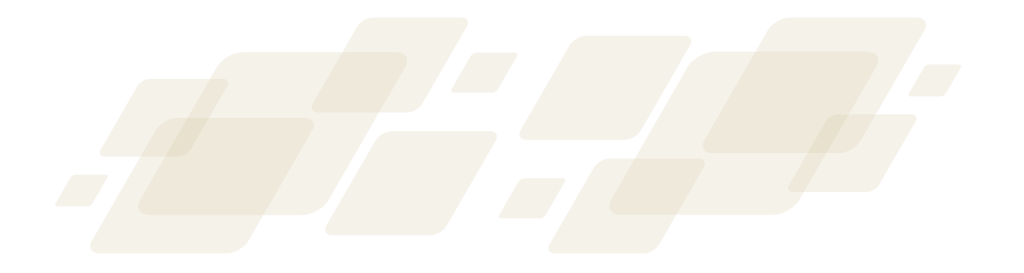
By the Numbers
*includes two partner authors
Partner Organizations
Adobe • Allen Institute for Artificial Intelligence • Amazon • Beijing University of Aeronautics and Astronautics • Carnegie Mellon University • Georgia Tech • Google • IBM • IIIT-Hyderabad • Indiana University • King Abdullah University of Science and Technology (KAUST) • Koc University • Lepton AI Inc. • Massachusetts Institute of Technology • Meta • Microsoft Research • Mohamed bin Zayed University of Artificial Intelligence • National University of Singapore • NVIDIA • Picsart AI Research • Princeton University • Qualcomm Inc • Reblink • Simon Fraser University • Snap Inc • Stanford University • State University of New York – Binghamton • State University of New York – Buffalo • Tel Aviv University • Terminus Group • Torc Robotics • Tsinghua University • Universidad de Los Andes • University of Bristol • University of British Columbia • University of California – Berkeley • University of California – Los Angeles • University of Catania • University of Illinois – Urbana-Champaign • University of Louisiana – Lafayette • University of Maryland – College Park • University of Minnesota • University of North Carolina – Chapel Hill • University of Oxford • University of Pennsylvania • University of Science and Technology of China • University of Texas – Austin • University of Tokyo • University of Trento • Virginia Polytechnic Institute and State University • Zhejiang University
Topics with Georgia Tech
- 3D from multi-view and sensors
- 3D from single images
- Datasets and evaluation
- Efficient and scalable vision
- Embodied vision: Active agents, simulation
- Humans: Face, body, pose, gesture, movement
- Image and video synthesis and generation
- Multi-modal learning
- Recognition: Categorization, detection, retrieval
- Robotics
- Scene analysis and understanding
- Segmentation, grouping and shape analysis
- Transfer, meta, low-shot, continual, or long-tail learning
- Transparency, fairness, accountability, privacy, ethics in vision
- Vision applications and systems
- Vision, language, and reasoning
Faculty 🔗
The Big Picture 🔗

Georgia Tech Experts and Partners
Georgia Tech’s 17 research contributions to the technical program include two research papers, 14 work-in-progress papers, and one workshop. Experts represent the College of Computing’s School of Interactive Computing, School of Computing Instruction, and the Online Master of Science in Computer Science degree program (OMSCS), as well as the School of Psychology, and Institute for Data Engineering and Science.
The ACM Learning @ Scale 2024 Conference is convening experts for its 10th annual gathering. This year also marks the 10th anniversary of the OMSCS program at Georgia Tech, with more than 10,000 graduates and a spring enrollment of 13,600 online students.
Search for experts in the chart and interact with the chart to highlight connections of interest. Explore more now.
Faculty Highlights
Thirteen academic and research faculty have research accepted in Learning @ Scale 2024. Among the faculty, four are lead authors on research contributions and two are included in multiple parts of the technical program:
- Keith Adkins and David Joyner are the leads on one research paper each; Alex Duncan leads three works-in-progress (WIPs) while Chaohua Ou leads one WIP.
- David Joyner and Thad Starner are both in multiple parts of the technical program. Joyner has seven contributions, two papers and five WIPs. Starner has five contributions, including four WIPs and a workshop.
- The Online Master of Science in Computer Science program and the School of Interactive Computing have the most Tech faculty in the program, with four each. Interact with the chart above for details.

Global Program
Learning @ Scale includes research with the aim of improving the experiences and outcomes of learners, teachers, and educators. This year a central theme is exploring the technological, social, and cultural aspects of the responsible use of AI in scaling learning.
The conference includes more than 300 experts from 90 organizations that span the globe, with 21 countries represented.
The chart shows teams, with one row representing a team and all the authors numbered. The dots indicate the first expert for each organization on a team.
Hover on chart elements to highlight topics of interest. Use the search bars to find team members based on organization, country, or name. Check out the Georgia Tech work here!


Educational Data Mining 2024 co-located with Learning @ Scale
The 17th International Conference on Educational Data Mining (EDM 2024) is July 14–17 in Atlanta.
Educational Data Mining is an emerging discipline, concerned with developing methods for exploring the unique and increasingly large-scale data that come from educational settings and using those methods to better understand students, and the settings which they learn in.
Explore the main program of EDM, including Georgia Tech’s three research contributions.
News 🔗

Learning at Scale: Researchers Examine the Evolution of Affordable At-Scale Degree
By Emily Smith
As Georgia Tech’s Online Master of Science in Computer Science (OMSCS) program marks its 10th anniversary, College of Computing’s David Joyner and Alex Duncan are examining key trends in the groundbreaking program that has transformed graduate education.
Their research paper, “Ten Years, Ten Trends: The First Decade of an Affordable At-Scale Degree” will be presented at the 2024 Learning @ Scale Conference, hosted at Georgia Tech.
Launched in 2014, the OMSCS program used Massive Open Online Course (MOOC) platforms to offer an affordable, high-quality graduate degree in Computer Science (CS), making advanced education accessible globally by overcoming cost and geographic barriers.
DevOps Methods in Machine Learning Courses Drastically Reduce Bugs in Assignments
New Research Helps to Scale CS Education for Large Classes
Teaching staff in Georgia Tech’s CS4641 and CS7641 machine learning courses have taken a significant step toward improving student experiences by integrating DevOps methodologies into the courses to reduce errors in large, complex assignments.
DevOps integrates and automates the work of software development and information technology operations as a means for improving and shortening the systems development life cycle.
“With surging enrollments, managing and scaling these courses effectively has become crucial,” said Max Mahdi Roozbahani, faculty member in the School of Computing Instruction and contributor to the effort.
Expand
The new approach involves the adoption of Continuous Integration and Continuous Deployment (CI/CD) pipelines along with GitFlow to streamline assignment validation and delivery.
In the machine learning courses, students tackle complex assignments designed to deepen their understanding of ML algorithms and their applications. However, managing such extensive coursework often led to bugs that could disrupt the learning process. To address this, the team of 12 teaching assistants (TAs) and Roozbahani, the instructor, implemented a CI/CD pipeline that automates the validation of assignments from creation to release. This system ensures that assignments are thoroughly tested, reducing the number of bugs and enhancing the overall student experience.
“The School of Computing Instruction is proud to be the first post-secondary organization in the country, or even internationally, to use DevOps for large classes,” said Roozbahani. “Our approach prepares and locally tests assignments to improve the student experience and avoid human-made mistakes.
“The efforts have yielded remarkable results. An analysis of assignment-related issues revealed a 57.22% decrease in bugs for assignments. This significant reduction highlights the effectiveness of integrating DevOps practices into the educational framework.”
Implementing DevOps methodologies in the ML courses has not only streamlined the assignment process but also allowed instructors to provide a more consistent and engaging learning environment for students.
The method is detailed in new research that Roozbahani said helps Georgia Tech remain at the forefront of educational innovation, “ensuring that its students receive top-tier education in the rapidly evolving field of machine learning.”
The work, titled Transforming CS Education with DevOps: Streamlined Assignment Validation and Delivery @ Scale, will be presented in July at the ACM Conference on Learning at Scale in Atlanta. Co-authors on the paper include Gururaj Deshpande, Shravan Cheekati, Shail Patel, Pranav Raj, Madhuri Singh, Mark Pindur, Nouf Al Soghyar, Bryan Zhao, Parisa Babolhavaeji, Mohammad Taher, Krish Nathan, Will Spaeth, and Max Mahdi Roozbahani.

Research 🔗
Paper
New Coding Assignments and the First Repository Effect on Inter-Semester Plagiarism
Keith Adkins and David Joyner
“The Internet—for all if its benefits—makes it easy for students to share assignments, creating a problem for academic institutions. Common mitigation tactics include discouraging students from sharing their work and routinely checking for and removing solutions shared online. While this can be successful in many cases, it is not always. In our experience, it can be a challenge if either students or hosting sites refuse to remove solutions. Pursuing legal options can be both time consuming and costly. One approach taken to combat this is to routinely create new coding assignments. Yet this can require a significant time commitment. It is worth exploring if this effort is worthwhile.
In this paper, we present an empirical study based on data that we collected over five semesters while addressing plagiarism within our large online computer science graduate program. We compare plagiarism rates between two courses: one integrating new assignments and the other continuing to reuse older assignments.
In this study, we explore the benefits derived from introducing new assignments to counter plagiarism, and how long these benefits last. We then explore the trends that publicly shared solutions have on plagiarism rates, and what those trends tell us about the value of implementing new assignments. Lastly, we explore the effects that the process of detection and intervention have on the frequency of misconduct.
We observed that the benefits gained by introducing new assignments faded quickly. Additionally, we observed that proactively seeking the removal of publicly shared solutions may be ineffective unless all solutions are removed. Lastly, we observed that early detection and notification to students results in reduced misconduct over time.
Our observations underscore the notion that a single solution posted publicly can swiftly erode the advantages gained from creating new assignments to help reduce plagiarism. This raises questions about whether the advantages of introducing new assignments outweigh benefits gained through reusing and refining assignments over time. More mature and well-developed assignments tend to lend themselves to robust, experience-backed rubrics and dynamic autograders which deliver a pedagogical benefit that may outweigh the integrity benefits of frequently developing new assessments.”
KEYWORDS: plagiarism, academic misconduct, programming assignments
Ten Years, Ten Trends: The First Decade of an Affordable At-Scale Degree
David Joyner and Alex Duncan
Ten years ago in 2014 saw the launch of the first in what would become a trend to launch online, at-scale degree programs (mostly at the graduate level) by leveraging MOOC pedagogies and platforms. Using publicly-available data, this paper describes ten trends that characterize the first decade of one such program in computer science in order to assess the audience and performance of students who elect to enroll in such programs. Past research has found that students in these programs tend to enroll in large part because they do not have other options for rigorous, respected credentials that fit into their professional and personal lives; this study unpacks many of these trends. Specifically, this study finds that the fraction of women enrolling in the program has steadily increased over time, that the gender discrepancy can be partially explained by underlying differences in women in CS across different nationalities, that applicants to the program tend to be evenly split between technical and non-technical backgrounds, and that the geographic distribution of students over time has shifted toward more international audiences even while the state in which the program originates comprises the largest fraction per capita of enrollees. The paper concludes by discussing how these trends might generalize to feedback to other similar programs and other at-scale initiatives.
KEYWORDS: affordable degrees at scale, public data, gender in CS
Work-in-Progress
Answer Watermarking: Using Answer Generation Assistance Tools to Find Evidence of Cheating
Christopher Cui, Jui-Tse Hung, Pranav Sharma, Saurabh Chatterjee and Thad Starner
Cheating detection in large classes with online, take-home exams is an extremely difficult problem. While some cheating can be identified through statistical analysis of all student responses, this analysis can easily be fooled by “smart cheaters” actively attempting to hide evidence of their unauthorized collaboration. We demonstrate the effectiveness of watermarks combined with creative question design to provide evidence of cheating. We provide results from an initial deployment of our answer watermarking method and do a case study into how “smart cheaters” attempt to cover their tracks, demonstrating the need for more advanced methods of catching cheating in online, take-home exams.
KEYWORDS: cheating detection, take-home exams, online learning, academic integrity
ChatGPT’s Performance on Problem Sets in an At-Scale Introductory Computer Science Course
Diana Popescu and David Joyner
This work in progress paper examines the impact of LLMs such as ChatGPT in a college-level introductory computing course offered simultaneously as a massive open online course (MOOC) on the edX platform, focusing on its strengths and limitations in solving coding assignments. The study reveals ChatGPT’s proficiency in some areas while highlighting challenges in pseudo-code interpretation, handling multiple correct answers, and addressing complex problem statements. In order to discourage over-reliance on AI assistance from students while preserving scalability, the paper proposes strategies to enhance the difficulty of coding assignments by adding more creative elements in their structure. This research provides insights into the dynamics of AI in education and emphasizes the need for a balanced approach between technological assistance and genuine student participation.
KEYWORDS: e-learning, artificial Intelligence, applied Computing
Cheating Detection in Online Take-Home Exams
Christopher Cui, Jui-Tse Hung, Vaibhav Malhotra, Hardik Goel, Raghav Apoorv and Thad Starner
Cheating detection in large classes with online, take-home exams is an extremely difficult problem. As class size increases, the process of detection and evidence building becomes a significant investment of time. To identify cheating without invasive real-time monitoring, [Anonymized for review] uses answer rarity and submission timestamps. Creative question design allows detection of cheating even when answers are correct. Automatic report generation saves instructors time when compiling evidence for cases. [Anonymized for review] streamlines the identification and reporting of cheating students in online take-home exams, analyzing over 2 million exam submission pairs across three semesters.
KEYWORDS: cheating detection, take-home exams, online learning, academic integrity
Collaborate and Listen: International Research Collaboration at Learning @ Scale
Alex Duncan, Travis Tang, Yinghong Huang, Jeanette Luu, Nirali Thakkar and David Joyner
International research collaboration (IRC) leads to stronger, more visible research, and it allows researchers to account for global perspectives in their work. This is particularly important in Learning @ Scale research, as “scale” often implies learning that spans countries and even continents. This paper examines IRC at Learning @ Scale by analyzing the authorship of all Learning @ Scale papers over the 10 years of the conference’s history. We find that IRC at the conference is low, and possibly trending downwards. Additionally, authors from South American, African, and Asian countries tend more towards IRC, and those countries that publish most frequently at Learning @ Scale tend to be less internationally collaborative. This paper serves as a call to action for researchers and the conference to increase IRC efforts, and we provide recommendations for accomplishing this goal.
KEYWORDS: international research collaboration, IRC, review
Do Virtual Teaching Assistants Enhance Teaching Presence? (Work in progress)
Robert Lindgren, Sandeep Kakar, Pratyusha Maiti, Karan Taneja and Ashok Goel
Online learning at scale has become dramatically more popular over the last decade. While these programs provide affordable and accessible education, low retention and engagement are persistent problems. Virtual Teaching Assistants (VTAs) offer a solution: VTAs can answer questions about course logistics and content, amplifying interaction between professors and students, increasing teaching presence, and thereby improving retention and engagement. Using the Community of Inquiry framework, this paper presents what we believe is the first experimental study of the effect a VTA has on student perceptions of teaching presence, social presence, and cognitive presence. Students in a large, online, graduate computer science course were randomly assigned to sections with and without access to the VTA. The Community of Inquiry survey was then administered at the end of the semester to measure the three presences. We find that the VTA has a small, positive, statistically significant effect on the Design & Organization dimension of teaching presence as well as social presence.
KEYWORDS: Virtual Teaching Assistant, VTA, online education, teaching presence, social presence, Community of Inquiry
Forums, Feedback, and Two Kinds of AI: A Selective History of Learning @ Scale
Alex Duncan, Travis Tang, Yinghong Huang, Jeanette Luu, Nirali Thakkar and David Joyner
Since the beginning of the ACM Conference on Learning @ Scale in 2014, research has focused on a wide variety of topics. In this paper, we look at trends in four specific topics over time to identify how focus on these research directions has changed over the first decade of the conference: discussion forums, AI and machine learning, accessibility and inclusivity, and peer review. These four topics have been foundational to the growth of Learning @ Scale. We find that broadly speaking, interest in discussion forums has remained relatively steady, while interest in artificial intelligence and accessibility & inclusivity has risen. Interest in peer review, by contrast, has waned considerably. These findings are based on a an analysis of 562 total papers spanning 2014 through 2023, including full papers, work-in progress papers, and short papers.
KEYWORDS: peer review, artificial intelligence in education, machine learning in education, discussion forums, accessibility and inclusivity
HTN-Based Tutors: A New Intelligent Tutoring Framework Based on Hierarchical Task Networks
Momin Siddiqui, Adit Gupta, Jennifer Reddig and Christopher Maclellan
Intelligent tutors have shown success in delivering a personalized and adaptive learning experience. However, there exist challenges regarding the granularity of knowledge in existing frameworks and the resulting instructions they can provide. To address these issues, we propose HTN-based tutors, a new intelligent tutoring framework that represents expert models using Hierarchical Task Networks (HTNs). Like other tutoring frameworks, it allows flexible encoding of different problem-solving strategies while providing the additional benefit of a hierarchical knowledge organization. We leverage the latter to create tutors that can adapt the granularity of their scaffolding. This organization also aligns well with the compositional nature of skills.
KEYWORDS: human-centered computing, intelligent tutoring systems, artificial intelligence, Hierarchical Task Network, scaffolding
Intelligent Tutors for Adult Learning at Scale: A Narrative Review
Utkarsh Nattamai Subramanian Rajkumar, Sibley Lyndgaard and Ruth Kanfer
Intelligent tutors and tutoring systems (ITS) are increasingly common in educational contexts. These tools have considerable potential for scaling highly effective 1:1 learner-instructor interactions. To date, most studies investigating ITS implementation have engaged non-adult (i.e., child, adolescent, or traditional college student) populations, however, their potential for scaling adult learning is increasingly recognized. We performed a selected, narrative review of adult ITS, and asked two guiding questions: (1) What are the primary domains in which ITS has been deployed to promote lifespan adult learning? (2) How specifically are ITS deployed within each domain? Fifteen papers were selected, and three themes emerged: (1) Adult literacy, (2) adult post-secondary/professional education, and (3) lifelong learning and career-related development. Exemplar papers from each theme were selected and presented/discussed in more detail. The results of the review are discussed in terms of the current state of the literature on ITS for adult learning, and how such tools can improve access to personalized learning support at scale. Limitations and future directions are discussed.
KEYWORDS: intelligent tutors, adult learning, lifelong learning
Leveraging Past Assignments to Determine If Students Are Using ChatGPT for Their Essays
Yuhui Zhao, Chunhao Zou, Rohit Sridhar, Christopher Cui and Thad Starner
The proliferation of powerful large language models with human-like abilities, like ChatGPT, pose serious challenges for educators to enforce academic integrity policies. To address this problem, we propose a novel approach that uses past students’ essay submissions dated before the popularization of ChatGPT, and ChatGPT generated essay responses as ground truth to train classifiers to detect ChatGPT usage for current student submissions. Our case study found that student written answers and ChatGPT generated answers are very different. Testing on the ground truth data shows very simple machine learning methods, including multinomial naive Bayes, linear discriminant analysis, and logistic regression, can achieve close to perfect accuracies in detecting ChatGPT generated responses. Using this approach, we suspect around 7% of current student submissions we investigated are ChatGPT generated.
KEYWORDS: plagiarism detection, academic integrity, large language model detection
Open, Collaborative, and AI-Augmented Peer Assessment: Student Participation, Performance, and Perceptions
Chaohua Ou, Ploy Thajchayapong and David Joyner
Research has consistently highlighted the efficacy of peer assessment on student achievement and attitudes across different subject domains in a wide variety of contexts. However, many of related studies were focused on anonymous peer assessment conducted in small classes as a one-off and noniterative experiment. This large-scale longitudinal study explores the effects of open, collaborative, and AI-augmented peer assessment on the participation, learning performance, and perceptions of 1,636 graduate students in a large online class across 12 semesters from 2018–2022. The research is focused on how student demographics, including age and gender, influence their peer assessment and how this peer assessment affects students’ cognitive and social-affective outcomes. Key findings reveal that both factors significantly affect the level of engagement in providing feedback and the perception of its effectiveness. It also provides new insights into the positive relationship between giving feedback and improved learning performance. These findings have important implications for curriculum design and future research on peer assessment. This paper shares the implementation of peer assessment and detailed findings of the study. The implications of the study for future research and practice are also discussed.
KEYWORDS: peer assessment at scale, peer feedback, collaborative learning, artificial intelligence
Scalable Oral Assessment Powered By AI
Jui-Tse Hung, Christopher Cui, Diana Popescu, Saurabh Chatterjee and Thad Starner
Interactive teaching methods often lead to higher levels of student engagement with course material. Yet, as class sizes increase, the demand on teaching staff becomes unsustainable. Our solution, [ANONYMIZED FOR REVIEW], employs Large Language Models to provide scalable, interactive oral assessments by functioning as a virtual instructor. This paper discusses the outcomes and user feedback from the preliminary implementation of our system in a large classroom environment with 600 students.
KEYWORDS: AI, education, oral assessment, socratic questioning, Large Language Models
Towards Educator-Driven Tutor Authoring: Generative AI Approaches for Creating Intelligent Tutor Interfaces
Tommaso Calò and Christopher MacLellan
Intelligent Tutoring Systems (ITSs) have shown great potential in delivering personalized and adaptive education, but their widespread adoption has been hindered by the need for specialized programming and design skills. Existing approaches overcome the programming limitations with no-code authoring through drag and drop, however they assume that educators possess the necessary skills to design effective and engaging tutor interfaces. To address this assumption we introduce generative AI capabilities to assist educators in creating tutor interfaces that meet their needs while adhering to design principles. Our approach leverages Large Language Models (LLMs) and prompt engineering to generate tutor layout and contents based on high-level requirements provided by educators as inputs. However, to allow them to actively participate in the design process, rather than relying entirely on AI-generated solutions, we allow generation both at the entire interface level and at the individual component level. The former provides educators with a complete interface that can be refined using direct manipulation, while the latter offers the ability to create specific elements to be added to the tutor interface. A small-scale comparison shows the potential of our approach to enhance the efficiency of tutor interface design. Moving forward, we raise critical questions for assisting educators with generative AI capabilities to create personalized, effective, and engaging tutors, ultimately enhancing their adoption.
KEYWORDS: human-centered computing, intelligent tutoring systems, UI/UX, Generative User Interface
Transforming CS Education with DevOps: Streamlined Assignment Validation and Delivery @ Scale
Gururaj Deshpande, Shravan Cheekati, Shail Patel, Pranav Raj, Madhuri Singh, Mark Pindur, Nouf Al Soghyar, Bryan Zhao, Parisa Babolhavaeji, Mohammad Taher, Krish Nathan, Will Spaeth and Max M Roozbahani
The surge in interest and demand for AI skills has significantly increased student enrollment in AI and Machine Learning (ML) classes. In a large ML course at Georgia Institute of Technology, multi-week assignments encourage students to critically think about various ML algorithms theoretically and in an applied setting. Given the complexity of these large assignments, there exists the potential for bugs to remain undetected even after the verification process. These bugs lead to a significant increase in student questions and concerns, necessitate re-releasing assignment, and degrade the homework experience. To reduce or even prevent bugs in assignments, we adopt the DevOps methodology and implement a novel CI/CD pipeline along with Gitflow to automate the validation process of an assignment, from creation to release. An analysis of our classroom forum across semesters demonstrates that integrating a CI/CD pipeline with Gitflow effectively reduces the number of bug-related posts, allowing the instructional team to refocus on enhancing the student learning experience.
KEYWORDS: devops, large classrooms, automation, cicd, gitflow
Who, What, and Where: Plotting Ten Years of Learning @ Scale Research
Alex Duncan, Travis Tang, Yinghong Huang, Jeanette Luu, Nirali Thakkar and David Joyner
This paper examines trends across the first ten years of the ACM Learning @ Scale conference across three dimensions: the context of the research, the subject matter addressed in the research, and the home of the authors of the research. All 562 papers published in the conference’s history were each coded for context, subject, and researcher affiliation. Analysis of the results of this coding reveal a significant drop in MOOC research, a rise in research from outside the United States, and a relatively stable focus on computer science and STEM curricula.
KEYWORDS: MOOCs, affordable at-scale degrees, informal learning environments, CS education
Workshop
Scaling Classrooms: A forum for practitioners seeking, developing and adapting their own tools
Christopher Cui, Gururaj Deshpande and Thad Starner
In this half-day workshop, we seek to establish a continuing dialogue across classrooms about the challenges faced from a rapidly expanding student body, such as is happening in Computer Science departments. We plan to catalog a list of these challenges, the tools developed internally to overcome these challenges, and the barriers to adoption of these tools for the learning community at large. We invite practitioners who are in the process or creating or adapting educational technologies for scale to share their challenges and experiences.
KEYWORDS: scaling, educational technologies, large classrooms
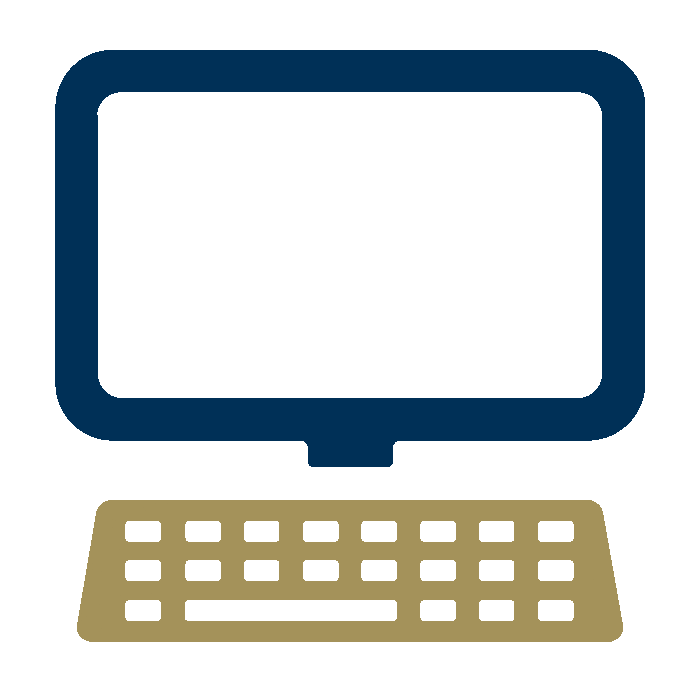
See you in Atlanta!
Development: College of Computing
Project Lead/Data Graphics: Joshua Preston
Select Photos: Kevin Beasley
Data Management: Joni Isbell