Attacks against Machine Learning
As machine learning based digital twinning efforts become more popular within the nuclear industry, it becomes increasingly necessary to identify potential threats against autonomous control systems and machine learning models. iFAN lab has developed a new attack scenario against autonomous control systems in the nuclear industry by attacking training and testing data being fed into the machine learning models. Adversarial label flip attacks (ALFA) flip a certain percentage of training data to train the machine learning model on false data. Gradient descent attacks flip testing data to trick the data scientist into questioning the validity of the model. Both methods prove a need to protect the training and testing data for autonomous control systems and machine learning-based digital twins within the nuclear industry.
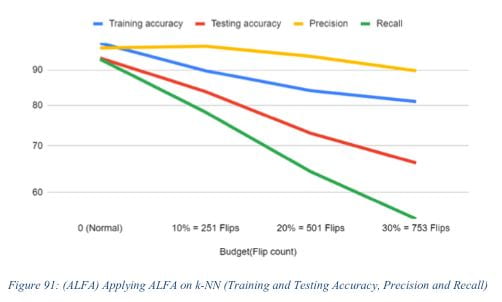
Adversarial Machine Learning
Anticipating new attack vectors is important in understanding potential adversarial actions. To understand more unique and innovative threats, a generative adversarial (GAN) is fed adversarial data to come up with a new plausible attack vector to be tested against the original adversarial model. The ultimate goal is to create a robust digital twin that can overcome new attack vectors by training on GAN-generated data.
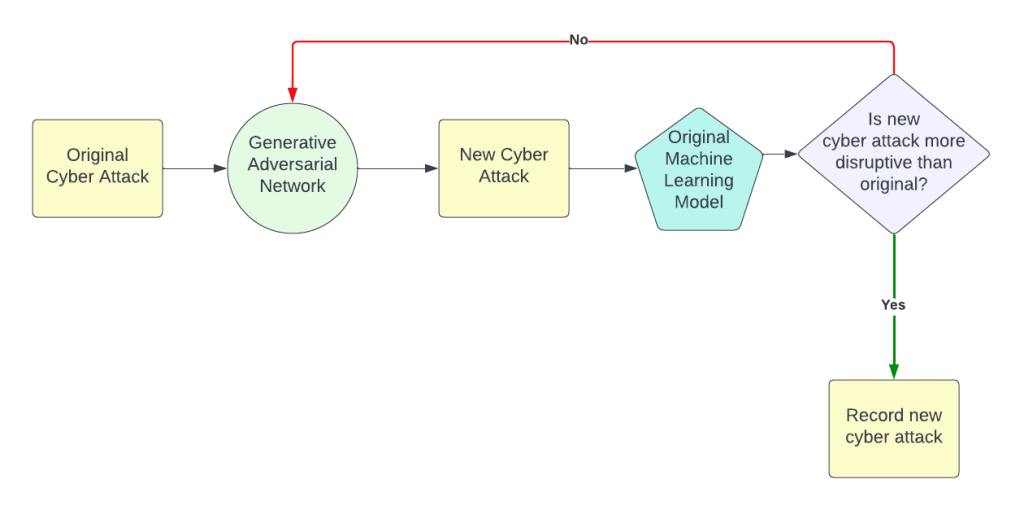