Biomechanics of Collective Cell Behavior
Modeling complex biological systems that involve a large number of interdependent components seems like a daunting task. Fortunately, the emergence of large-scale behavior in even very complex systems is often dictated by relatively simple local rules. In such cases, computational simulations provide a powerful tool for systematically exploring the mechanisms and interactions that generate the emergent behavior under consideration. We have used this strategy to study the early stages of vasculogenesis in healthy and Cerebral Cavernous Malformation (CCM) tissues.
CCM develops in about 0.5 percent of the population worldwide. It is caused by mutations in one of three genes CCM-1, -2, or -3 that lead to enlarged leaky blood vessels. If this happens in the central nervous system, people experience seizures, paralysis, bleeding in the brain, and loss of hearing or vision. Surgical treatment is often not an option. Therefore there is a need for pharmacological methods to treat this disease.
One particular aspect of the multicellular formation that we investigate is the role of cellular heterogeneity in blood vessel formation. Some endothelial cells seem to behave as anchors that stabilize the initial tube structure with respect to the ECM, whereas others become highly extended reaching out to “glue” parts together. Using experimental and computational techniques we explore whether such heterogeneity results from a memory mechanism, in which past interactions with the mechanical microenvironment lead to changes in transcriptional programs and long-lived cytoskeletal alternations that affect cell fate. The tube formation assay is ideal for studying whether and how the initial environment determines long-term dynamics of phenotypically identical cells and what is the molecular basis for such decision-making.
Cell Morphodynamics
Our lab is particularly interested in close integration of modeling approaches with novel computer vision algorithms, which in our view will greatly enhance the accuracy and predictive power of such models. To this end, we are developing a full-scale cell model that allows coupling of cell edge motion with dynamics of signaling proteins that regulate the cytoskeleton. The key requirements for the model are 1) it should allow realistic cell geometries to be studied; and 2) for a direct comparison with experiments, it must generate output that allows the same type of processing as applied to image data. The mechanical part of the model (cell morphodynamics) is intrinsically stochastic with local protrusions and retractions balancing each other unless internal or external cues provide a directional bias. A chemoattractant gradient is an example of an external cue. The spatiotemporal distribution of signaling proteins (modeled using reaction-diffusion equations) can generate internal cues. As one of the first applications of this model, we will study spatiotemporal correlations between the Rho-family GTPases, their major effectors (such as ROCK, mDia1, LIMK), and the adhesion proteins at the leading edge in a whole new level of detail and complexity.
Patterns of Natural Aging
Cellular senescence, the state in which cells lose their ability to divide and function properly, is closely linked to the aging process. One prominent biomarker of senescence is the protein p16INK4a (p16). Its expression increases with age and is associated with a wide range of age-related diseases. Monitoring p16 levels may offer valuable insights into a person’s health state and the rate of aging. Elevated p16 levels in various tissues have been linked to an increased risk of developing conditions such as cardiovascular disease, diabetes, and certain cancers. In collaboration with Dr. Mitin (Sapere Bio) and Dr. Muss (UNC Lineberger Comprehensive Cancer Center), we study p16 expression dynamics in peripheral blood T cells and its ability to predict functional decline before the onset of age-related diseases. Ultimately, assessing p16 as a biomarker of senescence can provide a potential tool for evaluating an individual’s overall health and their susceptibility to age-related diseases, offering opportunities for personalized healthcare and interventions to promote healthy aging.
Image Analysis
Automated quantification of image data is critically important for several reasons. It decreases personal bias in image interpretation and increases throughput for data processing and analysis, enabling study of highly heterogeneous biological processes. Often, image analysis tools are used to perform systematic and rigorous statistical analysis of results that are already anticipated based on visual inspection. For example, we used such an analysis to characterize phenotypic differences in cells where Src kinase has been rapidly and selectively activated in complex with specific effectors [ref]. However, it is often the case that important biological information is not apparent from visual inspection. For example, in a project on dissecting functional differences between homologous members of Src kinase family [ref], image analysis became the bottleneck of the whole study because of the high cell-to-cell variability in morphodynamics. By developing a novel computational technique for the automated classification of time-dependent changes in cell dynamics (e.g., identifying transitions from polarized spreading to migration) [ref], we were able to establish distinct roles for the various Src kinases in regulating cell migration.
We have developed a computation platform CellGeo to construct a mathematical characterization of the cell edge that allowed the extraction of shape features of any scale in cells with arbitrarily complex geometries [ref]. One powerful feature of this approach is that it allows the simultaneous detection and tracking of thin and broad protrusions in individual cells, allowing the dynamics of both structures to be distinguished and quantified, a task that cannot be accomplished manually. This ability is critical for studying highly dynamic cells with both types of protrusions. For example, CellGeo made it possible to dissect and quantify the behavior of both types of protrusions in epithelial cells located at the leading edge during fly dorsal closure [ref]. In particular, this analysis revealed distinctive roles for the actin associate proteins Diaphanous (Dia) and Enabled (Ena) in regulating both types of protrusions.
CellGeo proved to be a versatile tool to tackle different aspects of cytoskeletal dynamics in 2D. One of the aims in our lab is to extend this computing platform to the analysis of 3D data. This will allow CellGeo to be applied to many exciting physiological problems, including cell and tissue growth and migration in three-dimensional contexts.
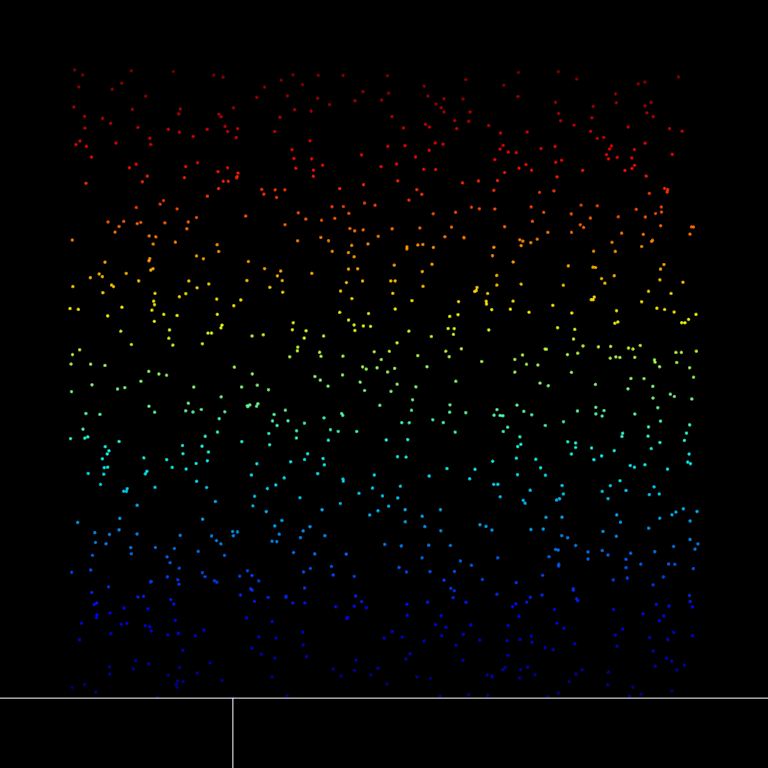