My research involves the creation of industrial engineering and operations research (IE/OR) methodologies to answer decision-making problems arising in public health and medicine.
I currently have three main streams of research: medical decision-making, healthcare access, infectious disease prevention and control. My work is motivated by addressing fundamental problems in these areas arising from challenges in maternal health, poliovirus, and chronic disease management with previous work on COVID-19. From a methodological perspective, I leverage data-driven optimization, Markov decision processes, agent-based modeling, simulation, and predictive models.
More details about each of these areas are provided below.
Maternal Health
Medical Decision Making
In this work, we are creating machine learning and decision-analytic models to help clinical decision-making throughout the perinatal period. I am working with Dr. Garcia, collaborators within Emory University’s Department of Obstetrics and Gynecology, and a group of PhD students
Check out this related paper:
Support for this work came from an Imagine, Innovate, and Impact (I3) Emory School of Medicine/Georgia Tech internal grant through the Georgia CTSA NIH award (UL1-TR002378) and the National Science Foundation through a Graduate Research Fellowship awarded to Ph.D. student, Meghan Meredith.
Health systems improvement
The maternal mortality rate in the United States is higher than that of other industrialized nations and is quickly growing (up 89% from 2018) with staggering racial/ethnic and urban/rural disparities.
The reasons for the maternal mortality crisis are complex and often are due to factors that span across multiple levels of influence, including patient-, provider-, community-, and health system-level factors. I am working with my Ph.D. student to analyze systems-level interventions, such as perinatal regionalization strategies.
Here is a related paper:
- The implications of using maternity care deserts to measure progress in access to obstetric care: a mixed-integer optimization analysis. Meghan Meredith, Lauren N. Steimle, Stephanie Radke. BMC Health Services Research 24, 682 (2024).
Recent work in this area is being supported by supported by a seed grant through the National Center for Advancing Translational Sciences of the National Institutes of Health under Award Number UL1TR002378.
Chronic Disease Management
Medical Decision-Making
Some of my papers on chronic disease management and/or MDPs include:
- Interpretable Policies and the Price of Interpretability in Hypertension Treatment Planning. Gian G. Garcia, Lauren N. Steimle, Wesley J. Marrero, and Jeremy B. Sussman. Manufacturing & Service Operations Management (Accepted).
- Decomposition methods for solving Markov decision processes with multiple models of the parameters. Lauren N. Steimle, Vinayak S. Ahluwalia*, Charmee Kamdar*, Brian T. Denton. IISE Transactions 53.12 (2021): 1295-1310. [PDF]
- Best Paper, IISE Transactions Focus Issue on Operations Engineering and Analytics, 2022
- Multi-model Markov decision processes. Lauren N. Steimle, David L. Kaufman, and Brian T. Denton. IISE Transactions 53.10 (2021): 1124-1139.
- Policy-based branch-and-bound for infinite-horizon multi-model Markov decision processes. Vinayak S. Ahluwalia*, Lauren N. Steimle, Brian T. Denton. Computers and Operations Research, 126 (2021): 105108. [PDF]
- Benefit and harm of intensive blood pressure treatment: Derivation and validation of risk models using data from the SPRINT and ACCORD trials. Sanjay Basu, Jeremy B. Sussman, Joseph Rigdon, Lauren N. Steimle, Brian T. Denton, Rodney A. Hayward. PLoS Medicine 14.10 (2017): e1002410.
- The Implications of State Aggregation in Markov Decision Processes for Medical Decision Making. Madeleine Pollack, Lauren N. Steimle. (Working Paper)
- Robust Markov decision processes for medical treatment decisions.Yuanhui Zhang, Lauren N. Steimle, Brian T. Denton. (Working Paper).
This work was supported by the National Science Foundation under grant numbers DGE-1256260 (Steimle’s NSF GRFP) and CMMI-1462060 (Denton).
Healthcare Access
With my PhD student Abel Sapirstein, I am using similar mathematical models to study access to tertiary cancer care in Rwanda. Rwandans experience significant delays in the diagnosis of cancer, in part because of challenges in accessing cancer care.
This work is supported by an NDSEG Fellowship awarded to my PhD student Abel Sapirstein.
Here is a related paper:
- Towards Expanded Access to Cancer Care with Cost-Awareness: An Optimization Modeling Analysis of Rwanda. Abel Sapirstein, Lauren N. Steimle, Cristina Stefan.
Infectious Disease Outbreak Prevention & Control
Mathematical modeling and analytics for responding to COVID-19

Much of my work in this area was aimed at helping institutions of higher education identify strategies for students to safely return to campus in the face of COVID-19. These decisions involve complex trade-offs and have to be made despite uncertainty about the future. The main goals of this project were to (1) create analytical tools for campus planners to make informed return-to-campus decisions and (2) generate general insights around the impact of these operational decisions on risk to students, faculty, and staff.
Check out the latest tools available on our team’s Github page.
Some papers on COVID-19 include:
- Empirical networks for localized COVID-19 interventions using WiFi infrastructure at university campuses. Vedant Das Swain, Jiajia Xie*, Maanit Madan, Sonia Sargolzaei, James Cai, Munmun De Choudhury, Gregory D. Abowd, Lauren N. Steimle and B. Aditya Prakash. Frontiers in Digital Health (2023), 5, 1060828.
- Multi-criteria Course Mode Selection and Classroom Assignment Under Sudden Space Scarcity Mehran Navabi-Shirazi*, Mohamed El Tonbari, Natashia Boland, Dima Nazzal, and Lauren N. Steimle. Manufacturing & Service Operations Management, (2022) 24(6):3252-3268.
- Finalist, INFORMS Service Science Best Cluster Paper Award (Check out our video)
- The Impact of Testing Capacity and Compliance with Isolation on Covid-19: A Mathematical Modeling Study. Zhuoting Yu, Pinar Keskinocak, Lauren N. Steimle, Inci Yildirim. AJPM Focus (2022), 100006.
- Students’ preferences for returning to colleges and universities during the COVID-19 pandemic: A discrete choice experiment. Lauren N. Steimle, Yuming Sun*, Lauren Johnson*, Tibor Besedeš, Patricia Mokhtarian, Dima Nazzal. Socio-Economic Planning Sciences, 82PB (2022), 101266.
- Revisiting the Small-World Property of Co-enrollment Networks: A Network Analysis of Hybrid Course Delivery Strategies. Di Wu, Hanna Hamilton, Liam Jagrowski, Dima Nazzal, Lauren N. Steimle. (Socio-Economic Planning Sciences, (Accepted).
This work was supported by a seed grant from the ISYE Thos and Clair Muller Endowment Fund and the Georgia Tech EVPR COVID-19 Rapid Response Seed Grant Program.
Informing Global Polio Eradication Efforts Through Innovative Modeling Approaches
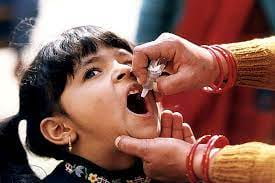
“Perhaps the biggest threat to [polio eradication] now is an explosion of vaccine-derived polio outbreaks in Africa that affected almost two dozen countries last year and paralyzed more than 500 children in 2020 and again in 2021”.
— From Science Magazine, 2022
While the Global Polio Eradication Initiative has successfully eradicated two of the three serotypes of poliovirus, a major challenge in achieving complete eradication is outbreaks of “circulating vaccine-derived poliovirus” (cVDPV). cVDPV outbreaks are caused when the oral poliovirus vaccine used to control outbreaks reverts to a form that has similar properties to wild poliovirus. I am working with Dr. Keskinocak and a great team of students to create mathematical models and analytical techniques that can be used to inform poliovirus outbreak prevention and response.
Check out this related paper:
- Outbreak response strategies to eliminate circulating vaccine-derived poliovirus: A case study of Serotype 2 in Northern Nigeria. Yuming Sun, Pinar Keskinocak, Lauren N. Steimle, Stephanie Kovacs, Steve Wassilak.Vaccine X (Accepted).
- A Compartmental Simulation Model to Improve Interventions for Controlling Poliovirus Outbreaks. Yuming Sun*, Pinar Keskinocak, Lauren N. Steimle, Stephanie D. Kovacs, Steven G. Wassilak.In 2023 Winter Simulation Conference (WSC), pp. 1184-1195. IEEE, 2023.
Support for this work comes from a cooperative agreement between Georgia Tech and the CDC (GH22-2272 U2R GH001919).