NeurIPS 2024
Annual Conference on Neural Information Processing Systems | Dec. 10–15, 2024 | Vancouver

The annual Conference on Neural Information Processing Systems (NeurIPS), Dec. 10-15, 2024, in Vancouver, is one of the largest and most influential conferences for research in artificial intelligence (AI), machine learning, and related fields, and showcases papers on topics such as deep learning, reinforcement learning, generative AI, and neuroscience-inspired computing.
Georgia Tech is represented by 84 teams—among the approximately 4,000 total in the main program—that have contributed new discoveries to AI technologies and applications.
Explore the interdisciplinary teams and papers from Georgia Tech and its partners. The institute’s College of Computing and College of Engineering faculty represent the majority of the 46 Tech faculty experts in the main program. Several share their thoughts here—in the section “Advancing AI Frontiers.” They share the direction their work is taking as AI opens a future full of capabilities and concerns. Explore now .
Artificial intelligence holds the power to amplify human creativity and aspirations, turning ideas into realities we once thought impossible. Meet the Georgia Tech experts charting a path forward.
#NeurIPS2024
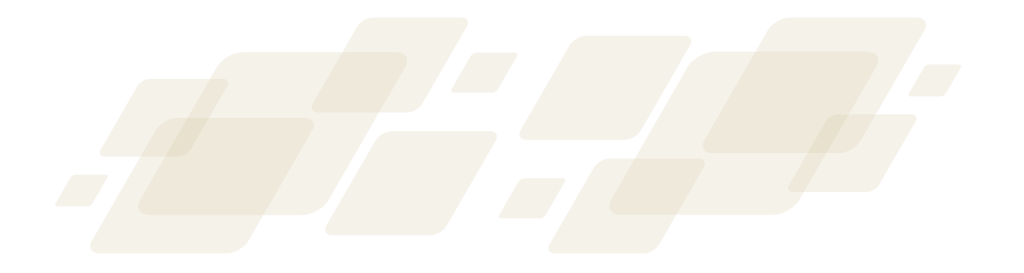
Georgia Tech at NeurIPS 2024
By the Numbers
Partner Organizations
Agency for Science, Technology and Research (A*STAR) • Alibaba Group • Allen Institute for AI • Amazon AGI Foundations • Apple • AX AI • Brown University • ByteDance Inc. • Caltech • Carnegie Mellon University • Chinese Academy of Sciences • Colfax International • Columbia University • Cornell University • Dartmouth College • Duke University • Eberhard-Karls-Universität Tübingen • Emory University • Georgia Tech • Google • Hamline University • Harvard University • Helixon • Hillbot Inc. • HKUST • IBM • Institut de Physique du Globe • Institute for Infocomm Research • Intel • International Brain Laboratory • Johns Hopkins University • Massachusetts Institute of Technology • Max-Planck-Institute for Intelligent Systems • McGill University / Mila • Meituan • Meta • Microsoft Research • Nanjing University • Nanyang Technological University • National University of Singapore • National Yang Ming Chiao Tung University • New York University • NVIDIA • Peking University • Pohang University of Science and Technology • Princeton University • PsiQuantum Corp • Rochester Institute of Technology • Rutgers University • Samsung Research America • Shanghai Jiao Tong University • Shenzhen Technology University • Snap, Inc. • Squirrel AI • Stanford University • Terminus Group • Texas A&M University • Together AI • Toyota Technological Institute at Chicago • TReNDS center • Tsinghua University • University College London • University of Alberta • University of Bonn • University of British Columbia • University of California, Berkeley • University of California, Davis • University of California, Los Angeles • University of California, San Diego • University of California, Santa Barbara • University of Central Florida • University of Chicago • University of Edinburgh • University of Illinois Urbana-Champaign • University of Massachusetts, Amherst • University of Minnesota – Twin Cities • University of North Carolina at Chapel Hill • University of Oxford • University of Pennsylvania • University of Rochester • University of Science and Technology of China • University of Southern California • University of Texas, Austin • University of Tokyo • University of Toronto • University of Trento • University of Tuebingen • University of Washington • Virginia Polytechnic Institute and State University • Weizmann Institute of Science • Xiamen University • Yale University • Zhejiang University •

Advancing AI Frontiers
What does artificial intelligence (AI) look like for our researchers and inventors? Explore this page to learn from Tech faculty about the growing impact of some featured areas.

Faculty in technical program 

AI FRONTIERS
Trustworthy Machine Learning
Trustworthy machine learning aims to create AI systems people can rely on, delivering fair, transparent, secure and accurate results aligned with human values. Our NeurIPS ’24 research, “Navigating the Safety Landscape: Measuring Risks in Finetuning Large Language Models” is in collaboration with IBM and introduces the “safety basin,” a universal phenomenon in large language models (LLMs). We found that fine-tuning, commonly used to adapt LLMs to new data, can pull models out of this safe zone, reducing their reliability. This discovery highlights unexpected safety risks in a common practice and points to new strategies for improving LLM safety.
Duen Horng “Polo” Chau
Professor • Computational Science and Engineering
AI FRONTIERS
Trustworthy Machine Learning

Duen Horng “Polo” Chau
Professor • Computational Science and Engineering
Trustworthy machine learning aims to create AI systems people can rely on, delivering fair, transparent, secure and accurate results aligned with human values. Our NeurIPS ’24 research, “Navigating the Safety Landscape: Measuring Risks in Finetuning Large Language Models” is in collaboration with IBM and introduces the “safety basin,” a universal phenomenon in large language models (LLMs). We found that fine-tuning, commonly used to adapt LLMs to new data, can pull models out of this safe zone, reducing their reliability. This discovery highlights unexpected safety risks in a common practice and points to new strategies for improving LLM safety.
The Big Picture 

AI FRONTIERS
Datasets and Benchmarks
General datasets like SQuAD and ImageNet have driven AI progress, but domain-specific benchmarks reveal crucial gaps in performance. As AI models have started surpassing human evaluations, building high-quality datasets becomes a greater challenge. It’s not just about testing models—it’s about redefining what “good” looks like in specialized domains like finance, where managing risk and capturing critical insights matter more than just precision and nuance.
Sudheer Chava
Alton M. Costley Chair Professor • Finance
AI FRONTIERS
Datasets and Benchmarks

Sudheer Chava
Alton M. Costley Chair Professor • Finance
General datasets like SQuAD and ImageNet have driven AI progress, but domain-specific benchmarks reveal crucial gaps in performance. As AI models have started surpassing human evaluations, building high-quality datasets becomes a greater challenge. It’s not just about testing models—it’s about redefining what “good” looks like in specialized domains like finance, where managing risk and capturing critical insights matter more than just precision and nuance.
Georgia Tech’s partners in the main program represent more than a dozen countries, with 375 total external partners. Approximately 75% of those partners are from domestic organizations.
Tech’s experts (~162) come from across the institute, and faculty primarily represent the College of Computing and College of Engineering. There are 46 total faculty in the main program.



AI FRONTIERS
Neuroscience and Cognitive Science
Neuroscience is now tapping into the potential of scaling up computation to discover breakthroughs, but it’s not easy—every brain recording is unique, and the brain’s complexity makes simple solutions impossible. At NeurIPS, we introduce a new approach that uses self-supervised learning to handle large-scale multi-region brain datasets. By training on data from multiple regions, tasks, and animals, our method moves us closer to creating universal models that can help shed light onto how the brain works.
Eva Dyer
Assoc. Professor • Biomedical Engineering
AI FRONTIERS
Neuroscience and Cognitive Science

Eva Dyer
Assoc. Professor • Biomedical Engineering
Neuroscience is now tapping into the potential of scaling up computation to discover breakthroughs, but it’s not easy—every brain recording is unique, and the brain’s complexity makes simple solutions impossible. At NeurIPS, we introduce a new approach that uses self-supervised learning to handle large-scale multi-region brain datasets. By training on data from multiple regions, tasks, and animals, our method moves us closer to creating universal models that can help shed light onto how the brain works.

FEATURED
Multipurpose Model Enhances Forecasting Across Epidemics, Energy, and Economics
By Bryant Wine
A new machine learning (ML) model from Georgia Tech could protect communities from diseases, better manage electricity consumption in cities, and promote business growth, all at the same time.
Researchers from the School of Computational Science and Engineering (CSE) created the Large Pre-Trained Time-Series Model (LPTM) framework. LPTM is a single foundational model that completes forecasting tasks across a broad range of domains.
Along with performing as well or better than models purpose-built for their applications, LPTM requires 40% less data and 50% less training time than current baselines. In some cases, LPTM can be deployed without any training data.
The key to LPTM is that it is pre-trained on datasets from different industries like healthcare, transportation, and energy. The Georgia Tech group created an adaptive segmentation module to make effective use of these vastly different datasets.

“The foundational model paradigm started with text and image, but people haven’t explored time-series tasks yet because those were considered too diverse across domains. Our work is a pioneer in this new area of exploration where only few attempts have been made so far.”
B. Aditya Prakash
Assoc. Professor, School of Computational Science and Engineering at Georgia Tech
FEATURED
New Dataset Takes Aim at Subjective Misinformation in Earnings Calls and Other Public Hearings
By Bryant Wine
Georgia Tech researchers have created a dataset that trains computer models to understand nuances in human speech during financial earnings calls. The dataset provides a new resource to study how public correspondence affects businesses and markets.
SubjECTive-QA is the first human-curated dataset on question-answer pairs from earnings call transcripts (ECTs). The dataset teaches models to identify subjective features in ECTs, like clarity and cautiousness.
The dataset lays the foundation for a new approach to identifying disinformation and misinformation caused by nuances in speech. While ECT responses can be technically true, unclear or irrelevant information can misinform stakeholders and affect their decision-making.

“SubjECTive-QA has the potential to revolutionize nowcasting predictions with enhanced clarity and relevance. Its nuanced analysis of qualities in executive responses, like optimism and cautiousness, deepens our understanding of economic forecasts and financial transparency.”
Agam Alkeshkumar Shah
Machine Learning Ph.D. student, School of Computational Science and Engineering at Georgia Tech


AI FRONTIERS
Multimodal Models for Action
Multimodal models have enabled chat assistants that can answer questions about an image or summarize a video. But can they act in the world? Our work has developed methods that adapt multimodal models that are trained from web data, soaking up common-sense knowledge, and directly output actions that a robot can carry out. Interestingly, we show that the language component is critical to allowing the robots to generalize better across many different tasks. As multimodal models continue to improve, they have a strong potential to further make our robots smarter.
Zsolt Kira
Assoc. Professor • Interactive Computing
AI FRONTIERS
Multimodal Models for Action

Zsolt Kira
Assoc. Professor • Interactive Computing
Multimodal models have enabled chat assistants that can answer questions about an image or summarize a video. But can they act in the world? Our work has developed methods that adapt multimodal models that are trained from web data, soaking up common-sense knowledge, and directly output actions that a robot can carry out. Interestingly, we show that the language component is critical to allowing the robots to generalize better across many different tasks. As multimodal models continue to improve, they have a strong potential to further make our robots smarter.
Top NeurIPS Papers 
Georgia Tech experts (below in italicized bold) contributed to the following top papers. Of the 4000+ papers accepted to NeurIPS 2024, 10 percent are recognized as the top contributions in the program. “Oral” papers are the top one percent (61 papers), with “spotlight” papers representing the remaining top 10 percent (327 papers).
MeshFormer : High-Quality Mesh Generation with 3D-Guided Reconstruction Model
Minghua Liu, Chong Zeng, Xinyue Wei, Ruoxi Shi, Linghao Chen, Chao Xu, Mengqi Zhang, Zhaoning Wang, Xiaoshuai Zhang, Isabella Liu, Hongzhi Wu, Hao Su
Double-Ended Synthesis Planning with Goal-Constrained Bidirectional Search
Kevin Yu, Jihye Roh, Ziang Li, Wenhao Gao, Runzhong Wang, Connor Coley
FlashAttention-3: Fast and Accurate Attention with Asynchrony and Low-precision
Jay Shah, Ganesh Bikshandi, Ying Zhang, Vijay Thakkar, Pradeep Ramani, Tri Dao
In-and-Out: Algorithmic Diffusion for Sampling Convex Bodies
Yunbum Kook, Santosh Vempala, Matthew Zhang
Langevin Unlearning: A New Perspective of Noisy Gradient Descent for Machine Unlearning
Eli Chien, Haoyu Wang, Ziang Chen, Pan Li
Latent Diffusion for Neural Spiking Data
Jaivardhan Kapoor, Auguste Schulz, Julius Vetter, Felix Pei, Richard Gao, Jakob H Macke
Pre-trained Text-to-Image Diffusion Models Are Versatile Representation Learners for Control
Gunshi Gupta, Karmesh Yadav, Yarin Gal, Dhruv Batra, Zsolt Kira, Cong Lu, Tim G. J. Rudner
Sample Complexity of Posted Pricing for a Single Item
Billy Jin, Thomas Kesselheim, Will Ma, Sahil Singla
Selective Generation for Controllable Language Models
Minjae Lee, Kyungmin Kim, Taesoo Kim, Sangdon Park

AI FRONTIERS
Computer Vision
3D rendering and large language models (LLMs) are two foundational workloads driving innovation in robotics, autonomous driving, and intelligent agents across numerous real-world applications. These fields demand solutions that are not only efficient and scalable but also easy to develop and deploy across diverse platforms. Our NeurIPS 2024 papers rise to this challenge, delivering state-of-the-art techniques to advance efficient 3D intelligence, computer vision, and LLM-powered systems. By pushing the boundaries of efficiency and ease of development, our research aspires to bring transformative AI closer to daily life and unlock new possibilities for ubiquitous intelligence.
Yingyan “Celine” Lin
Assoc. Professor • Computer Science
AI FRONTIERS
Computer Vision

Yingyan “Celine” Lin
Assoc. Professor • Computer Science
3D rendering and large language models (LLMs) are two foundational workloads driving innovation in robotics, autonomous driving, and intelligent agents across numerous real-world applications. These fields demand solutions that are not only efficient and scalable but also easy to develop and deploy across diverse platforms. Our NeurIPS 2024 papers rise to this challenge, delivering state-of-the-art techniques to advance efficient 3D intelligence, computer vision, and LLM-powered systems. By pushing the boundaries of efficiency and ease of development, our research aspires to bring transformative AI closer to daily life and unlock new possibilities for ubiquitous intelligence.
RESEARCH 
SELECT TOPIC
- Algorithms
- Applications
- Attention Mechanisms
- Bilevel optimization
- Computational Biology
- Computer Vision
- Data-centric AI methods and tools
- Datasets and Benchmarks
- Deep RL
- Diffusion models
- Discrete and Combinatorial Optimization
- Fairness
- Game Theory
- Graph Neural Networks
- Human-computer interaction
- Image Generation
- Kernel methods
- Language
- Learning for Optimization
- Learning Theory
- Monte Carlo and Sampling Methods
- Multimodal Models
- Neuroscience, Cognitive Science
- New Approaches
- Non-Convex
- Online Learning
- Privacy
- Reasoning
- Representation Learning
- Robotics
- Self-Supervised Learning
- Speech
- Text Understanding
- Theory
- Time Series
- Trustworthy Machine Learning
- Other
DEPrune: Depth-wise Separable Convolution Pruning for Maximizing GPU Parallelism
Cheonjun Park, Mincheol Park, Hyunchan Moon, Myung Kuk Yoon, Seokjin Go, Suhyun Kim, Won Woo Ro
Depth-wise Separable Convolution (DSConv) has a powerful representation even with fewer parameters and computation, leading to its adoption by almost all of the state-of-the-art CNN models. DSConv models are already compact making it hard to apply pruning, and there are few previous pruning techniques that target depth-wise convolution (DW-conv).In this paper, we present Depth-wise Separable Convolution Pruning (DEPrune), a novel pruning method applied to both point-wise and depth-wise convolutions. DEPrune is optimized by analyzing the computation of DSConv on GPUs.DEPrune employs a fine-grained pruning approach, yet it achieves the structured sparsity typically absent in fine-grained pruning, enabling practical hardware acceleration. Moreover, this method maintains a high pruning ratio without causing any accuracy drop.We additionally represent techniques that further enhance DEPrune performance: 1) balanced workload tuning (BWT), and 2) hardware-aware sparsity recalibration (HSR).Experiment results show that DEPrune achieves up to $3.74\times$ practical speedup in DSConv inference on GPUs while maintaining the accuracy of EfficientNet-B0 on ImageNet.
ShiftAddLLM: Accelerating Pretrained LLMs via Post-Training Multiplication-Less Reparameterization
Haoran You, Yipin Guo, Yichao Fu, Wei Zhou, Huihong Shi, Xiaofan Zhang, Souvik Kundu, Amir Yazdanbakhsh, Yingyan (Celine) Lin
Large language models (LLMs) have shown impressive performance on language tasks but face challenges when deployed on resource-constrained devices due to their extensive parameters and reliance on dense multiplications, resulting in high memory demands and latency bottlenecks. Shift-and-add reparameterization offers a promising solution by replacing costly multiplications with hardware-friendly primitives in both the attention and multi-layer perceptron (MLP) layers of an LLM. However, current reparameterization techniques require training from scratch or full parameter fine-tuning to restore accuracy, which is resource-intensive for LLMs. To address this, we propose accelerating pretrained LLMs through post-training shift-and-add reparameterization, creating efficient multiplication-free models, dubbed ShiftAddLLM. Specifically, we quantize each weight matrix into binary matrices paired with group-wise scaling factors. The associated multiplications are reparameterized into (1) shifts between activations and scaling factors and (2) queries and adds according to the binary matrices. To reduce accuracy loss, we present a multi-objective optimization method to minimize both weight and output activation reparameterization errors. Additionally, based on varying sensitivity across layers to reparameterization, we develop an automated bit allocation strategy to further reduce memory usage and latency. Experiments on five LLM families and eight tasks consistently validate the effectiveness of ShiftAddLLM, achieving average perplexity reductions of 5.6 and 22.7 points at comparable or lower latency compared to the most competitive quantized LLMs at 3- and 2-bit precision, respectively, and more than 80% memory and energy reductions over the original LLMs. Codes and models are available at https://github.com/GATECH-EIC/ShiftAddLLM.
Lorenzo Orecchia, Jiawei Hu, Xue He, Wang Mark, Xulei Yang, Min Wu, Xue Geng
Current methods for training Binarized Neural Networks (BNNs) heavily rely on the heuristic straight-through estimator (STE), which crucially enables the application of SGD-based optimizers to the combinatorial training problem. Although the STE heuristics and their variants have led to significant improvements in BNN performance, their theoretical underpinnings remain unclear and relatively understudied. In this paper, we propose a theoretically motivated optimization framework for BNN training based on Gaussian variational inference. In its simplest form, our approach yields a non-convex linear programming formulation whose variables and associated gradients motivate the use of latent weights and STE gradients. More importantly, our framework allows us to formulate semidefinite programming (SDP) relaxations to the BNN training task. Such formulations are able to explicitly models pairwise correlations between weights during training, leading to a more accurate optimization characterization of the training problem. As the size of such formulations grows quadratically in the number of weights, quickly becoming intractable for large networks, we apply the Burer-Monteiro approach and only optimize over linear-size low-rank SDP solutions. Our empirical evaluation on CIFAR-10, CIFAR-100, Tiny-ImageNet and ImageNet datasets shows our method consistently outperforming all state-of-the-art algorithms for training BNNs.
SPOTLIGHT Double-Ended Synthesis Planning with Goal-Constrained Bidirectional Search
Kevin Yu, Jihye Roh, Ziang Li, Wenhao Gao, Runzhong Wang, Connor Coley
Computer-aided synthesis planning (CASP) algorithms have demonstrated expert-level abilities in planning retrosynthetic routes to molecules of low to moderate complexity. However, current search methods assume the sufficiency of reaching arbitrary building blocks, failing to address the common real-world constraint where using specific molecules is desired. To this end, we present a formulation of synthesis planning with starting material constraints. Under this formulation, we propose Double-Ended Synthesis Planning ($\texttt{DESP}$), a novel CASP algorithm under a _bidirectional graph search_ scheme that interleaves expansions from the target and from the goal starting materials to ensure constraint satisfiability. The search algorithm is guided by a goal-conditioned cost network learned offline from a partially observed hypergraph of valid chemical reactions. We demonstrate the utility of $\texttt{DESP}$ in improving solve rates and reducing the number of search expansions by biasing synthesis planning towards expert goals on multiple new benchmarks. $\texttt{DESP}$ can make use of existing one-step retrosynthesis models, and we anticipate its performance to scale as these one-step model capabilities improve.
Faster Neighborhood Attention: Reducing the O(n^2) Cost of Self Attention at the Threadblock Level
Ali Hassani, Wen-Mei Hwu, Humphrey Shi
Neighborhood attention reduces the cost of self attention by restricting each token’s attention span to its nearest neighbors. This restriction, parameterized by a window size and dilation factor, draws a spectrum of possible attention patterns between linear projection and self attention. Neighborhood attention, and more generally sliding window attention patterns, have long been bounded by infrastructure, particularly in higher-rank spaces (2-D and 3-D), calling for the development of custom kernels, which have been limited in either functionality, or performance, if not both. In this work, we aim to massively improve upon existing infrastructure by providing two new methods for implementing neighborhood attention. We first show that neighborhood attention can be represented as a batched GEMM problem, similar to standard attention, and implement it for 1-D and 2-D neighborhood attention. These kernels on average provide 895% and 272% improvement in full precision runtime compared to existing naive CUDA kernels for 1-D and 2-D neighborhood attention respectively. We find that aside from being heavily bound by memory bandwidth, certain inherent inefficiencies exist in all unfused implementations of neighborhood attention, which in most cases undo their theoretical efficiency gain. Motivated by the progress made into fused dot-product attention kernels, we developed fused neighborhood attention; an adaptation of fused dot-product attention kernels that allow fine-grained control over attention across different spatial axes. Known for reducing the quadratic time complexity of self attention to a linear complexity, neighborhood attention can now enjoy a reduced and constant memory footprint, and record-breaking half precision runtime. We observe that our fused implementation successfully circumvents some of the unavoidable inefficiencies in unfused implementations. While our unfused GEMM-based kernels only improve half precision performance compared to naive kernels by an average of 548% and 193% in 1-D and 2-D problems respectively, our fused kernels improve naive kernels by an average of 1759% and 958% in 1-D and 2-D problems respectively. These improvements translate into up to 104% improvement in inference and 39% improvement in training existing models based on neighborhood attention, and additionally extend its applicability to image and video perception, as well as other modalities. Our work is open-sourced at https://github.com/SHI-Labs/NATTEN/.
SPOTLIGHT FlashAttention-3: Fast and Accurate Attention with Asynchrony and Low-precision
Jay Shah, Ganesh Bikshandi, Ying Zhang, Vijay Thakkar, Pradeep Ramani, Tri Dao
Attention, as a core layer of the ubiquitous Transformer architecture, is the bottleneck for large language models and long-context applications. elaborated an approach to speed up attention on GPUs through minimizing memory reads/writes. However, it has yet to take advantage of new capabilities present in recent hardware, with FlashAttention-2 achieving only 35% utilization on the H100 GPU.We develop three main techniques to speed up attention on Hopper GPUs: exploiting asynchrony of the Tensor Cores and TMA to (1) overlap overall computation and data movement via warp-specialization and (2) interleave block-wise matmul and softmax operations, and (3) block quantization and incoherent processing that leverages hardware support for FP8 low-precision. We demonstrate that our method, FlashAttention-3, achieves speedup on H100 GPUs by 1.5-2.0$\times$ with BF16 reaching up to 840 TFLOPs/s (85\% utilization), and with FP8 reaching 1.3 PFLOPs/s. We validate that FP8 FlashAttention-3 achieves 2.6$\times$ lower numerical error than a baseline FP8 attention.
First-Order Methods for Linearly Constrained Bilevel Optimization
Guy Kornowski, Swati Padmanabhan, Kai Wang, Zhe Zhang, Suvrit Sra
Algorithms for bilevel optimization often encounter Hessian computations, which are prohibitive in high dimensions. While recent works offer first-order methods for unconstrained bilevel problems, the constrained setting remains relatively underexplored. We present first-order linearly constrained optimization methods with finite-time hypergradient stationarity guarantees. For linear equality constraints, we attain $\epsilon$-stationarity in $\widetilde{O}(\epsilon^{-2})$ gradient oracle calls, which is nearly-optimal. For linear inequality constraints, we attain $(\delta,\epsilon)$-Goldstein stationarity in $\widetilde{O}(d{\delta^{-1} \epsilon^{-3}})$ gradient oracle calls, where $d$ is the upper-level dimension. Finally, we obtain for the linear inequality setting dimension-free rates of $\widetilde{O}({\delta^{-1} \epsilon^{-4}})$ oracle complexity under the additional assumption of oracle access to the optimal dual variable. Along the way, we develop new nonsmooth nonconvex optimization methods with inexact oracles. Our numerical experiments verify these guarantees.
Semi-supervised Knowledge Transfer Across Multi-omic Single-cell Data
Fan Zhang, Tianyu Liu, Zihao Chen, Xiaojiang Peng, Chong Chen, Xian-Sheng Hua, Xiao Luo, Hongyu Zhao
Knowledge transfer between multi-omic single-cell data aims to effectively transfer cell types from scRNA-seq data to unannotated scATAC-seq data. Several approaches aim to reduce the heterogeneity of multi-omic data while maintaining the discriminability of cell types with extensive annotated data. However, in reality, the cost of collecting both a large amount of labeled scRNA-seq data and scATAC-seq data is expensive. Therefore, this paper explores a practical yet underexplored problem of knowledge transfer across multi-omic single-cell data under cell type scarcity. To address this problem, we propose a semi-supervised knowledge transfer framework named Dual label scArcity elimiNation with Cross-omic multi-samplE Mixup (DANCE). To overcome the label scarcity in scRNA-seq data, we generate pseudo-labels based on optimal transport and merge them into the labeled scRNA-seq data. Moreover, we adopt a divide-and-conquer strategy which divides the scATAC-seq data into source-like and target-specific data. For source-like samples, we employ consistency regularization with random perturbations while for target-specific samples, we select a few candidate labels and progressively eliminate incorrect cell types from the label set for additional supervision. Next, we generate virtual scRNA-seq samples with multi-sample Mixup based on the class-wise similarity to reduce cell heterogeneity. Extensive experiments on many benchmark datasets suggest the superiority of our DANCE over a series of state-of-the-art methods.
3D Gaussian Rendering Can Be Sparser: Efficient Rendering via Learned Fragment Pruning
Zhifan Ye, Chenxi Wan, Chaojian Li, Jihoon Hong, Sixu Li, Leshu Li, Yongan Zhang, Yingyan (Celine) Lin
3D Gaussian splatting has recently emerged as a promising technique for novel view synthesis from sparse image sets, yet comes at the cost of requiring millions of 3D Gaussian primitives to reconstruct each 3D scene. This largely limits its application to resource-constrained devices and applications.Despite advances in Gaussian pruning techniques that aim to remove individual 3D Gaussian primitives, the significant reduction in primitives often fails to translate into commensurate increases in rendering speed, impeding efficiency and practical deployment. We identify that this discrepancy arises due to the overlooked impact of fragment count per Gaussian (i.e., the number of pixels each Gaussian is projected onto). To bridge this gap and meet the growing demands for efficient on-device 3D Gaussian rendering, we propose fragment pruning, an orthogonal enhancement to existing pruning methods that can significantly accelerate rendering by selectively pruning fragments within each Gaussian. Our pruning framework dynamically optimizes the pruning threshold for each Gaussian, markedly improving rendering speed and quality. Extensive experiments in both static and dynamic scenes validate the effectiveness of our approach. For instance, by integrating our fragment pruning technique with state-of-the-art Gaussian pruning methods, we achieve up to a 1.71$\times$ speedup on an edge GPU device, the Jetson Orin NX, and enhance rendering quality by an average of 0.16 PSNR on the Tanks\&Temples dataset. Our code is available at https://github.com/GATECH-EIC/Fragment-Pruning.
ORAL MeshFormer : High-Quality Mesh Generation with 3D-Guided Reconstruction Model
Minghua Liu, Chong Zeng, Xinyue Wei, Ruoxi Shi, Linghao Chen, Chao Xu, Mengqi Zhang, Zhaoning Wang, Xiaoshuai Zhang, Isabella Liu, Hongzhi Wu, Hao Su
Open-world 3D reconstruction models have recently garnered significant attention. However, without sufficient 3D inductive bias, existing methods typically entail expensive training costs and struggle to extract high-quality 3D meshes. In this work, we introduce MeshFormer, a sparse-view reconstruction model that explicitly leverages 3D native structure, input guidance, and training supervision. Specifically, instead of using a triplane representation, we store features in 3D sparse voxels and combine transformers with 3D convolutions to leverage an explicit 3D structure and projective bias. In addition to sparse-view RGB input, we require the network to take input and generate corresponding normal maps. The input normal maps can be predicted by 2D diffusion models, significantly aiding in the guidance and refinement of the geometry’s learning. Moreover, by combining Signed Distance Function (SDF) supervision with surface rendering, we directly learn to generate high-quality meshes without the need for complex multi-stage training processes. By incorporating these explicit 3D biases, MeshFormer can be trained efficiently and deliver high-quality textured meshes with fine-grained geometric details. It can also be integrated with 2D diffusion models to enable fast single-image-to-3D and text-to-3D tasks. **Videos are available at https://meshformer3d.github.io/**
Rad-NeRF: Ray-decoupled Training of Neural Radiance Field
Lidong Guo, Xuefei Ning, Yonggan Fu, Tianchen Zhao, Zhuoliang Kang, Jincheng Yu, Yingyan (Celine) Lin, Yu Wang
Although the neural radiance field (NeRF) exhibits high-fidelity visualization on the rendering task, it still suffers from rendering defects, especially in complex scenes. In this paper, we delve into the reason for the unsatisfactory performance and conjecture that it comes from interference in the training process. Due to occlusions in complex scenes, a 3D point may be invisible to some rays. On such a point, training with those rays that do not contain valid information about the point might interfere with the NeRF training. Based on the above intuition, we decouple the training process of NeRF in the ray dimension softly and propose a Ray-decoupled Training Framework for neural rendering (Rad-NeRF). Specifically, we construct an ensemble of sub-NeRFs and train a soft gate module to assign the gating scores to these sub-NeRFs based on specific rays. The gate module is jointly optimized with the sub-NeRF ensemble to learn the preference of sub-NeRFs for different rays automatically. Furthermore, we introduce depth-based mutual learning to enhance the rendering consistency among multiple sub-NeRFs and mitigate the depth ambiguity. Experiments on five datasets demonstrate that Rad-NeRF can enhance the rendering performance across a wide range of scene types compared with existing single-NeRF and multi-NeRF methods. With only 0.2% extra parameters, Rad-NeRF improves rendering performance by up to 1.5dB. Code is available at https://github.com/thu-nics/Rad-NeRF.
Data-centric AI methods and tools
DART-Math: Difficulty-Aware Rejection Tuning for Mathematical Problem-Solving
Yuxuan Tong, Xiwen Zhang, Rui Wang, Ruidong Wu, Junxian He
Solving mathematical problems requires advanced reasoning abilities and presents notable challenges for large language models. Previous works usually synthesize data from proprietary models to augment existing datasets, followed by instruction tuning to achieve top-tier results. However, our analysis of these datasets reveals severe biases towards easy queries, with frequent failures to generate any correct response for the most challenging queries.Hypothesizing that difficult queries are crucial to learning complex reasoning, we propose *Difficulty-Aware Rejection Tuning* (`DART`), a method that allocates difficult queries more trials during the synthesis phase, enabling more extensive training on difficult samples.Utilizing `DART`, we have created new datasets for mathematical problem-solving that focus more on difficult queries and are substantially smaller than previous ones. Remarkably, our synthesis process solely relies on a 7B-sized open-weight model, without reliance on the commonly used proprietary GPT-4.We fine-tune various base models on our datasets ranging from 7B to 70B in size, resulting in a series of strong models called `DART-Math`.In comprehensive in-domain and out-of-domain evaluation on 6 mathematical benchmarks, `DART-Math` outperforms vanilla rejection tuning significantly, being superior or comparable to previous arts, despite using much smaller datasets and no proprietary models. Furthermore, our results position our synthetic datasets as the most effective and cost-efficient publicly available resources for advancing mathematical problem-solving. Our datasets, models and code are publicly available at https://github.com/hkust-nlp/dart-math.
cPAPERS: A Dataset of Situated and Multimodal Interactive Conversations in Scientific Papers
Anirudh Sundar, Jin Xu, William Gay, Christopher Richardson, Larry Heck
An emerging area of research in situated and multimodal interactive conversations (SIMMC) includes interactions in scientific papers. Since scientific papers are primarily composed of text, equations, figures, and tables, SIMMC methods must be developed specifically for each component to support the depth of inquiry and interactions required by research scientists. This work introduces $Conversational Papers$ (cPAPERS), a dataset of conversational question-answer pairs from reviews of academic papers grounded in these paper components and their associated references from scientific documents available on arXiv. We present a data collection strategy to collect these question-answer pairs from OpenReview and associate them with contextual information from $LaTeX$ source files. Additionally, we present a series of baseline approaches utilizing Large Language Models (LLMs) in both zero-shot and fine-tuned configurations to address the cPAPERS dataset.
Semi-Truths: A Large-Scale Dataset for Testing Robustness of AI-Generated Image Detectors
Anisha Pal, Julia Kruk, Mansi Phute, Manognya Bhattaram, Diyi Yang, Duen Horng Chau, Judy Hoffman
While text-to-image diffusion models have demonstrated impactful applications in art, design, and entertainment, these technologies also facilitate the spread of misinformation. Recent efforts have developed AI-generated image detectors claiming robustness against various augmentations, but their effectiveness remains unclear. Can these systems detect varying degrees of augmentation? Do they exhibit biases towards specific scenes or data distributions? To address these questions, we introduce Semi-Truths, featuring 27,635 real images, 245,360 masks, and 850,226 AI-augmented images featuring varying degrees of targeted and localized edits, created using diverse augmentation methods, diffusion models, and data distributions. Each augmented image includes detailed metadata for standardized, targeted evaluation of detector robustness. Our findings suggest that state-of-the-art detectors are sensitive to different degrees of edits, data distributions, and editing techniques, providing deeper insights into their functionality.
SubjECTive-QA: A dataset for the subjective evaluation of answers in Earnings Call Transcripts (ECTs)
Huzaifa Pardawala, Siddhant Sukhani, Veer Kejriwal, Rohan Bhasin, Abhishek Pillai, Dhruv Adha, Tarun Mandapati, Andrew DiBiasio, Agam Shah, Sudheer Chava
Fact-checking is extensively studied in the context of misinformation and disinformation, addressing objective inaccuracies. However, a softer form of misinformation involves responses that are factually correct but lack certain features such as clarity and relevance. This challenge is prevalent in formal Question-Answer (QA) settings such as press conferences in finance, politics, sports, and other domains, where subjective answers can obscure transparency. Despite this, there is a lack of manually annotated datasets for subjective features across multiple dimensions. To address this gap, we introduce SubjECTive-QA, a manually annotated dataset created by nine annotators on Earnings Call Transcripts (ECTs) as the companies’ statements are often subjective and open to scrutiny. The dataset includes 2,747 annotated long-form QA pairs across six features: Assertive, Cautious, Optimistic, Specific, Clear, and Relevant. Benchmarking on our dataset reveals that the best-performing Pre-trained Language Model (PLM), RoBERTa-base, has similar weighted F1 scores to Llama-3-70b-Chat on features with lower subjectivity, such as Relevant and Clear, with a mean difference of 2.17% in their weighted F1 scores, but significantly better on features with higher subjectivity, such as Specific and Assertive, with a mean difference of 10.01% in their weighted F1 scores. Furthermore, testing SubjECTive-QA’s generalizability using QAs from White House Press Briefings and Gaggles yields an average weighted F1 score of 65.97% using our best models for each feature, demonstrating broader applicability beyond the financial domain. SubjECTive-QA is currently made available anonymously under the CC BY 4.0 license.
Adaptive Preference Scaling for Reinforcement Learning with Human Feedback
Ilgee Hong, Zichong Li, Alexander Bukharin, Yixiao Li, Haoming Jiang, Tianbao Yang, Tuo Zhao
Reinforcement learning from human feedback (RLHF) is a prevalent approach to align AI systems with human values by learning rewards from human preference data. Due to various reasons, however, such data typically takes the form of rankings over pairs of trajectory segments, which fails to capture the varying strengths of preferences across different pairs. In this paper, we propose a novel adaptive preference loss, underpinned by distributionally robust optimization (DRO), designed to address this uncertainty in preference strength. By incorporating an adaptive scaling parameter into the loss for each pair, our method increases the flexibility of the reward function. Specifically, it assigns small scaling parameters to pairs with ambiguous preferences, leading to more comparable rewards, and large scaling parameters to those with clear preferences for more distinct rewards. Computationally, our proposed loss function is strictly convex and univariate with respect to each scaling parameter, enabling its efficient optimization through a simple second-order algorithm. Our method is versatile and can be readily adapted to various preference optimization frameworks, including direct preference optimization (DPO). Our experiments with robotic control and natural language generation with large language models (LLMs) show that our method not only improves policy performance but also aligns reward function selection more closely with policy optimization, simplifying the hyperparameter tuning process.
Efficient Reinforcement Learning by Discovering Neural Pathways
Samin Yeasar Arnob, Riyasat Ohib, Sergey Plis, Amy Zhang, Alessandro Sordoni, Doina Precup
Reinforcement learning (RL) algorithms have been very successful at tackling complex control problems, such as AlphaGo or fusion control. However, current research mainly emphasizes solution quality, often achieved by using large models trained on large amounts of data, and does not account for the financial, environmental, and societal costs associated with developing and deploying such models. Modern neural networks are often overparameterized and a significant number of parameters can be pruned without meaningful loss in performance, resulting in more efficient use of the model’s capacity lottery ticket. We present a methodology for identifying sub-networks within a larger network in reinforcement learning (RL). We call such sub-networks, neural pathways. We show empirically that even very small learned sub-networks, using less than 5% of the large network’s parameters, can provide very good quality solutions. We also demonstrate the training of multiple pathways within the same networks in a multitask setup, where each pathway is encouraged to tackle a separate task. We evaluate empirically our approach on several continuous control tasks, in both online and offline training
Mitigating Partial Observability in Decision Processes via the Lambda Discrepancy
Cameron Allen, Aaron Kirtland, Ruo Yu Tao, Sam Lobel, Daniel Scott, Nicholas Petrocelli, Omer Gottesman, Ronald Parr, Michael Littman, George Konidaris
Reinforcement learning algorithms typically rely on the assumption that the environment dynamics and value function can be expressed in terms of a Markovian state representation. However, when state information is only partially observable, how can an agent learn such a state representation, and how can it detect when it has found one? We introduce a metric that can accomplish both objectives, without requiring access to—or knowledge of—an underlying, unobservable state space. Our metric, the λ-discrepancy, is the difference between two distinct temporal difference (TD) value estimates, each computed using TD(λ) with a different value of λ. Since TD(λ=0) makes an implicit Markov assumption and TD(λ=1) does not, a discrepancy between these estimates is a potential indicator of a non-Markovian state representation. Indeed, we prove that the λ-discrepancy is exactly zero for all Markov decision processes and almost always non-zero for a broad class of partially observable environments. We also demonstrate empirically that, once detected, minimizing the λ-discrepancy can help with learning a memory function to mitigate the corresponding partial observability. We then train a reinforcement learning agent that simultaneously constructs two recurrent value networks with different λ parameters and minimizes the difference between them as an auxiliary loss. The approach scales to challenging partially observable domains, where the resulting agent frequently performs significantly better (and never performs worse) than a baseline recurrent agent with only a single value network.
Robust Reinforcement Learning from Corrupted Human Feedback
Alexander Bukharin, Ilgee Hong, Haoming Jiang, Zichong Li, Qingru Zhang, Zixuan Zhang, Tuo Zhao
Reinforcement learning from human feedback (RLHF) provides a principled framework for aligning AI systems with human preference data. For various reasons, e.g., personal bias, context ambiguity, lack of training, etc, human annotators may give incorrect or inconsistent preference labels. To tackle this challenge, we propose a robust RLHF approach — $R^3M$, which models the potentially corrupted preference label as sparse outliers. Accordingly, we formulate the robust reward learning as an $\ell_1$-regularized maximum likelihood estimation problem. Computationally, we develop an efficient alternating optimization algorithm, which only incurs negligible computational overhead compared with the standard RLHF approach. Theoretically, we prove that under proper regularity conditions, $R^3M$ can consistently learn the underlying reward and identify outliers, provided that the number of outlier labels scales sublinearly with the preference sample size. Furthermore, we remark that $R^3M$ is versatile and can be extended to various preference optimization methods, including direct preference optimization (DPO). Our experiments on robotic control and natural language generation with large language models (LLMs) show that $R^3M$ improves robustness of the reward against several types of perturbations to the preference data.
Attack-Resilient Image Watermarking Using Stable Diffusion
Lijun Zhang, Xiao Liu, Antoni Martin, Cindy Bearfield, Yuriy Brun, Hui Guan
Watermarking images is critical for tracking image provenance and proving ownership. With the advent of generative models, such as stable diffusion, that can create fake but realistic images, watermarking has become particularly important to make human-created images reliably identifiable. Unfortunately, the very same stable diffusion technology can remove watermarks injected using existing methods.To address this problem, we present ZoDiac, which uses a pre-trained stable diffusion model to inject a watermark into the trainable latent space, resulting in watermarks that can be reliably detected in the latent vector even when attacked. We evaluate ZoDiac on three benchmarks, MS-COCO, DiffusionDB, and WikiArt, and find that ZoDiac is robust against state-of-the-art watermark attacks, with a watermark detection rate above 98% and a false positive rate below 6.4%, outperforming state-of-the-art watermarking methods. We hypothesize that the reciprocating denoising process in diffusion models may inherently enhance the robustness of the watermark when faced with strong attacks and validate the hypothesis. Our research demonstrates that stable diffusion is a promising approach to robust watermarking, able to withstand even stable-diffusion-based attack methods. ZoDiac is open-sourced and available at https://github.com/zhanglijun95/ZoDiac.
Evaluating the design space of diffusion-based generative models
Yuqing Wang, Ye He, Molei Tao
Most existing theoretical investigations of the accuracy of diffusion models, albeit significant, assume the score function has been approximated to a certain accuracy, and then use this a priori bound to control the error of generation. This article instead provides a first quantitative understanding of the whole generation process, i.e., both training and sampling. More precisely, it conducts a non-asymptotic convergence analysis of denoising score matching under gradient descent. In addition, a refined sampling error analysis for variance exploding models is also provided. The combination of these two results yields a full error analysis, which elucidates (again, but this time theoretically) how to design the training and sampling processes for effective generation. For instance, our theory implies a preference toward noise distribution and loss weighting in training that qualitatively agree with the ones used in [Karras et al., 2022]. It also provides perspectives on the choices of time and variance schedules in sampling: when the score is well trained, the design in [Song et al., 2021] is more preferable, but when it is less trained, the design in [Karras et al., 2022] becomes more preferable.
RefDrop: Controllable Consistency in Image or Video Generation via Reference Feature Guidance
Jiaojiao Fan, Haotian Xue, Qinsheng Zhang, Yongxin Chen
There is a rapidly growing interest in controlling consistency across multiple generated images using diffusion models. Among various methods, recent works have found that simply manipulating attention modules by concatenating features from multiple reference images provides an efficient approach to enhancing consistency without fine-tuning. Despite its popularity and success, few studies have elucidated the underlying mechanisms that contribute to its effectiveness. In this work, we reveal that the popular approach is a linear interpolation of image self-attention and cross-attention between synthesized content and reference features, with a constant rank-1 coefficient. Motivated by this observation, we find that a rank-1 coefficient is not necessary and simplifies the controllable generation mechanism. The resulting algorithm, which we coin as RefDrop, allows users to control the influence of reference context in a direct and precise manner. Besides further enhancing consistency in single-subject image generation, our method also enables more interesting applications, such as the consistent generation of multiple subjects, suppressing specific features to encourage more diverse content, and high-quality personalized video generation by boosting temporal consistency. Even compared with state-of-the-art image-prompt-based generators, such as IP-Adapter, RefDrop is competitive in terms of controllability and quality while avoiding the need to train a separate image encoder for feature injection from reference images, making it a versatile plug-and-play solution for any image or video diffusion model.
Discrete and Combinatorial Optimization
On Sparse Canonical Correlation Analysis
Yongchun Li, Santanu Dey, Weijun Xie
The classical Canonical Correlation Analysis (CCA) identifies the correlations between two sets of multivariate variables based on their covariance, which has been widely applied in diverse fields such as computer vision, natural language processing, and speech analysis. Despite its popularity, CCA can encounter challenges in explaining correlations between two variable sets within high-dimensional data contexts. Thus, this paper studies Sparse Canonical Correlation Analysis (SCCA) that enhances the interpretability of CCA. We first show that SCCA generalizes three well-known sparse optimization problems, sparse PCA, sparse SVD, and sparse regression, which are all classified as NP-hard problems. This result motivates us to develop strong formulations and efficient algorithms. Our main contributions include (i) the introduction of a combinatorial formulation that captures the essence of SCCA and allows the development of exact and approximation algorithms; (ii) the establishment of the complexity results for two low-rank special cases of SCCA; and (iii) the derivation of an equivalent mixed-integer semidefinite programming model that facilitates a specialized branch-and-cut algorithm with analytical cuts. The effectiveness of our proposed formulations and algorithms is validated through numerical experiments.
Ahmad-Reza Ehyaei, Golnoosh Farnadi, Samira Samadi
In recent years, Wasserstein Distributionally Robust Optimization (DRO) has garnered substantial interest for its efficacy in data-driven decision-making under distributional uncertainty. However, limited research has explored the application of DRO to address individual fairness concerns, particularly when considering causal structures and discrete sensitive attributes in learning problems.To address this gap, we first formulate the DRO problem from the perspectives of causality and individual fairness. We then present the DRO dual formulation as an efficient tool to convert the main problem into a more tractable and computationally efficient form. Next, we characterize the closed form of the approximate worst-case loss quantity as a regularizer, eliminating the max-step in the Min-Max DRO problem. We further estimate the regularizer in more general cases and explore the relationship between DRO and classical robust optimization. Finally, by removing the assumption of a known structural causal model, we provide finite sample error bounds when designing DRO with empirical distributions and estimated causal structures to ensure efficiency and robust learning.
Bayesian Strategic Classification
Lee Cohen, Saeed Sharifi-Malvajerdi, Kevin Stangl, Ali Vakilian, Juba Ziani
In strategic classification, agents modify their features, at a cost, to obtain a positive classification outcome from the learner’s classifier, typically assuming agents have full knowledge of the deployed classifier. In contrast, we consider a Bayesian setting where agents have a common distributional prior on the classifier being used and agents manipulate their features to maximize their expected utility according to this prior.The learner can reveal truthful, yet not necessarily complete, information about the classifier to the agents, aiming to release just enough information to shape the agents’ behavior and thus maximize accuracy. We show that partial information release can counter-intuitively benefit the learner’s accuracy, allowing qualified agents to pass the classifier while preventing unqualified agents from doing so. Despite the intractability of computing the best response of an agent in the general case, we provide oracle-efficient algorithms for scenarios where the learner’s hypothesis class consists of low-dimensional linear classifiers or when the agents’ cost function satisfies a sub-modularity condition. Additionally, we address the learner’s optimization problem, offering both positive and negative results on determining the optimal information release to maximize expected accuracy, particularly in settings where an agent’s qualification can be represented by a real-valued number.
Online Relational Inference for Evolving Multi-agent Interacting Systems
Beomseok Kang, Priyabrata Saha, Sudarshan Sharma, Biswadeep Chakraborty, Saibal Mukhopadhyay
We introduce a novel framework, Online Relational Inference (ORI), designed to efficiently identify hidden interaction graphs in evolving multi-agent interacting systems using streaming data. Unlike traditional offline methods that rely on a fixed training set, ORI employs online backpropagation, updating the model with each new data point, thereby allowing it to adapt to changing environments in real-time. A key innovation is the use of an adjacency matrix as a trainable parameter, optimized through a new adaptive learning rate technique called AdaRelation, which adjusts based on the historical sensitivity of the decoder to changes in the interaction graph. Additionally, a data augmentation method named Trajectory Mirror (TM) is introduced to improve generalization by exposing the model to varied trajectory patterns. Experimental results on both synthetic datasets and real-world data (CMU MoCap for human motion) demonstrate that ORI significantly improves the accuracy and adaptability of relational inference in dynamic settings compared to existing methods. This approach is model-agnostic, enabling seamless integration with various neural relational inference (NRI) architectures, and offers a robust solution for real-time applications in complex, evolving systems.
Emotion-LLaMA: Multimodal Emotion Recognition and Reasoning with Instruction Tuning
Zebang Cheng, Zhi-Qi Cheng, Jun-Yan He, Kai Wang, Yuxiang Lin, Zheng Lian, Xiaojiang Peng, Alexander Hauptmann
Accurate emotion perception is crucial for various applications, including human-computer interaction, education, and counseling.However, traditional single-modality approaches often fail to capture the complexity of real-world emotional expressions, which are inherently multimodal. Moreover, existing Multimodal Large Language Models (MLLMs) face challenges in integrating audio and recognizing subtle facial micro-expressions. To address this, we introduce the MERR dataset, containing 28,618 coarse-grained and 4,487 fine-grained annotated samples across diverse emotional categories. This dataset enables models to learn from varied scenarios and generalize to real-world applications. Furthermore, we propose Emotion-LLaMA, a model that seamlessly integrates audio, visual, and textual inputs through emotion-specific encoders. By aligning features into a shared space and employing a modified LLaMA model with instruction tuning, Emotion-LLaMA significantly enhances both emotional recognition and reasoning capabilities. Extensive evaluations show Emotion-LLaMA outperforms other MLLMs, achieving top scores in Clue Overlap (7.83) and Label Overlap (6.25) on EMER, an F1 score of 0.9036 on MER2023-SEMI challenge, and the highest UAR (45.59) and WAR (59.37) in zero-shot evaluations on DFEW dataset.
Codec Avatar Studio: Paired Human Captures for Complete, Driveable, and Generalizable Avatars
Julieta Martinez, Emily Kim, Javier Romero, Timur Bagautdinov, Shunsuke Saito, Shoou-I Yu, Stuart Anderson, Michael Zollhöfer, Te-Li Wang, Shaojie Bai, Shih-En Wei, Rohan Joshi, Wyatt Borsos, Tomas Simon, Jason Saragih, Paul Theodosis, Alexander Greene, Anjani Josyula, Silvio Maeta, Andrew Jewett, Simion Venshtain, Christopher Heilman, Yueh-Tung Chen, Sidi Fu, Mohamed Elshaer, Tingfang Du, Longhua Wu, Shen-Chi Chen, Kai Kang, Michael Wu, Youssef Emad, Steven Longay, Ashley Brewer, Hitesh Shah, James Booth, Taylor Koska, Kayla Haidle, Joanna Hsu, Thomas Dauer, Peter Selednik, Tim Godisart, Scott Ardisson, Matthew Cipperly, Ben Humberston, Lon Farr, Bob Hansen, Peihong Guo, Dave Braun, Steven Krenn, He Wen, Lucas Evans, Natalia Fadeeva, Matthew Stewart, Gabriel Schwartz, Divam Gupta, Gyeongsik Moon, Kaiwen Guo, Yuan Dong, Yichen Xu, Takaaki Shiratori, Fabian Prada Nino, Bernardo Pires, Bo Peng, Julia Buffalini, Autumn Trimble, Kevyn McPhail, Melissa Schoeller, Yaser Sheikh
To build photorealistic avatars that users can embody, human modelling must be complete (cover the full body), driveable (able to reproduce the current motion and appearance from the user), and generalizable (_i.e._, easily adaptable to novel identities).Towards these goals, _paired_ captures, that is, captures of the same subject obtained from systems of diverse quality and availability, are crucial.However, paired captures are rarely available to researchers outside of dedicated industrial labs: _Codec Avatar Studio_ is our proposal to close this gap.Towards generalization and driveability, we introduce a dataset of 256 subjects captured in two modalities: high resolution multi-view scans of their heads, and video from the internal cameras of a headset.Towards completeness, we introduce a dataset of 4 subjects captured in eight modalities: high quality relightable multi-view captures of heads and hands, full body multi-view captures with minimal and regular clothes, and corresponding head, hands and body phone captures.Together with our data, we also provide code and pre-trained models for different state-of-the-art human generation models.We hope Codec Avatar Studio will serve as a toolkit to accelerate academic engagement with the core problems of telepresence.
Dense Associative Memory Through the Lens of Random Features
Benjamin Hoover, Duen Horng Chau, Hendrik Strobelt, Parikshit Ram, Dmitry Krotov
Dense Associative Memories are high storage capacity variants of the Hopfield networks that are capable of storing a large number of memory patterns in the weights of the network of a given size. Their common formulations typically require storing each pattern in a separate set of synaptic weights, which leads to the increase of the number of synaptic weights when new patterns are introduced. In this work we propose an alternative formulation of this class of models using random features, commonly used in kernel methods. In this formulation the number of network’s parameters remains fixed. At the same time, new memories can be added to the network by modifying existing weights. We show that this novel network closely approximates the energy function and dynamics of conventional Dense Associative Memories and shares their desirable computational properties.
AmoebaLLM: Constructing Any-Shape Large Language Models for Efficient and Instant Deployment
Yonggan Fu, Zhongzhi Yu, Junwei Li, Jiayi Qian, Yongan Zhang, Xiangchi Yuan, Dachuan Shi, Roman Yakunin, Yingyan (Celine) Lin
Motivated by the transformative capabilities of large language models (LLMs) across various natural language tasks, there has been a growing demand to deploy these models effectively across diverse real-world applications and platforms. However, the challenge of efficiently deploying LLMs has become increasingly pronounced due to the varying application-specific performance requirements and the rapid evolution of computational platforms, which feature diverse resource constraints and deployment flows. These varying requirements necessitate LLMs that can adapt their structures (depth and width) for optimal efficiency across different platforms and application specifications. To address this critical gap, we propose AmoebaLLM, a novel framework designed to enable the instant derivation of LLM subnets of arbitrary shapes, which achieve the accuracy-efficiency frontier and can be extracted immediately after a one-time fine-tuning. In this way, AmoebaLLM significantly facilitates rapid deployment tailored to various platforms and applications. Specifically, AmoebaLLM integrates three innovative components: (1) a knowledge-preserving subnet selection strategy that features a dynamic-programming approach for depth shrinking and an importance-driven method for width shrinking; (2) a shape-aware mixture of LoRAs to mitigate gradient conflicts among subnets during fine-tuning; and (3) an in-place distillation scheme with loss-magnitude balancing as the fine-tuning objective. Extensive experiments validate that AmoebaLLM not only sets new standards in LLM adaptability but also successfully delivers subnets that achieve state-of-the-art trade-offs between accuracy and efficiency.
Dual Lagrangian Learning for Conic Optimization
Mathieu Tanneau, Pascal Van Hentenryck
This paper presents Dual Lagrangian Learning (DLL), a principled learning methodology for dual conic optimization proxies.DLL leverages conic duality and the representation power of ML models to provide high-duality, dual-feasible solutions, and therefore valid Lagrangian dual bounds, for linear and nonlinear conic optimization problems.The paper introduces a systematic dual completion procedure, differentiable conic projection layers, and a self-supervised learning framework based on Lagrangian duality.It also provides closed-form dual completion formulae for broad classes of conic problems, which eliminate the need for costly implicit layers.The effectiveness of DLL is demonstrated on linear and nonlinear conic optimization problems.The proposed methodology significantly outperforms a state-of-the-art learning-based method, and achieves 1000x speedups over commercial interior-point solvers with optimality gaps under 0.5\% on average.
A Unifying Post-Processing Framework for Multi-Objective Learn-to-Defer Problems
Mohammad-Amin Charusaie, Samira Samadi
Learn-to-Defer is a paradigm that enables learning algorithms to work not in isolation but as a team with human experts. In this paradigm, we permit the system to defer a subset of its tasks to the expert. Although there are currently systems that follow this paradigm and are designed to optimize the accuracy of the final human-AI team, the general methodology for developing such systems under a set of constraints (e.g., algorithmic fairness, expert intervention budget, defer of anomaly, etc.) remains largely unexplored. In this paper, using a d-dimensional generalization to the fundamental lemma of Neyman and Pearson (d-GNP), we obtain the Bayes optimal solution for learn-to-defer systems under various constraints. Furthermore, we design a generalizable algorithm to estimate that solution and apply this algorithm to the COMPAS, Hatespeech, and ACSIncome datasets. Our algorithm shows improvements in terms of constraint violation over a set of learn-to-defer baselines and can control multiple constraint violations at once. The use of d-GNP is beyond learn-to-defer applications and can potentially obtain a solution to decision-making problems with a set of controlled expected performance measures.
High-dimensional (Group) Adversarial Training in Linear Regression
Yiling Xie, Xiaoming Huo
Adversarial training can achieve robustness against adversarial perturbations and has been widely used in machine-learning models. This paper delivers a non-asymptotic consistency analysis of the adversarial training procedure under $\ell_\infty$-perturbation in high-dimensional linear regression. It will be shown that, under the restricted eigenvalue condition, the associated convergence rate of prediction error can achieve the minimax rate up to a logarithmic factor in the high-dimensional linear regression on the class of sparse parameters. Additionally, the group adversarial training procedure is analyzed. Compared with classic adversarial training, it will be proved that the group adversarial training procedure enjoys a better prediction error upper bound under certain group-sparsity patterns.
Monte Carlo and Sampling Methods
A Separation in Heavy-Tailed Sampling: Gaussian vs. Stable Oracles for Proximal Samplers
Ye He, Alireza Mousavi-Hosseini, Krishnakumar Balasubramanian, Murat Erdogdu
We study the complexity of heavy-tailed sampling and present a separation result in terms of obtaining high-accuracy versus low-accuracy guarantees i.e., samplers that require only $\mathcal{O}(\log(1/\varepsilon))$ versus $\Omega(\text{poly}(1/\varepsilon))$ iterations to output a sample which is $\varepsilon$-close to the target in $\chi^2$-divergence. Our results are presented for proximal samplers that are based on Gaussian versus stable oracles. We show that proximal samplers based on the Gaussian oracle have a fundamental barrier in that they necessarily achieve only low-accuracy guarantees when sampling from a class of heavy-tailed targets. In contrast, proximal samplers based on the stable oracle exhibit high-accuracy guarantees, thereby overcoming the aforementioned limitation. We also prove lower bounds for samplers under the stable oracle and show that our upper bounds cannot be fundamentally improved.
Ye He, Kevin Rojas, Molei Tao
This paper considers the problem of sampling from non-logconcave distribution, based on queries of its unnormalized density. It first describes a framework, Denoising Diffusion Monte Carlo (DDMC), based on the simulation of a denoising diffusion process with its score function approximated by a generic Monte Carlo estimator. DDMC is an oracle-based meta-algorithm, where its oracle is the assumed access to samples that generate a Monte Carlo score estimator. Then we provide an implementation of this oracle, based on rejection sampling, and this turns DDMC into a true algorithm, termed Zeroth-Order Diffusion Monte Carlo (ZOD-MC). We provide convergence analyses by first constructing a general framework, i.e. a performance guarantee for DDMC, without assuming the target distribution to be log-concave or satisfying any isoperimetric inequality. Then we prove that ZOD-MC admits an inverse polynomial dependence on the desired sampling accuracy, albeit still suffering from the curse of dimensionality. Consequently, for low dimensional distributions, ZOD-MC is a very efficient sampler, with performance exceeding latest samplers, including also-denoising-diffusion-based RDMC and RSDMC. Last, we experimentally demonstrate the insensitivity of ZOD-MC to increasingly higher barriers between modes or discontinuity in non-convex potential.
CuMo: Scaling Multimodal LLM with Co-Upcycled Mixture-of-Experts
Jiachen Li, Xinyao Wang, Sijie Zhu, Chia-Wen Kuo, Lu XU, Fan Chen, Jitesh Jain, Humphrey Shi, Longyin Wen
Recent advancements in Multimodal Large Language Models (LLMs) have focused primarily on scaling by increasing text-image pair data and enhancing LLMs to improve performance on multimodal tasks. However, these scaling approaches are computationally expensive and overlook the significance of efficiently improving model capabilities from the vision side. Inspired by the successful applications of Mixture-of-Experts (MoE) in LLMs, which improves model scalability during training while keeping inference costs similar to those of smaller models, we propose CuMo, which incorporates Co-upcycled Top-K sparsely-gated Mixture-of-experts blocks into both the vision encoder and the MLP connector, thereby enhancing the multimodal LLMs with neglectable additional activated parameters during inference.CuMo first pre-trains the MLP blocks and then initializes each expert in the MoE block from the pre-trained MLP block during the visual instruction tuning stage, with auxiliary losses to ensure a balanced loading of experts.CuMo outperforms state-of-the-art multimodal LLMs across various VQA and visual-instruction-following benchmarks within each model size group, all while training exclusively on open-sourced datasets.
Grounding Multimodal Large Language Models in Actions
Andrew Szot, Bogdan Mazoure, Harsh Agrawal, R Devon Hjelm, Zsolt Kira, Alexander Toshev
Multimodal Large Language Models (MLLMs) have demonstrated a wide range of capabilities across many domains including Embodied AI. In this work, we study how to best ground a MLLM into different embodiments and their associated action spaces, including both continuous and discrete actions. For continuous actions, a set of learned tokenizations that capture an action at various resolutions allows for sufficient modeling precision, yielding the best performance on downstream tasks. For discrete actions, semantically aligning these actions with the native output token space of the MLLM leads to the strongest performance. We arrive at these lessons via a thorough study of seven action grounding approaches on five different environments, encompassing over 114 embodied tasks.
Learning Spatially-Aware Language and Audio Embeddings
Bhavika Devnani, Skyler Seto, Zakaria Aldeneh, Alessandro Toso, Elena Menyaylenko, Barry-John Theobald, Jonathan Sheaffer, Miguel Sarabia
Humans can picture a sound scene given an imprecise natural language description. For example, it is easy to imagine an acoustic environment given a phrase like “the lion roar came from right behind me!”. For a machine to have the same degree of comprehension, the machine must know what a lion is (semantic attribute), what the concept of “behind” is (spatial attribute) and how these pieces of linguistic information align with the semantic and spatial attributes of the sound (what a roar sounds like when its coming from behind). State-of-the-art audio foundation models, such as CLAP, which learn to map between audio scenes and natural textual descriptions, are trained on non-spatial audio and text pairs, and hence lack spatial awareness. In contrast, sound event localization and detection models are limited to recognizing sounds from a fixed number of classes, and they localize the source to absolute position (e.g., 0.2m) rather than a position described using natural language (e.g., “next to me”). To address these gaps, we present ELSA (Embeddings for Language and Spatial Audio), a spatially aware-audio and text embedding model trained using multimodal contrastive learning. ELSA supports non-spatial audio, spatial audio, and open vocabulary text captions describing both the spatial and semantic components of sound. To train ELSA: (a) we spatially augment the audio and captions of three open-source audio datasets totaling 4,738 hours and 890,038 samples of audio comprised from 8,972 simulated spatial configurations, and (b) we design an encoder to capture the semantics of non-spatial audio, and the semantics and spatial attributes of spatial audio using contrastive learning. ELSA is a single model that is competitive with state-of-the-art for both semantic retrieval and 3D source localization. In particular, ELSA achieves +2.8\% mean audio-to-text and text-to-audio R@1 above the LAION-CLAP baseline, and outperforms by -11.6° mean-absolute-error in 3D source localization over the SeldNET baseline on the TUT Sound Events 2018 benchmark. Moreover, we show that the representation-space of ELSA is structured, enabling swapping of direction of audio via vector arithmetic of two directional text embeddings.
Neuroscience, Cognitive Science
Active design of two-photon holographic stimulation for identifying neural population dynamics
Andrew Wagenmaker, Lu Mi, Marton Rozsa, Matthew Bull, Karel Svoboda, Kayvon Daie, Matthew Golub, Kevin Jamieson
Recent advances in techniques for monitoring and perturbing neural populations have greatly enhanced our ability to study circuits in the brain. In particular, two-photon holographic optogenetics now enables precise photostimulation of experimenter-specified groups of individual neurons, while simultaneous two-photon calcium imaging enables the measurement of ongoing and induced activity across the neural population. Despite the enormous space of potential photostimulation patterns and the time-consuming nature of photostimulation experiments, very little algorithmic work has been done to determine the most effective photostimulation patterns for identifying the neural population dynamics. Here, we develop methods to efficiently select which neurons to stimulate such that the resulting neural responses will best inform a dynamical model of the neural population activity. Using neural population responses to photostimulation in mouse motor cortex, we demonstrate the efficacy of a low-rank linear dynamical systems model, and develop an active learning procedure which takes advantage of low-rank structure to determine informative photostimulation patterns. We demonstrate our approach on both real and synthetic data, obtaining in some cases as much as a two-fold reduction in the amount of data required to reach a given predictive power. Our active stimulation design method is based on a novel active learning procedure for low-rank regression, which may be of independent interest.
Exploring Behavior-Relevant and Disentangled Neural Dynamics with Generative Diffusion Models
Yule Wang, Chengrui Li, Weihan Li, Anqi Wu
Understanding the neural basis of behavior is a fundamental goal in neuroscience. Current research in large-scale neuro-behavioral data analysis often relies on decoding models, which quantify behavioral information in neural data but lack details on behavior encoding. This raises an intriguing scientific question: “how can we enable in-depth exploration of neural representations in behavioral tasks, revealing interpretable neural dynamics associated with behaviors”. However, addressing this issue is challenging due to the varied behavioral encoding across different brain regions and mixed selectivity at the population level. To tackle this limitation, our approach, named (“BeNeDiff”), first identifies a fine-grained and disentangled neural subspace using a behavior-informed latent variable model. It then employs state-of-the-art generative diffusion models to synthesize behavior videos that interpret the neural dynamics of each latent factor. We validate the method on multi-session datasets containing widefield calcium imaging recordings across the dorsal cortex. Through guiding the diffusion model to activate individual latent factors, we verify that the neural dynamics of latent factors in the disentangled neural subspace provide interpretable quantifications of the behaviors of interest. At the same time, the neural subspace in BeNeDiff demonstrates high disentanglement and neural reconstruction quality.
Inferring stochastic low-rank recurrent neural networks from neural data
Matthijs Pals, A Erdem Sağtekin, Felix Pei, Manuel Gloeckler, Jakob H Macke
A central aim in computational neuroscience is to relate the activity of large populations of neurons to an underlying dynamical system. Models of these neural dynamics should ideally be both interpretable and fit the observed data well. Low-rank recurrent neural networks (RNNs) exhibit such interpretability by having tractable dynamics. However, it is unclear how to best fit low-rank RNNs to data consisting of noisy observations of an underlying stochastic system. Here, we propose to fit stochastic low-rank RNNs with variational sequential Monte Carlo methods. We validate our method on several datasets consisting of both continuous and spiking neural data, where we obtain lower dimensional latent dynamics than current state of the art methods. Additionally, for low-rank models with piecewise linear nonlinearities, we show how to efficiently identify all fixed points in polynomial rather than exponential cost in the number of units, making analysis of the inferred dynamics tractable for large RNNs. Our method both elucidates the dynamical systems underlying experimental recordings and provides a generative model whose trajectories match observed variability.
SPOTLIGHT Latent Diffusion for Neural Spiking Data
Jaivardhan Kapoor, Auguste Schulz, Julius Vetter, Felix Pei, Richard Gao, Jakob H Macke
Modern datasets in neuroscience enable unprecedented inquiries into the relationship between complex behaviors and the activity of many simultaneously recorded neurons. While latent variable models can successfully extract low-dimensional embeddings from such recordings, using them to generate realistic spiking data, especially in a behavior-dependent manner, still poses a challenge. Here, we present Latent Diffusion for Neural Spiking data (LDNS), a diffusion-based generative model with a low-dimensional latent space: LDNS employs an autoencoder with structured state-space (S4) layers to project discrete high-dimensional spiking data into continuous time-aligned latents. On these inferred latents, we train expressive (conditional) diffusion models, enabling us to sample neural activity with realistic single-neuron and population spiking statistics. We validate LDNS on synthetic data, accurately recovering latent structure, firing rates, and spiking statistics. Next, we demonstrate its flexibility by generating variable-length data that mimics human cortical activity during attempted speech. We show how to equip LDNS with an expressive observation model that accounts for single-neuron dynamics not mediated by the latent state, further increasing the realism of generated samples. Finally, conditional LDNS trained on motor cortical activity during diverse reaching behaviors can generate realistic spiking data given reach direction or unseen reach trajectories. In summary, LDNS simultaneously enables inference of low-dimensional latents and realistic conditional generation of neural spiking datasets, opening up further possibilities for simulating experimentally testable hypotheses.
Probabilistic Decomposed Linear Dynamical Systems for Robust Discovery of Latent Neural Dynamics
Yenho Chen, Noga Mudrik, Kyle A. Johnsen, Sankaraleengam Alagapan, Adam Charles, Christopher Rozell
Time-varying linear state-space models are powerful tools for obtaining mathematically interpretable representations of neural signals. For example, switching and decomposed models describe complex systems using latent variables that evolve according to simple locally linear dynamics. However, existing methods for latent variable estimation are not robust to dynamical noise and system nonlinearity due to noise-sensitive inference procedures and limited model formulations. This can lead to inconsistent results on signals with similar dynamics, limiting the model’s ability to provide scientific insight. In this work, we address these limitations and propose a probabilistic approach to latent variable estimation in decomposed models that improves robustness against dynamical noise. Additionally, we introduce an extended latent dynamics model to improve robustness against system nonlinearities. We evaluate our approach on several synthetic dynamical systems, including an empirically-derived brain-computer interface experiment, and demonstrate more accurate latent variable inference in nonlinear systems with diverse noise conditions. Furthermore, we apply our method to a real-world clinical neurophysiology dataset, illustrating the ability to identify interpretable and coherent structure where previous models cannot.
Towards a “Universal Translator” for Neural Dynamics at Single-Cell, Single-Spike Resolution
Yizi Zhang, Yanchen Wang, Donato Jiménez-Benetó, Zixuan Wang, Mehdi Azabou, Blake Richards, Renee Tung, Olivier Winter, Brain Laboratory International, Eva Dyer, Liam Paninski, Cole Hurwitz
Neuroscience research has made immense progress over the last decade, but our understanding of the brain remains fragmented and piecemeal: the dream of probing an arbitrary brain region and automatically reading out the information encoded in its neural activity remains out of reach. In this work, we build towards a first foundation model for neural spiking data that can solve a diverse set of tasks across multiple brain areas. We introduce a novel self-supervised modeling approach for population activity in which the model alternates between masking out and reconstructing neural activity across different time steps, neurons, and brain regions. To evaluate our approach, we design unsupervised and supervised prediction tasks using the International Brain Laboratory repeated site dataset, which is comprised of Neuropixels recordings targeting the same brain locations across 48 animals and experimental sessions. The prediction tasks include single-neuron and region-level activity prediction, forward prediction, and behavior decoding. We demonstrate that our multi-task-masking (MtM) approach significantly improves the performance of current state-of-the-art population models and enables multi-task learning. We also show that by training on multiple animals, we can improve the generalization ability of the model to unseen animals, paving the way for a foundation model of the brain at single-cell, single-spike resolution.
HYDRA: Model Factorization Framework for Black-Box LLM Personalization
Yuchen Zhuang, Haotian Sun, Yue Yu, Rushi Qiang, Qifan Wang, Chao Zhang, Bo Dai
Personalization has emerged as a critical research area in modern intelligent systems, focusing on mining users’ behavioral history and adapting to their preferences for delivering tailored experiences. Despite the remarkable few-shot capabilities exhibited by black-box large language models (LLMs), the inherent opacity of their model parameters presents significant challenges in aligning the generated output with individual expectations. Existing solutions have primarily focused on prompt design to incorporate user-specific profiles and behaviors; however, such approaches often struggle to generalize effectively due to their inability to capture shared knowledge among all users. To address these challenges, we propose HYDRA, a model factorization framework that captures both user-specific behavior patterns from historical data and shared general knowledge among all users to deliver personalized generation. In order to capture user-specific behavior patterns, we first train a reranker to prioritize the most useful information from top-retrieved relevant historical records.By combining the prioritized history with the corresponding query, we train an adapter to align the output with individual user-specific preferences, eliminating the reliance on access to inherent model parameters of black-box LLMs. Both the reranker and the adapter can be decomposed into a base model with multiple user-specific heads, resembling a hydra. The base model maintains shared knowledge across users, while the multiple personal heads capture user-specific preferences. Experimental results demonstrate that \method outperforms existing state-of-the-art prompt-based methods by an average relative improvement of 9.01% across five diverse personalization tasks in the LaMP benchmark.
Zhenghao Xu, Yuqing Wang, Tuo Zhao, Rachel Ward, Molei Tao
We study the convergence rate of first-order methods for rectangular matrix factorization, which is a canonical nonconvex optimization problem. Specifically, given a rank-$r$ matrix $\mathbf{A}\in\mathbb{R}^{m\times n}$, we prove that gradient descent (GD) can find a pair of $\epsilon$-optimal solutions $\mathbf{X}_T\in\mathbb{R}^{m\times d}$ and $\mathbf{Y}_T\in\mathbb{R}^{n\times d}$, where $d\geq r$, satisfying $\lVert\mathbf{X}_T\mathbf{Y}_T^\top-\mathbf{A}\rVert_F\leq\epsilon\lVert\mathbf{A}\rVert_F$ in $T=O(\kappa^2\log\frac{1}{\epsilon})$ iterations with high probability, where $\kappa$ denotes the condition number of $\mathbf{A}$. Furthermore, we prove that Nesterov’s accelerated gradient (NAG) attains an iteration complexity of $O(\kappa\log\frac{1}{\epsilon})$, which is the best-known bound of first-order methods for rectangular matrix factorization. Different from small balanced random initialization in the existing literature, we adopt an unbalanced initialization, where $\mathbf{X}_0$ is large and $\mathbf{Y}_0$ is $0$. Moreover, our initialization and analysis can be further extended to linear neural networks, where we prove that NAG can also attain an accelerated linear convergence rate. In particular, we only require the width of the network to be greater than or equal to the rank of the output label matrix. In contrast, previous results achieving the same rate require excessive widths that additionally depend on the condition number and the rank of the input data matrix.
Quantitative Convergences of Lie Group Momentum Optimizers
Lingkai Kong, Molei Tao
Explicit, momentum-based dynamics that optimize functions defined on Lie groups can be constructed via variational optimization and momentum trivialization. Structure preserving time discretizations can then turn this dynamics into optimization algorithms. This article investigates two types of discretization, Lie Heavy-Ball, which is a known splitting scheme, and Lie NAG-SC, which is newly proposed. Their convergence rates are explicitly quantified under $L$-smoothness and \emph{local} strong convexity assumptions. Lie NAG-SC provides acceleration over the momentumless case, i.e. Riemannian gradient descent, but Lie Heavy-Ball does not. When compared to existing accelerated optimizers for general manifolds, both Lie Heavy-Ball and Lie NAG-SC are computationally cheaper and easier to implement, thanks to their utilization of group structure. Only gradient oracle and exponential map are required, but not logarithm map or parallel transport which are computational costly.
SPOTLIGHT Sample Complexity of Posted Pricing for a Single Item
Billy Jin, Thomas Kesselheim, Will Ma, Sahil Singla
Selling a single item to $n$ self-interested bidders is a fundamental problem in economics, where the two objectives typically considered are welfare maximization and revenue maximization. Since the optimal auctions are often impractical and do not work for sequential bidders, posted pricing auctions, where fixed prices are set for the item for different bidders, have emerged as a practical and effective alternative. This paper investigates how many samples are needed from bidders’ value distributions to find near-optimal posted prices, considering both independent and correlated bidder distributions, and welfare versus revenue maximization. We obtain matching upper and lower bounds (up to logarithmic terms) on the sample complexity for all these settings.
Certified Machine Unlearning via Noisy Stochastic Gradient Descent
Eli Chien, Haoyu Wang, Ziang Chen, Pan Li
“The right to be forgotten” ensured by laws for user data privacy becomes increasingly important. Machine unlearning aims to efficiently remove the effect of certain data points on the trained model parameters so that it can be approximately the same as if one retrains the model from scratch. We propose to leverage projected noisy stochastic gradient descent for unlearning and establish its first approximate unlearning guarantee under the convexity assumption. Our approach exhibits several benefits, including provable complexity saving compared to retraining, and supporting sequential and batch unlearning. Both of these benefits are closely related to our new results on the infinite Wasserstein distance tracking of the adjacent (un)learning processes. Extensive experiments show that our approach achieves a similar utility under the same privacy constraint while using $2\%$ and $10\%$ of the gradient computations compared with the state-of-the-art gradient-based approximate unlearning methods for mini-batch and full-batch settings, respectively.
Differentially Private Graph Diffusion with Applications in Personalized PageRanks
Rongzhe Wei, Eli Chien, Pan Li
Graph diffusion, which iteratively propagates real-valued substances among the graph, is used in numerous graph/network-involved applications. However, releasing diffusion vectors may reveal sensitive linking information in the data such as transaction information in financial network data. However, protecting the privacy of graph data is challenging due to its interconnected nature. This work proposes a novel graph diffusion framework with edge-level different privacy guarantees by using noisy diffusion iterates. The algorithm injects Laplace noise per diffusion iteration and adopts a degree-based thresholding function to mitigate the high sensitivity induced by low-degree nodes. Our privacy loss analysis is based on Privacy Amplification by Iteration (PABI), which to our best knowledge, is the first effort that analyzes PABI with Laplace noise and provides relevant applications. We also introduce a novel $\infty$-Wasserstein distance tracking method, which tightens the analysis of privacy leakage and makes PABI more applicable in practice. We evaluate this framework by applying it to Personalized Pagerank computation for ranking tasks. Experiments on real-world network data demonstrate the superiority of our method under stringent privacy conditions.
SPOTLIGHT Langevin Unlearning: A New Perspective of Noisy Gradient Descent for Machine Unlearning
Eli Chien, Haoyu Wang, Ziang Chen, Pan Li
Machine unlearning has raised significant interest with the adoption of laws ensuring the “right to be forgotten”. Researchers have provided a probabilistic notion of approximate unlearning under a similar definition of Differential Privacy (DP), where privacy is defined as statistical indistinguishability to retraining from scratch. We propose Langevin unlearning, an unlearning framework based on noisy gradient descent with privacy guarantees for approximate unlearning problems. Langevin unlearning unifies the DP learning process and the privacy-certified unlearning process with many algorithmic benefits. These include approximate certified unlearning for non-convex problems, complexity saving compared to retraining, sequential and batch unlearning for multiple unlearning requests.
DARG: Dynamic Evaluation of Large Language Models via Adaptive Reasoning Graph
Zhehao Zhang, Jiaao Chen, Diyi Yang
The current paradigm of evaluating Large Language Models (LLMs) through static benchmarks comes with significant limitations, such as vulnerability to data contamination and a lack of adaptability to the evolving capabilities of LLMs. Therefore, evaluation methods that can adapt and generate evaluation data with controlled complexity are urgently needed. In this work, we introduce Dynamic Evaluation of LLMs via Adaptive Reasoning Graph Evolvement (DARG) to dynamically extend current benchmarks with controlled complexity and diversity. Specifically, we first extract the reasoning graphs of data points in current benchmarks and then perturb the reasoning graphs to generate novel testing data. Such newly generated test samples can have different levels of complexity while maintaining linguistic diversity similar to the original benchmarks. We further use a code-augmented LLM to ensure the label correctness of newly generated data. We apply our DARG framework to diverse reasoning tasks in four domains with 15 state-of-the-art LLMs. Experimental results show that almost all LLMs experience a performance decrease with increased complexity and certain LLMs exhibit significant drops. Additionally, we find that LLMs exhibit more biases when being evaluated via the data generated by DARG with higher complexity levels. These observations provide useful insights into how to dynamically and adaptively evaluate LLMs.
SPOTLIGHT Pre-trained Text-to-Image Diffusion Models Are Versatile Representation Learners for Control
Gunshi Gupta, Karmesh Yadav, Yarin Gal, Dhruv Batra, Zsolt Kira, Cong Lu, Tim G. J. Rudner
Embodied AI agents require a fine-grained understanding of the physical world mediated through visual and language inputs. Such capabilities are difficult to learn solely from task-specific data. This has led to the emergence of pre-trained vision-language models as a tool for transferring representations learned from internet-scale data to downstream tasks and new domains. However, commonly used contrastively trained representations such as in CLIP have been shown to fail at enabling embodied agents to gain a sufficiently fine-grained scene understanding—a capability vital for control. To address this shortcoming, we consider representations from pre-trained text-to-image diffusion models, which are explicitly optimized to generate images from text prompts and as such, contain text-conditioned representations that reflect highly fine-grained visuo-spatial information. Using pre-trained text-to-image diffusion models, we construct Stable Control Representations which allow learning downstream control policies that generalize to complex, open-ended environments. We show that policies learned using Stable Control Representations are competitive with state-of-the-art representation learning approaches across a broad range of simulated control settings, encompassing challenging manipulation and navigation tasks. Most notably, we show that Stable Control Representations enable learning policies that exhibit state-of-the-art performance on OVMM, a difficult open-vocabulary navigation benchmark.
What Variables Affect Out-of-Distribution Generalization in Pretrained Models?
Md Yousuf Harun, Kyungbok Lee, Gianmarco Gallardo, Giri Krishnan, Christopher Kanan
Embeddings produced by pre-trained deep neural networks (DNNs) are widely used; however, their efficacy for downstream tasks can vary widely. We study the factors influencing transferability and out-of-distribution (OOD) generalization of pre-trained DNN embeddings through the lens of the tunnel effect hypothesis, which is closely related to intermediate neural collapse. This hypothesis suggests that deeper DNN layers compress representations and hinder OOD generalization. Contrary to earlier work, our experiments show this is not a universal phenomenon. We comprehensively investigate the impact of DNN architecture, training data, image resolution, and augmentations on transferability. We identify that training with high-resolution datasets containing many classes greatly reduces representation compression and improves transferability. Our results emphasize the danger of generalizing findings from toy datasets to broader contexts.
Diffusion Policy Attacker: Crafting Adversarial Attacks for Diffusion-based Policies
Yipu Chen, Haotian Xue, Yongxin Chen
Diffusion models have emerged as a promising approach for behavior cloning (BC), leveraging their exceptional ability to model multi-modal distributions. Diffusion policies (DP) have elevated BC performance to new heights, demonstrating robust efficacy across diverse tasks, coupled with their inherent flexibility and ease of implementation. Despite the increasing adoption of Diffusion Policies (DP) as a foundation for policy generation, the critical issue of safety remains largely unexplored. While previous attempts have targeted deep policy networks, DP used diffusion models as the policy network, making it ineffective to be attacked using previous methods because of its chained structure and randomness injected. In this paper, we undertake a comprehensive examination of DP safety concerns by introducing adversarial scenarios, encompassing offline and online attacks, global and patch-based attacks. We propose DP-Attacker, a suite of algorithms that can craft effective adversarial attacks across all aforementioned scenarios. We conduct attacks on pre-trained diffusion policies across various manipulation tasks. Through extensive experiments, we demonstrate that DP-Attacker has the capability to significantly decrease the success rate of DP for all scenarios. Particularly in offline scenarios, we exhibit the generation of highly transferable perturbations applicable to all frames. Furthermore, we illustrate the creation of adversarial physical patches that, when applied to the environment, effectively deceive the model. Video results areput in: https://sites.google.com/view/dp-attacker-videos/.
QueST: Self-Supervised Skill Abstractions for Learning Continuous Control
Atharva Mete, Haotian Xue, Albert Wilcox, Yongxin Chen, Animesh Garg
Generalization capabilities, or rather a lack thereof, is one of the most important unsolved problems in the field of robot learning, and while several large scale efforts have set out to tackle this problem, unsolved it remains. In this paper, we hypothesize that learning temporal action abstractions using latent variable models (LVMs), which learn to map data to a compressed latent space and back, is apromising direction towards low-level skills that can readily be used for new tasks. Although several works have attempted to show this, they have generally been limited by architectures that do not faithfully capture sharable representations. To address this we present Quantized Skill Transformer (QueST), which learns a larger and more flexible latent encoding that is more capable of modeling the breadth of low-level skills necessary for a variety of tasks. To make use of this extra flexibility, QueST imparts causal inductive bias from the action sequence data into the latent space, leading to more semantically useful and transferable representations. We compare to state-of-the-art imitation learning and LVM baselines and see that QueST’s architecture leads to strong performance on several multitask and few-shot learning benchmarks. Further results and videos are available at https://quest-model.github.io.
RadarOcc: Robust 3D Occupancy Prediction with 4D Imaging Radar
Fangqiang Ding, Xiangyu Wen, Yunzhou Zhu, Yiming Li, Chris Xiaoxuan Lu
3D occupancy-based perception pipeline has significantly advanced autonomous driving by capturing detailed scene descriptions and demonstrating strong generalizability across various object categories and shapes. Current methods predominantly rely on LiDAR or camera inputs for 3D occupancy prediction. These methods are susceptible to adverse weather conditions, limiting the all-weather deployment of self-driving cars. To improve perception robustness, we leverage the recent advances in automotive radars and introduce a novel approach that utilizes 4D imaging radar sensors for 3D occupancy prediction. Our method, RadarOcc, circumvents the limitations of sparse radar point clouds by directly processing the 4D radar tensor, thus preserving essential scene details. RadarOcc innovatively addresses the challenges associated with the voluminous and noisy 4D radar data by employing Doppler bins descriptors, sidelobe-aware spatial sparsification, and range-wise self-attention mechanisms. To minimize the interpolation errors associated with direct coordinate transformations, we also devise a spherical-based feature encoding followed by spherical-to-Cartesian feature aggregation. We benchmark various baseline methods based on distinct modalities on the public K-Radar dataset. The results demonstrate RadarOcc’s state-of-the-art performance in radar-based 3D occupancy prediction and promising results even when compared with LiDAR- or camera-based methods. Additionally, we present qualitative evidence of the superior performance of 4D radar in adverse weather conditions and explore the impact of key pipeline components through ablation studies.
Your contrastive learning problem is secretly a distribution alignment problem
Zihao Chen, Chi-Heng Lin, Ran Liu, Jingyun Xiao, Eva Dyer
Despite the success of contrastive learning (CL) in vision and language, its theoretical foundations and mechanisms for building representations remain poorly understood. In this work, we build connections between noise contrastive estimation losses widely used in CL and distribution alignment with entropic optimal transport (OT). This connection allows us to develop a family of different losses and multistep iterative variants for existing CL methods. Intuitively, by using more information from the distribution of latents, our approach allows a more distribution-aware manipulation of the relationships within augmented sample sets.We provide theoretical insights and experimental evidence demonstrating the benefits of our approach for generalized contrastive alignment. Through this framework, it is possible to leverage tools in OT to build unbalanced losses to handle noisy views and customize the representation space by changing the constraints on alignment.By reframing contrastive learning as an alignment problem and leveraging existing optimization tools for OT, our work provides new insights and connections between different self-supervised learning models in addition to new tools that can be more easily adapted to incorporate domain knowledge into learning.
Self-Taught Recognizer: Toward Unsupervised Adaptation for Speech Foundation Models
Yuchen Hu, CHEN CHEN, Chao-Han Yang, Chengwei Qin, Pin-Yu Chen, Eng-Siong Chng, Chao Zhang
We propose an unsupervised adaptation framework, Self-TAught Recognizer (STAR), which leverages unlabeled data to enhance the robustness of automatic speech recognition (ASR) systems in diverse target domains, such as noise and accents. STAR is developed for prevalent speech foundation models based on Transformer-related architecture with auto-regressive decoding (e.g., Whisper, Canary). Specifically, we propose a novel indicator that empirically integrates step-wise information during decoding to assess the token-level quality of pseudo labels without ground truth, thereby guiding model updates for effective unsupervised adaptation. Experimental results show that STAR achieves an average of 13.5% relative reduction in word error rate across 14 target domains, and it sometimes even approaches the upper-bound performance of supervised adaptation. Surprisingly, we also observe that STAR prevents the adapted model from the common catastrophic forgetting problem without recalling source-domain data. Furthermore, STAR exhibits high data efficiency that only requires less than one-hour unlabeled data, and seamless generality to alternative large speech models and speech translation tasks. Our code aims to open source to the research communities.
Chieh-Yun Chen, Chiang Tseng, Li-Wu Tsao, Hong-Han Shuai
This paper analyzes the impact of causal manner in the text encoder of text-to-image (T2I) diffusion models, which can lead to information bias and loss. Previous works have focused on addressing the issues through the denoising process. However, there is no research discussing how text embedding contributes to T2I models, especially when generating more than one object. In this paper, we share a comprehensive analysis of text embedding: i) how text embedding contributes to the generated images and ii) why information gets lost and biases towards the first-mentioned object. Accordingly, we propose a simple but effective text embedding balance optimization method, which is training-free, with an improvement of 125.42\% on information balance in stable diffusion. Furthermore, we propose a new automatic evaluation metric that quantifies information loss more accurately than existing methods, achieving 81\% concordance with human assessments. This metric effectively measures the presence and accuracy of objects, addressing the limitations of current distribution scores like CLIP’s text-image similarities.
Precise asymptotics of reweighted least-squares algorithms for linear diagonal networks
Chiraag Kaushik, Justin Romberg, Vidya Muthukumar
The classical iteratively reweighted least-squares (IRLS) algorithm aims to recover an unknown signal from linear measurements by performing a sequence of weighted least squares problems, where the weights are recursively updated at each step. Varieties of this algorithm have been shown to achieve favorable empirical performance and theoretical guarantees for sparse recovery and $\ell_p$-norm minimization. Recently, some preliminary connections have also been made between IRLS and certain types of non-convex linear neural network architectures that are observed to exploit low-dimensional structure in high-dimensional linear models. In this work, we provide a unified asymptotic analysis for a family of algorithms that encompasses IRLS, the recently proposed lin-RFM algorithm (which was motivated by feature learning in neural networks), and the alternating minimization algorithm on linear diagonal neural networks. Our analysis operates in a “batched” setting with i.i.d. Gaussian covariates and shows that, with appropriately chosen reweighting policy, the algorithm can achieve favorable performance in only a handful of iterations. We also extend our results to the case of group-sparse recovery and show that leveraging this structure in the reweighting scheme provably improves test error compared to coordinate-wise reweighting.
Alexander Havrilla, Wenjing Liao
When training deep neural networks, a model’s generalization error is often observed to follow a power scaling law dependent both on the model size and the data size. Perhaps the best known example of such scaling laws are for transformer-based large language models (**LLMs**), where networks with billions of parameters are trained on trillions of tokens of text. Yet, despite sustained widespread interest, a rigorous understanding of why transformer scaling laws exist is still missing. To answer this question, we establish novel statistical estimation and mathematical approximation theories for transformers when the input data are concentrated on a low-dimensional manifold. Our theory predicts a power law between the generalization error and both the training data size and the network size for transformers, where the power depends on the intrinsic dimension $d$ of the training data. Notably, the constructed model architecture is shallow, requiring only logarithmic depth in $d$. By leveraging low-dimensional data structures under a manifold hypothesis, we are able to explain transformer scaling laws in a way which respects the data geometry. Moreover, we test our theory with empirical observation by training LLMs on natural language datasets. We find the observed empirical scaling laws closely agree with our theoretical predictions. Taken together, these results rigorously show the intrinsic dimension of data to be a crucial quantity affecting transformer scaling laws in both theory and practice.
When are dynamical systems learned from time series data statistically accurate?
Jeongjin Park, Nicole Yang, Nisha Chandramoorthy
Conventional notions of generalization often fail to describe the ability of learned models to capture meaningful information from dynamical data. A neural network that learns complex dynamics with a small test error may still fail to reproduce its \emph{physical} behavior, including associated statistical moments and Lyapunov exponents. To address this gap, we propose an ergodic theoretic approach to generalization of complex dynamical models learned from time series data. Our main contribution is to define and analyze generalization of a broad suite of neural representations of classes of ergodic systems, including chaotic systems, in a way that captures emulating underlying invariant, physical measures. Our results provide theoretical justification for why regression methods for generators of dynamical systems (Neural ODEs) fail to generalize, and why their statistical accuracy improves upon adding Jacobian information during training. We verify our results on a number of ergodic chaotic systems and neural network parameterizations, including MLPs, ResNets, Fourier Neural layers, and RNNs.
Large Pre-trained time series models for cross-domain Time series analysis tasks
Harshavardhan Prabhakar Kamarthi, B. Aditya Prakash
Large pre-trained models have been vital in recent advancements in domains like language and vision, making model training for individual downstream tasks more efficient and provide superior performance. However, tackling time-series analysis tasks usually involves designing and training a separate model from scratch leveraging training data and domain expertise specific to the task. We tackle a significant challenge for pre-training a foundational time-series model from multi-domain time-series datasets: extracting semantically useful tokenized inputs to the model across heterogeneous time-series from different domains. We propose Large Pre-trained Time-series Models (LPTM) that introduces a novel method of adaptive segmentation that automatically identifies optimal dataset-specific segmentation strategy during pre-training. This enables LPTM to perform similar to or better than domain-specific state-of-art model when fine-tuned to different downstream time-series analysis tasks and under zero-shot settings. LPTM achieves superior forecasting and time-series classification results taking up to 40% less data and 50% less training time compared to state-of-art baselines.
Time-MMD: A New Multi-Domain Multimodal Dataset for Time Series Analysis
Haoxin Liu, Shangqing Xu, Zhiyuan Zhao, Lingkai Kong, Harshavardhan Prabhakar Kamarthi, Aditya Sasanur, Megha Sharma, Jiaming Cui, Qingsong Wen, Chao Zhang, B. Aditya Prakash
Time series data are ubiquitous across a wide range of real-world domains. While real-world time series analysis (TSA) requires human experts to integrate numerical series data with multimodal domain-specific knowledge, most existing TSA models rely solely on numerical data, overlooking the significance of information beyond numerical series. This oversight is due to the untapped potential of textual series data and the absence of a comprehensive, high-quality multimodal dataset. To overcome this obstacle, we introduce Time-MMD, the first multi-domain, multimodal time series dataset covering 9 primary data domains. Time-MMD ensures fine-grained modality alignment, eliminates data contamination, and provides high usability. Additionally, we develop MM-TSFlib, the first multimodal time series forecasting (TSF) library, seamlessly pipelining multimodal TSF evaluations based on Time-MMD for in-depth analyses. Extensive experiments conducted on Time-MMD through MM-TSFlib demonstrate significant performance enhancements by extending unimodal TSF to multimodality, evidenced by over 15\% mean squared error reduction in general, and up to 40\% in domains with rich textual data. More importantly, our datasets and library revolutionize broader applications, impacts, research topics to advance TSA. The dataset and library are available at https://github.com/AdityaLab/Time-MMD and https://github.com/AdityaLab/MM-TSFlib.
Aligning Large Language Models with Representation Editing: A Control Perspective
Lingkai Kong, Haorui Wang, Wenhao Mu, Yuanqi Du, Yuchen Zhuang, Yifei Zhou, Yue Song, Rongzhi Zhang, Kai Wang, Chao Zhang
Aligning large language models (LLMs) with human objectives is crucial for real-world applications. However, fine-tuning LLMs for alignment often suffers from unstable training and requires substantial computing resources. Test-time alignment techniques, such as prompting and guided decoding, do not modify the underlying model, and their performance remains dependent on the original model’s capabilities. To address these challenges, we propose aligning LLMs through representation editing. The core of our method is to view a pre-trained autoregressive LLM as a discrete-time stochastic dynamical system. To achieve alignment for specific objectives, we introduce external control signals into the state space of this language dynamical system. We train a value function directly on the hidden states according to the Bellman equation, enabling gradient-based optimization to obtain the optimal control signals at test time. Our experiments demonstrate that our method outperforms existing test-time alignment techniques while requiring significantly fewer resources compared to fine-tuning methods. Our code is available at [https://github.com/Lingkai-Kong/RE-Control](https://github.com/Lingkai-Kong/RE-Control).
Designs for Enabling Collaboration in Human-Machine Teaming via Interactive and Explainable Systems
Rohan Paleja, Michael Munje, Kimberlee Chang, Reed Jensen, Matthew Gombolay
Collaborative robots and machine learning-based virtual agents are increasingly entering the human workspace with the aim of increasing productivity and enhancing safety. Despite this, we show in a ubiquitous experimental domain, Overcooked-AI, that state-of-the-art techniques for human-machine teaming (HMT), which rely on imitation or reinforcement learning, are brittle and result in a machine agent that aims to decouple the machine and human’s actions to act independently rather than in a synergistic fashion. To remedy this deficiency, we develop HMT approaches that enable iterative, mixed-initiative team development allowing end-users to interactively reprogram interpretable AI teammates. Our 50-subject study provides several findings that we summarize into guidelines. While all approaches underperform a simple collaborative heuristic (a critical, negative result for learning-based methods), we find that white-box approaches supported by interactive modification can lead to significant team development, outperforming white-box approaches alone, and that black-box approaches are easier to train and result in better HMT performance highlighting a tradeoff between explainability and interactivity versus ease-of-training. Together, these findings present three important future research directions: 1) Improving the ability to generate collaborative agents with white-box models, 2) Better learning methods to facilitate collaboration rather than individualized coordination, and 3) Mixed-initiative interfaces that enable users, who may vary in ability, to improve collaboration.
Lisa: Lazy Safety Alignment for Large Language Models against Harmful Fine-tuning Attack
Tiansheng Huang, Sihao Hu, Fatih Ilhan, Selim Tekin, Ling Liu
Recent studies show that Large Language Models (LLMs) with safety alignment can be jail-broken by fine-tuning on a dataset mixed with harmful data. For the first time in the literature, we show that the jail-break effect can be mitigated by separating two states in the fine-tuning stage to respectively optimize over the alignment and user datasets. Unfortunately, our subsequent study shows that this simple Bi-State Optimization (BSO) solution experiences convergence instability when steps invested in its alignment state is too small, leading to downgraded alignment performance. By statistical analysis, we show that the \textit{excess drift} towards the switching iterates of the two states could be a probable reason for the instability. To remedy this issue, we propose \textbf{L}azy(\textbf{i}) \textbf{s}afety \textbf{a}lignment (\textbf{Lisa}), which introduces a proximal term to constraint the drift of each state. Theoretically, the benefit of the proximal term is supported by the convergence analysis, wherein we show that a sufficient large proximal factor is necessary to guarantee Lisa’s convergence. Empirically, our results on four downstream fine-tuning tasks show that Lisa with a proximal term can significantly increase alignment performance while maintaining the LLM’s accuracy on the user tasks. Code is available at https://github.com/git-disl/Lisa.
Navigating the Safety Landscape: Measuring Risks in Finetuning Large Language Models
ShengYun Peng, Pin-Yu Chen, Matthew Hull, Duen Horng Chau
Safety alignment is crucial to ensure that large language models (LLMs) behave in ways that align with human preferences and prevent harmful actions during inference. However, recent studies show that the alignment can be easily compromised through finetuning with only a few adversarially designed training examples. We aim to measure the risks in finetuning LLMs through navigating the LLM safety landscape. We discover a new phenomenon observed universally in the model parameter space of popular open-source LLMs, termed as “safety basin”: random perturbations to model weights maintain the safety level of the original aligned model within its local neighborhood. However, outside this local region, safety is fully compromised, exhibiting a sharp, step-like drop. This safety basin contrasts sharply with the LLM capability landscape, where model performance peaks at the origin and gradually declines as random perturbation increases. Our discovery inspires us to propose the new VISAGE safety metric that measures the safety in LLM finetuning by probing its safety landscape. Visualizing the safety landscape of the aligned model enables us to understand how finetuning compromises safety by dragging the model away from the safety basin. The LLM safety landscape also highlights the system prompt’s critical role in protecting a model, and that such protection transfers to its perturbed variants within the safety basin. These observations from our safety landscape research provide newinsights for future work on LLM safety community. Our code is publicly available at https://github.com/ShengYun-Peng/llm-landscape.
SPOTLIGHT Selective Generation for Controllable Language Models
Minjae Lee, Kyungmin Kim, Taesoo Kim, Sangdon Park
Trustworthiness of generative language models (GLMs) is crucial in their deployment to critical decision making systems. Hence, certified risk control methods such as selective prediction and conformal prediction have been applied to mitigating the hallucination problem in various supervised downstream tasks. However, the lack of appropriate correctness metric hinders applying such principled methods to language generation tasks. In this paper, we circumvent this problem by leveraging the concept of textual entailment to evaluate the correctness of the generated sequence, and propose two selective generation algorithms which control the false discovery rate with respect to the textual entailment relation (FDR-E) with a theoretical guarantee: $\texttt{SGen}^{\texttt{Sup}}$ and $\texttt{SGen}^{\texttt{Semi}}$. $\texttt{SGen}^{\texttt{Sup}}$, a direct modification of the selective prediction, is a supervised learning algorithm which exploits entailment-labeled data, annotated by humans. Since human annotation is costly, we further propose a semi-supervised version, $\texttt{SGen}^{\texttt{Semi}}$, which fully utilizes the unlabeled data by pseudo-labeling, leveraging an entailment set function learned via conformal prediction. Furthermore, $\texttt{SGen}^{\texttt{Semi}}$ enables to use more general class of selection functions, neuro-selection functions, and provides users with an optimal selection function class given multiple candidates. Finally, we demonstrate the efficacy of the $\texttt{SGen}$ family in achieving a desired FDR-E level with comparable selection efficiency to those from baselines on both open and closed source GLMs. Code and datasets are provided at https://github.com/ml-postech/selective-generation.
The Group Robustness is in the Details: Revisiting Finetuning under Spurious Correlations
Tyler LaBonte, John Hill, Xinchen Zhang, Vidya Muthukumar, Abhishek Kumar
Modern machine learning models are prone to over-reliance on spurious correlations, which can often lead to poor performance on minority groups. In this paper, we identify surprising and nuanced behavior of finetuned models on worst-group accuracy via comprehensive experiments on four well-established benchmarks across vision and language tasks. We first show that the commonly used class-balancing techniques of mini-batch upsampling and loss upweighting can induce a decrease in worst-group accuracy (WGA) with training epochs, leading to performance no better than without class-balancing. While in some scenarios, removing data to create a class-balanced subset is more effective, we show this depends on group structure and propose a mixture method which can outperform both techniques. Next, we show that scaling pretrained models is generally beneficial for worst-group accuracy, but only in conjunction with appropriate class-balancing. Finally, we identify spectral imbalance in finetuning features as a potential source of group disparities — minority group covariance matrices incur a larger spectral norm than majority groups once conditioned on the classes. Our results show more nuanced interactions of modern finetuned models with group robustness than was previously known. Our code is available at https://github.com/tmlabonte/revisiting-finetuning.
Vaccine: Perturbation-aware Alignment for Large Language Models against Harmful Fine-tuning Attack
Tiansheng Huang, Sihao Hu, Ling Liu
The new paradigm of fine-tuning-as-a-service introduces a new attack surface for Large Language Models (LLMs): a few harmful data uploaded by users can easily trick the fine-tuning to produce an alignment-broken model. We conduct an empirical analysis and uncovera \textit{harmful embedding drift} phenomenon, showing a probable cause of the alignment-broken effect. Inspired by our findings, we propose Vaccine, a perturbation-aware alignment technique to mitigate the security risk of users fine-tuning. The core idea of Vaccine is to produce invariant hidden embeddings by progressively adding crafted perturbation to them in the alignment phase. This enables the embeddings to withstand harmful perturbation from un-sanitized user data in the fine-tuning phase. Our results on open source mainstream LLMs (e.g., Llama2, Opt, Vicuna) demonstrate that Vaccine can boost the robustness of alignment against harmful prompts induced embedding drift while reserving reasoning ability towards benign prompts. Our code is available at https://github.com/git-disl/Vaccine.
Derivative-enhanced Deep Operator Network
Yuan Qiu, Nolan Bridges, Peng Chen
The deep operator networks (DeepONet), a class of neural operators that learn mappings between function spaces, have recently been developed as surrogate models for parametric partial differential equations (PDEs). In this work we propose a derivative-enhanced deep operator network (DE-DeepONet), which leverages derivative information to enhance the solution prediction accuracy and provides a more accurate approximation of solution-to-parameter derivatives, especially when training data are limited. DE-DeepONet explicitly incorporates linear dimension reduction of high dimensional parameter input into DeepONet to reduce training cost and adds derivative loss in the loss function to reduce the number of required parameter-solution pairs. We further demonstrate that the use of derivative loss can be extended to enhance other neural operators, such as the Fourier neural operator (FNO). Numerical experiments validate the effectiveness of our approach.
Diffusion Spectral Representation for Reinforcement Learning
Dmitry Shribak, Chen-Xiao Gao, Yitong Li, Chenjun Xiao, Bo Dai
Diffusion-based models have achieved notable empirical successes in reinforcement learning (RL) due to their expressiveness in modeling complex distributions. Despite existing methods being promising, the key challenge of extending existing methods for broader real-world applications lies in the computational cost at inference time, i.e., sampling from a diffusion model is considerably slow as it often requires tens to hundreds of iterations to generate even one sample. To circumvent this issue, we propose to leverage the flexibility of diffusion models for RL from a representation learning perspective. In particular, by exploiting the connection between diffusion models and energy-based models, we develop Diffusion Spectral Representation (Diff-SR), a coherent algorithm framework that enables extracting sufficient representations for value functions in Markov decision processes (MDP) and partially observable Markov decision processes (POMDP). We further demonstrate how Diff-SR facilitates efficient policy optimization and practical algorithms while explicitly bypassing the difficulty and inference cost of sampling from the diffusion model. Finally, we provide comprehensive empirical studies to verify the benefits of Diff-SR in delivering robust and advantageous performance across various benchmarks with both fully and partially observable settings.
FineStyle: Fine-grained Controllable Style Personalization for Text-to-image Models
Gong Zhang, Kihyuk Sohn, Meera Hahn, Humphrey Shi, Irfan Essa
Few-shot fine-tuning of text-to-image (T2I) generation models enables people to create unique images in their own style using natural languages without requiring extensive prompt engineering. However, fine-tuning with only a handful, as little as one, of image-text paired data prevents fine-grained control of style attributes at generation. In this paper, we present FineStyle, a few-shot fine-tuning method that allows enhanced controllability for style personalized text-to-image generation. To overcome the lack of training data for fine-tuning, we propose a novel concept-oriented data scaling that amplifies the number of image-text pair, each of which focuses on different concepts (e.g., objects) in the style reference image. We also identify the benefit of parameter-efficient adapter tuning of key and value kernels of cross-attention layers. Extensive experiments show the effectiveness of FineStyle at following fine-grained text prompts and delivering visual quality faithful to the specified style, measured by CLIP scores and human raters.
GeSS: Benchmarking Geometric Deep Learning under Scientific Applications with Distribution Shifts
Deyu Zou, Shikun Liu, Siqi Miao, Victor Fung, Shiyu Chang, Pan Li
Geometric deep learning (GDL) has gained significant attention in scientific fields, for its proficiency in modeling data with intricate geometric structures. Yet, very few works have delved into its capability of tackling the distribution shift problem, a prevalent challenge in many applications.To bridge this gap, we propose GeSS, a comprehensive benchmark designed for evaluating the performance of GDL models in scientific scenarios with distribution shifts.Our evaluation datasets cover diverse scientific domains from particle physics, materials science to biochemistry, and encapsulate a broad spectrum of distribution shifts including conditional, covariate, and concept shifts. Furthermore, we study three levels of information access from the out-of-distribution (OOD) test data, including no OOD information, only unlabeled OOD data, and OOD data with a few labels. Overall, our benchmark results in 30 different experiment settings, and evaluates 3 GDL backbones and 11 learning algorithms in each setting. A thorough analysis of the evaluation results is provided, poised to illuminate insights for GDL researchers and domain practitioners who are to use GDL in their applications.
SPOTLIGHT In-and-Out: Algorithmic Diffusion for Sampling Convex Bodies
Yunbum Kook, Santosh Vempala, Matthew Zhang
We present a new random walk for uniformly sampling high-dimensional convex bodies. It achieves state-of-the-art runtime complexity with stronger guarantees on the output than previously known, namely in Rényi divergence (which implies TV, $\mathcal{W}_2$, KL, $\chi^2$). The proof departs from known approaches for polytime algorithms for the problem – we utilize a stochastic diffusion perspective to show contraction to the target distribution with the rate of convergence determined by functional isoperimetric constants of the stationary density.
Large Spatial Model: End-to-end Unposed Images to Semantic 3D
Zhiwen Fan, Jian Zhang, Wenyan Cong, Peihao Wang, Renjie Li, Kairun Wen, Shijie Zhou, Achuta Kadambi, Zhangyang "Atlas" Wang, Danfei Xu, Boris Ivanovic, Marco Pavone, Yue Wang
Reconstructing and understanding 3D structures from a limited number of images is a classical problem in computer vision. Traditional approaches typically decompose this task into multiple subtasks, involving several stages of complex mappings between different data representations. For example, dense reconstruction using Structure-from-Motion (SfM) requires transforming images into key points, optimizing camera parameters, and estimating structures. Following this, accurate sparse reconstructions are necessary for further dense modeling, which is then input into task-specific neural networks. This multi-stage paradigm leads to significant processing times and engineering complexity.In this work, we introduce the Large Spatial Model (LSM), which directly processes unposed RGB images into semantic radiance fields. LSM simultaneously estimates geometry, appearance, and semantics in a single feed-forward pass and can synthesize versatile label maps by interacting through language at novel views. Built on a general Transformer-based framework, LSM predicts global geometry via pixel-aligned point maps. To improve spatial attribute regression, we adopt local context aggregation with multi-scale fusion, enhancing the accuracy of fine local details. To address the scarcity of labeled 3D semantic data and enable natural language-driven scene manipulation, we incorporate a pre-trained 2D language-based segmentation model into a 3D-consistent semantic feature field. An efficient decoder parameterizes a set of semantic anisotropic Gaussians, allowing supervised end-to-end learning. Comprehensive experiments on various tasks demonstrate that LSM unifies multiple 3D vision tasks directly from unposed images, achieving real-time semantic 3D reconstruction for the first time.
Zixuan Zhang, Kaiqi Zhang, Minshuo Chen, Yuma Takeda, Mengdi Wang, Tuo Zhao, Yu-Xiang Wang
Convolutional residual neural networks (ConvResNets), though overparametersized, can achieve remarkable prediction performance in practice, which cannot be well explained by conventional wisdom. To bridge this gap, we study the performance of ConvResNeXts trained with weight decay, which cover ConvResNets as a special case, from the perspective of nonparametric classification. Our analysis allows for infinitely many building blocks in ConvResNeXts, and shows that weight decay implicitly enforces sparsity on these blocks. Specifically, we consider a smooth target function supported on a low-dimensional manifold, then prove that ConvResNeXts can adapt to the function smoothness and low-dimensional structures and efficiently learn the function without suffering from the curse of dimensionality. Our findings partially justify the advantage of overparameterized ConvResNeXts over conventional machine learning models.
RankRAG: Unifying Context Ranking with Retrieval-Augmented Generation in LLMs
Yue Yu, Wei Ping, Zihan Liu, Boxin Wang, Jiaxuan You, Chao Zhang, Mohammad Shoeybi, Bryan Catanzaro
Large language models (LLMs) typically utilize the top-k contexts from a retriever in retrieval-augmented generation (RAG). In this work, we propose a novel method called RankRAG, which instruction-tunes a single LLM for both context ranking and answer generation in RAG. In particular, the instruction-tuned LLMs work surprisingly well by adding a small fraction of ranking data into the training blend, and outperform existing expert ranking models, including the same LLM exclusively fine-tuned on a large amount of ranking data. For generation, we compare our model with many strong baselines, including ChatQA-1.5, an open-sourced model with the state-of-the-art performance on RAG benchmarks. Specifically, our Llama3-RankRAG-8B and Llama3-RankRAG-70B significantly outperform Llama3-ChatQA-1.5-8B and Llama3-ChatQA-1.5-70B, respectively, on nine general knowledge-intensive benchmarks for RAG. In addition, it also performs comparably to GPT-4 on five RAG benchmarks in the biomedical domain without instruction fine-tuning on biomedical data, demonstrating its superb capability for generalization to new domains.
Regularizing Hidden States Enables Learning Generalizable Reward Model for LLMs
Rui Yang, Ruomeng Ding, Yong Lin, Huan Zhang, Tong Zhang
Reward models trained on human preference data have been proven to effectively align Large Language Models (LLMs) with human intent within the framework of reinforcement learning from human feedback (RLHF). However, current reward models have limited generalization capabilities to unseen prompts and responses, which can lead to an unexpected phenomenon known as reward over-optimization, resulting in a decline in actual performance due to excessive optimization of rewards. While previous research has advocated for constraining policy optimization, our study introduces a novel approach to enhance the reward model’s generalization ability against distribution shifts by regularizing the hidden states. Specifically, we retain the base model’s language model head and incorporate a suite of text-generation losses to preserve the hidden states’ text-generation capabilities, while concurrently learning a reward head behind the same hidden states. Our experimental results demonstrate that the introduced regularization technique markedly improves the accuracy of learned reward models across a variety of out-of-distribution (OOD) tasks and effectively alleviates the over-optimization issue in RLHF, offering a more reliable and robust preference learning paradigm.
Rethinking Weight Decay for Robust Fine-Tuning of Foundation Models
Junjiao Tian, Chengyue Huang, Zsolt Kira
Modern optimizers such as AdamW, equipped with momentum and adaptive learning rate, are designed to escape local minima and explore the vast parameter space. This exploration is beneficial for finding good loss basins when training from scratch. It is not necessarily ideal when resuming from a powerful foundation model because it can lead to large deviations from the pre-trained initialization and, consequently, worse robustness and generalization. At the same time, strong regularization on all parameters can lead to under-fitting. We hypothesize that selectively regularizing the parameter space is the key to fitting and retraining the pre-trained knowledge. This paper proposes a new weight decay technique, Selective Projection Decay (SPD), that selectively imposes a strong penalty on certain layers while allowing others to change freely. Intuitively, SPD expands and contracts the parameter search space for layers with consistent and inconsistent loss reduction, respectively. Experimentally, when equipped with SPD, Adam consistently provides better in-distribution generalization and out-of-distribution robustness performance on multiple popular vision and language benchmarks.
S$^{2}$FT: Efficient, Scalable and Generalizable LLM Fine-tuning by Structured Sparsity
Xinyu Yang, Jixuan Leng, Geyang Guo, Jiawei Zhao, Ryumei Nakada, Linjun Zhang, Huaxiu Yao, Beidi Chen
Current PEFT methods for LLMs can achieve either high quality, efficient training, or scalable serving, but not all three simultaneously. To address this limitation, we investigate sparse fine-tuning and observe a remarkable improvement in generalization ability. Utilizing this key insight, we propose a family of Structured Sparse Fine-Tuning (S${^2}$FT) methods for LLMs, which concurrently achieve state-of-the-art fine-tuning performance, training efficiency, and inference scalability. S${^2}$FT accomplishes this by selecting sparsely and computing densely. It selects a few heads and channels in the MHA and FFN modules for each Transformer Block, respectively. Next, it co-permutes weight matrices on both sides of the coupled structures in LLMs to connect the selected components in each layer into a dense submatrix. Finally, \model performs in-place gradient updates on all submatrices. Through theoretical analysis and empirical results, our method prevents overfitting and forgetting, delivers SOTA performance on both commonsense and arithmetic reasoning with 4.6% and 1.3% average improvements compared to LoRA, and outperforms full FT by 11.5% when generalize to various domains after instruction tuning. By integrating our partial back-propagation algorithm, \model saves the fine-tuning memory up to 3$\times$ and improves the latency by 1.5-2.7$\times$ compared to full FT, while delivering an average 10% improvement over LoRA on both metrics. We further demonstrate that S${^2}$FT can be decoupled into adapters, enabling effective fusion, fast switch, and efficient parallelism for serving multiple fine-tuned models.
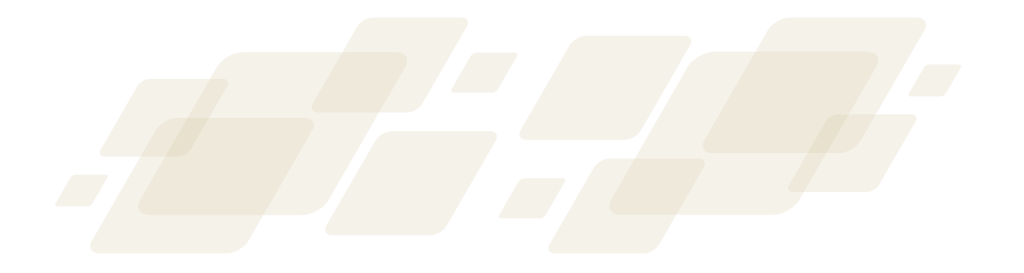
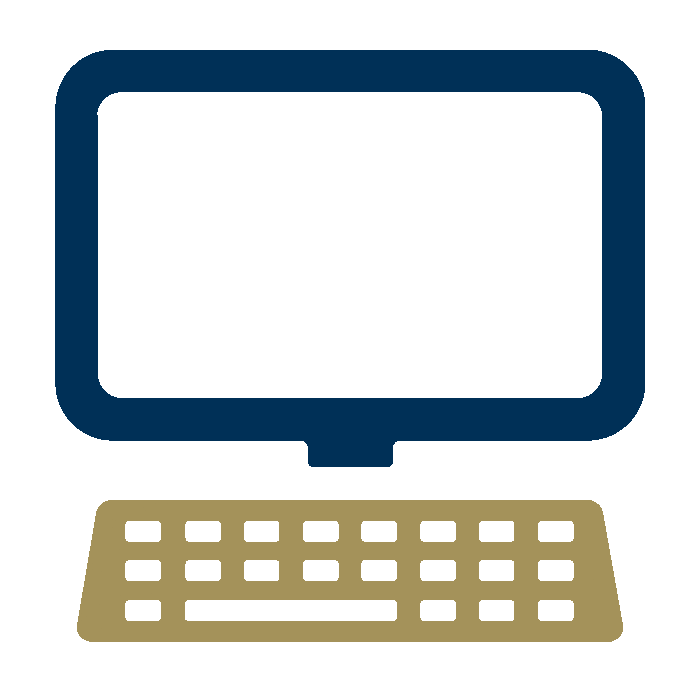
See you in Vancouver!
Development: College of Computing
Project Lead/Data Graphics: Joshua Preston
News: Bryant Wine
Featured Photography: Kevin Beasley, Terence Rushin
Data Management: Joni Isbell
Data: https://neurips.cc/