The Next Generation of AI
Computer Vision Advancements at Georgia Tech are Shaping a New Generation of Artificial Intelligence Capabilities
Georgia Tech at CVPR 2023
June 18 – 22 | Vancouver
Computer vision plays a pivotal role in enabling new AI applications by providing machines with the ability to see and interpret visual data, similar to human vision. Research is crucial for advancing computer vision technologies and pushing the boundaries of what is possible.
Discover Georgia Tech’s people and work at CVPR

Georgia Tech at CVPR 2023
Georgia Tech is a leading contributor to CVPR 2023, a research venue focused on computer vision and developing machines that can “see” like humans.
*top 10% of papers
Partner Organizations
Albert Ludwigs Universität Freiburg • Allen Institute for Artificial Intelligence • Amazon • Apple • Argo AI • Boston University • Carnegie Mellon University • Cornell University • Delhi Technological University • ETH Zurich • Florida International University • Fordham University • Google • Huawei Technologies Ltd. • IBM • Imperial College London • Indian Institute of Information Technology • Jabalpur • Massachusetts Institute of Technology • Meta • Michigan State University • MIT-IBM Watson AI Lab • Nanyang Technological University • National Technological University • Near Earth Autonomy Inc. • NVIDIA • Oregon State University • Pennsylvania State University • Princeton University • Rice University • Samsung • Shenzhen • Simon Fraser University • Stanford University • State University of New York at Buffalo • Technische Universität Darmstadt • The Chinese University of Hong Kong • Tongji University • Toyota Research Institute • UC Irvine • Unity Technologies • University of California at Merced • University of Freiburg • University of Illinois • University of Massachusetts at Amherst • University of Michigan-Ann Arbor • University of Pennsylvania • University of Texas • University of Virginia • University of Washington • Vidyasirimedhi Institute of Science and Technology • Whiting School of Engineering
RESEARCH & ACTIVITIES




COMPUTER VISION EXPERTS 
Georgia Tech Researchers Included in the Main Technical Papers Program
On the Horizon
More powerful artificial intelligence (AI) is entering the mainstream on a screen near you. It’s now possible to test drive a handful of services and applications that show this new computing power and automation in high gear.
As this tech spreads across our digital landscape, we asked Georgia Tech experts publishing new research in computer vision — a subfield of AI involving the processing of visual data (e.g. photos and video) — to share their take on the state of AI and what this new horizon of technology looks like.

Faculty Experts on the State of Artificial Intelligence 
→ Hover on images to flip cards
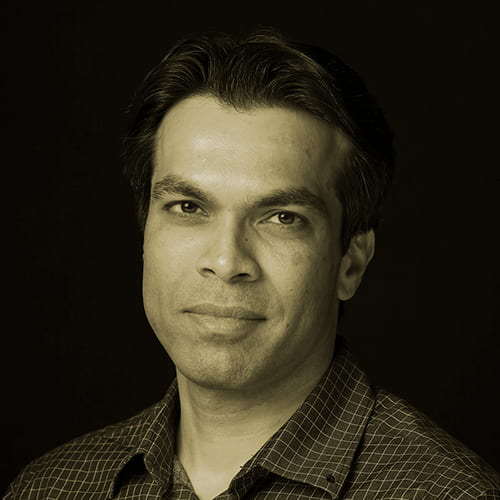
Dhruv Batra
Assoc. Professor, Interactive Computing
Contemporary discussion — and unfortunately, hype — about LLMs and AGI seems oblivious of Moravec’s paradox. We’ve hypothesized since the ’80s that the hardest problems in AI involve sensorimotor control, not abstract thought or reasoning. It explains why AI is mastering games, dialogue, and scene generation. But robots are conspicuously missing from this revolution.
At Georgia Tech, we are pursuing the embodied road to general intelligence – the idea that intelligence emerges in the interaction of an agent with an environment and as a result of sensorimotor activity. We study problems of embodied navigation, mobile manipulation, world modeling, future prediction, social interaction, etc in rich 3D simulators at scale and sim2real transfer to real robots.
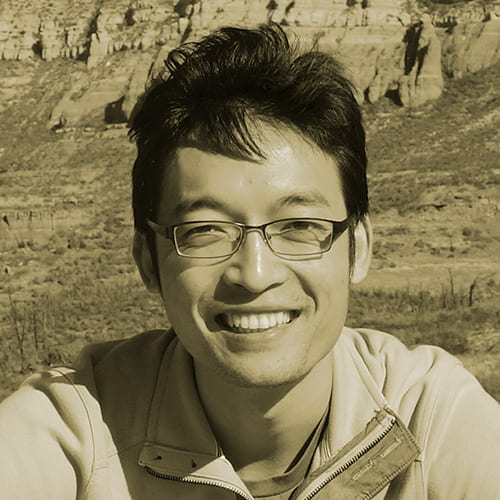
Yongxin Chen
Asst. Professor, Aerospace Engineering
The emergence of generative AI such as text2image and ChatGPT heralds a new era of AI. It not only greatly expands the applications of AI but also enables the public to have access to frontier AI technologies. Its commercial potential will further boost the development of AI.
Our lab focus is on the diffusion model, the backbone of text2image. Our CVPR paper presents a method that can effectively utilize incomplete or fragmented data for training generative models with the aim to relax the conventional dependency on complete datasets for better scalability with regard to training data availability.
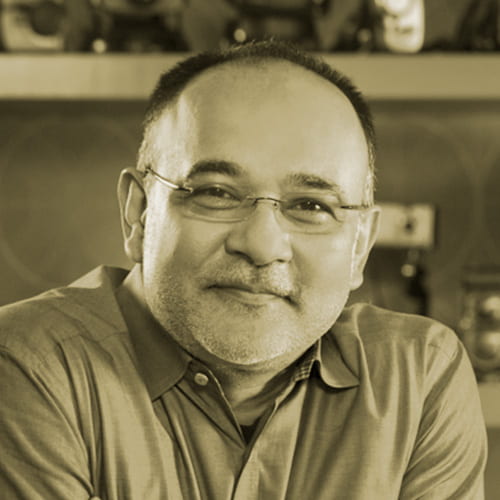
Irfan Essa
Professor, Interactive Computing
Recent developments in artificial intelligence are both a cause for excitement and concern. This rapid growth brings to the fore the importance of responsible deployment of these amazing technologies.
Not only should we ask if and when AI will replace people, but we should also ask when people using AI will replace people not using AI.
AI’s true power in recent times will be how it augments us in our daily lives and how we should responsibly develop these technologies to advance the human condition while being cautious as to how it may negatively impact it.
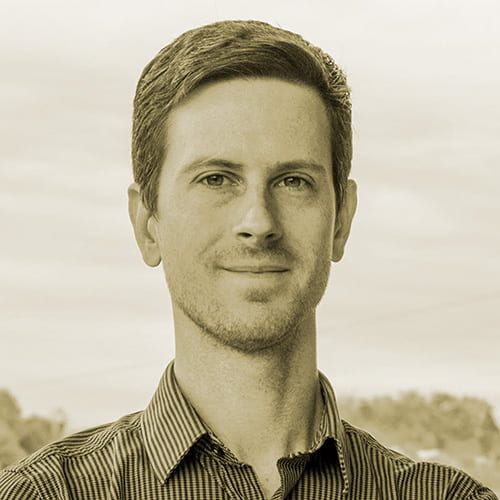
James Hays
Assoc. Professor, Interactive Computing
The long standing computer vision “grand challenge” — to give machines a human-like understanding of images — is nearly solved due to advances in learning, computation, and training data (in increasing order of importance).
Similar advances in other artificial intelligence fields have led to worries that AI is an “existential threat”. There is no reason to worry in the near and medium-term. AI is not embodied, and even if it were it would still need widespread human cooperation to be a threat.
We have passed many AI “singularities” (e.g. machines have been faster at math for a century), and it is exciting to pass a few more this decade, but many more remain!

Judy Hoffman
Asst. Professor, Interactive Computing
It’s truly an amazing time to be working in AI! The world is paying attention and eager to find out how AI will impact their lives. Late breaking research developments are rapidly being integrated into products and propelling new commercial frontiers.
As the reach of this technology further expands in the coming years, our challenge in research will be to not only expand capabilities and make them more accessible, but to advance the reliability and trustworthiness so that AI continues to benefit society.
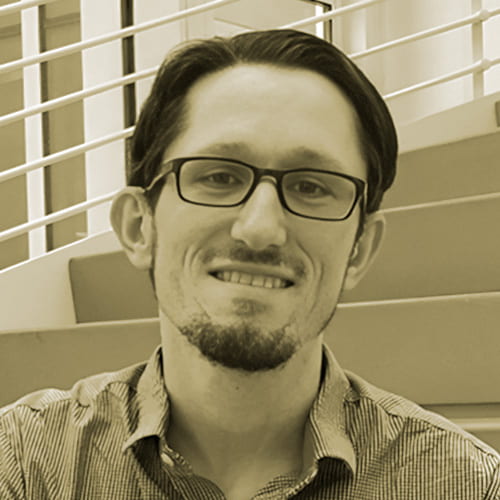
Zsolt Kira
Asst. Professor, Interactive Computing
One of the exciting aspects of current progress in AI is the unification of models across all modalities including language, vision, and audio. As language models become more powerful, I’m excited about the interaction between perception and language, allowing us to ground language models to the real world — preventing these models from hallucinating — and chat with computers about images and videos.
The ability to process all of these types of information jointly and reason about them brings us closer to more general intelligence.
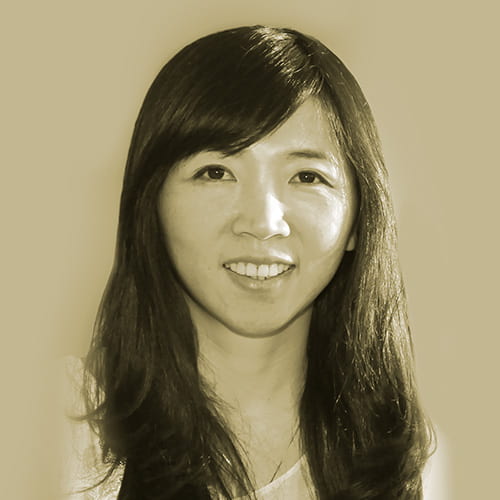
Yingyan (Celine) Lin
Assoc. Professor, Computer Science
AI is transforming numerous sectors of our technology and society, thanks to its amazing breakthroughs, especially in computer vision and natural language processing. While its potential is immense, promising a future of efficient, eco-conscious technology driving societal change, this brings computational and environmental challenges.
Training powerful AI models demands significant resources and emits enormous carbon emissions. Looking forward, balancing computational demand, model performance, and sustainability is crucial for AI advancement and its widespread applications.
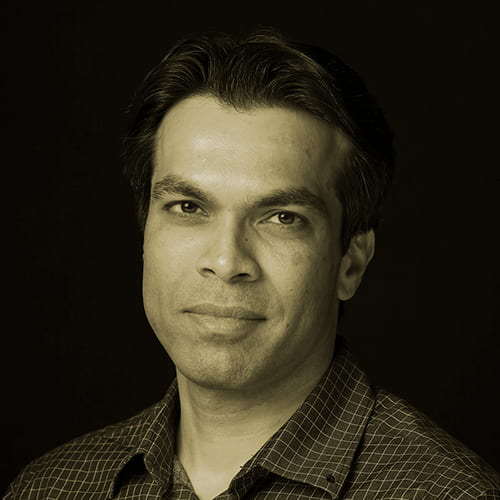
Dhruv Batra
Assoc. Professor, Interactive Computing
Contemporary discussion — and unfortunately, hype — about large language models (LLMs) and artificial general intelligence (AGI) seems oblivious of Moravec’s paradox. We’ve hypothesized since the ’80s that the hardest problems in AI involve sensorimotor control, not abstract thought or reasoning. It explains why AI is mastering games, dialogue, and scene generation. But robots are conspicuously missing from this revolution.
At Georgia Tech, we are pursuing the embodied road to general intelligence – the idea that intelligence emerges in the interaction of an agent with an environment and as a result of sensorimotor activity. We study problems of embodied navigation, mobile manipulation, world modeling, future prediction, social interaction, etc in rich 3D simulators at scale and sim2real transfer to real robots.
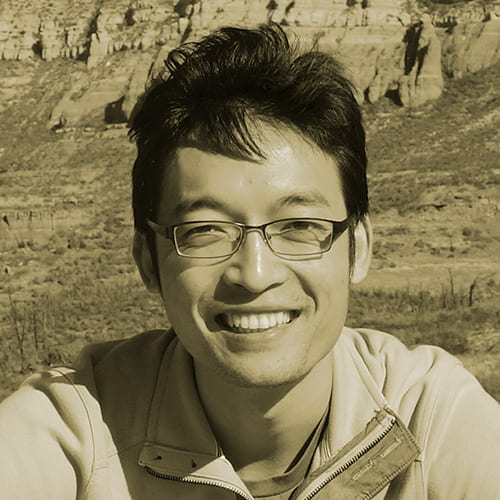
Yongxin Chen
Asst. Professor, Aerospace Engineering
The emergence of generative AI such as text2image and ChatGPT heralds a new era of AI. It not only greatly expands the applications of AI but also enables the public to have access to frontier AI technologies. Its commercial potential will further boost the development of AI.
Our lab focus is on the diffusion model, the backbone of text2image. Our CVPR paper presents a method that can effectively utilize incomplete or fragmented data for training generative models with the aim to relax the conventional dependency on complete datasets for better scalability with regard to training data availability.
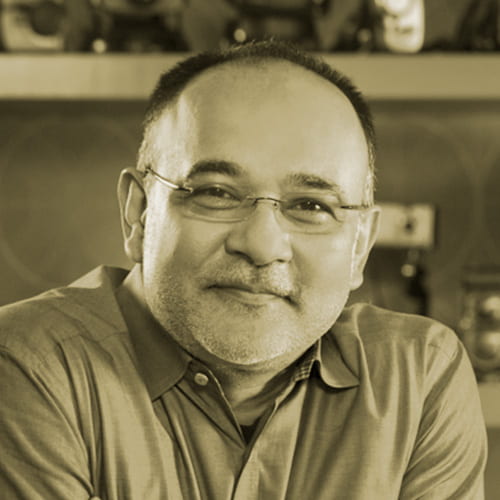
Irfan Essa
Professor, Interactive Computing
Recent developments in artificial intelligence are both a cause for excitement and concern. This rapid growth brings to the fore the importance of responsible deployment of these amazing technologies.
Not only should we ask if and when AI will replace people, but we should also ask when people using AI will replace people not using AI.
AI’s true power in recent times will be how it augments us in our daily lives and how we should responsibly develop these technologies to advance the human condition while being cautious as to how it may negatively impact it.
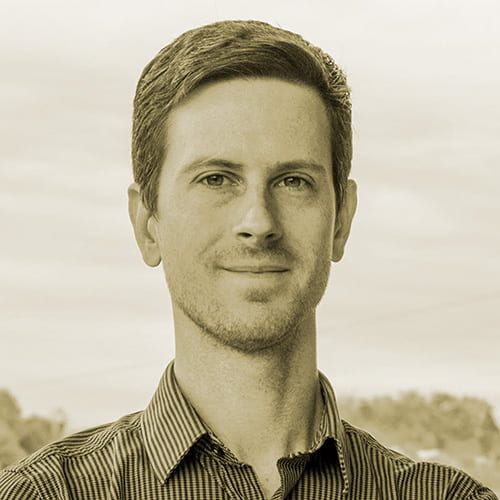
James Hays
Assoc. Professor, Interactive Computing
The long standing computer vision “grand challenge” — to give machines a human-like understanding of images — is nearly solved due to advances in learning, computation, and training data (in increasing order of importance).
Similar advances in other artificial intelligence fields have led to worries that AI is an “existential threat”. There is no reason to worry in the near and medium-term. AI is not embodied, and even if it were it would still need widespread human cooperation to be a threat.
We have passed many AI “singularities” (e.g. machines have been faster at math for a century), and it is exciting to pass a few more this decade, but many more remain!

Judy Hoffman
Asst. Professor, Interactive Computing
It’s truly an amazing time to be working in AI! The world is paying attention and eager to find out how AI will impact their lives. Late breaking research developments are rapidly being integrated into products and propelling new commercial frontiers.
As the reach of this technology further expands in the coming years, our challenge in research will be to not only expand capabilities and make them more accessible, but to advance the reliability and trustworthiness so that AI continues to benefit society.
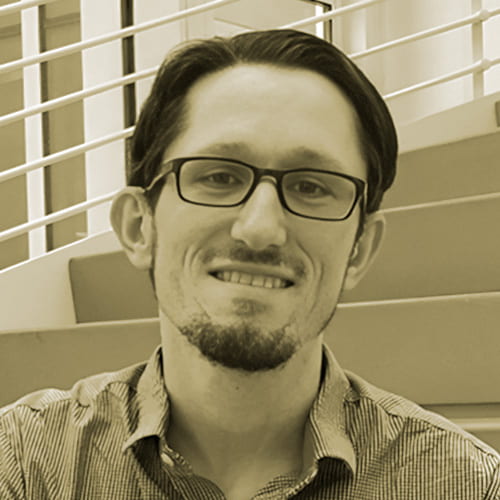
Zsolt Kira
Asst. Professor, Interactive Computing
One of the exciting aspects of current progress in AI is the unification of models across all modalities including language, vision, and audio. As language models become more powerful, I’m excited about the interaction between perception and language, allowing us to ground language models to the real world — preventing these models from hallucinating — and chat with computers about images and videos.
The ability to process all of these types of information jointly and reason about them brings us closer to more general intelligence.
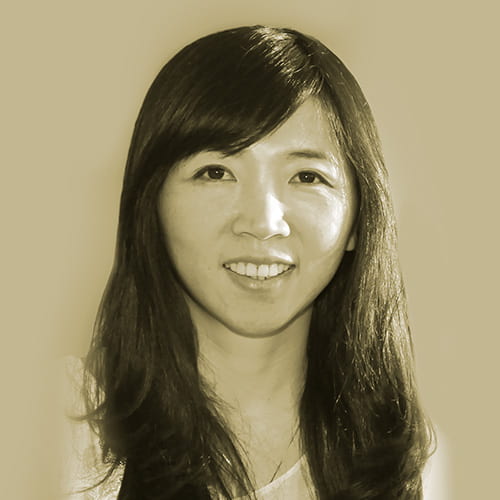
Yingyan (Celine) Lin
Assoc. Professor, Computer Science
AI is transforming numerous sectors of our technology and society, thanks to its amazing breakthroughs, especially in computer vision and natural language processing. While its potential is immense, promising a future of efficient, eco-conscious technology driving societal change, this brings computational and environmental challenges.
Training powerful AI models demands significant resources and emits enormous carbon emissions. Looking forward, balancing computational demand, model performance, and sustainability is crucial for AI advancement and its widespread applications.
Student Researchers working on the AI Frontier
Emerging neural graphic pipelines represent 3D scenes using neural networks. While they can tackle ill-posed reconstruction problems by learning from data, they fall short in rendering speed compared to traditional graphic primitives like mesh, which are more compatible with graphic infrastructures.
Thus, promising advancements can be made in next-generation neural graphics by developing (1) hybrid scene representations that marry neural-based and mesh-based ones to hit a better sweet spot, and (2) tools and libraries to accelerate emerging neural/hybrid representations on commodity hardware.

Yonggan Fu
Ph.D. student in Computer Science
AI invites exciting innovations, especially in multi-modal personal assistants. These systems will adapt to individual needs and contexts, paving the way for personalized technology that understands us deeply. Imagine a world where your devices continually learn from you, leading to unprecedented convenience and quality of life.
However, the intimacy of these AI systems with our lives necessitates robust safeguards for personal information and privacy. AI advancements must go hand-in-hand with stringent data protection measures, ensuring that our smart future is respectful of individual rights.

James Smith
Ph.D. candidate in Machine Learning
Humans empower AIs as intelligent tools to handle various tasks, such as traditional classification, segmentation, and detection. Nowadays, large models of trillion parameters revolutionize the way we interact, making some of the magical tools of science fiction a reality, e.g., voice changers, tracking glasses, personal AR assistants, etc.
Also, those powerful models show a trend to unify multiple tasks into one shared backbone, making it easier to be accelerated by a dedicated chip for mobile computing scenarios like autonomous driving, AR/VR, etc.

Haoran You
Ph.D. student in ML and Computer Architecture
As foundational AI models become more prevalent due to larger model sizes and advanced pretraining techniques, they’re shaping the mainstream of AI-driven applications. With their exceptional ability to generalize and adapt, I foresee a revolution in these applications.
Notably, like humans, AI-driven applications will soon be adaptable to a wide range of tasks with just a few data prompts and minimal resource tuning. Thus, the erstwhile notion of data-intensive and exhaustive tuning in AI could soon be history.

Zhongzhi Yu
Ph.D. student in Computer Science
Faculty Feature 

Assistant Professor Judy Hoffman is at the forefront of training computing systems to ‘see’ the world as people do and adapt in real-time as the situation demands
By Joshua Preston | Photos by Kevin Beasley

Judy Hoffman is planning a large-scale research event that in some ways could be compared to a Broadway musical — it’s a limited production with the promise of high spectacle and will draw a global audience eager to see a show.
The event is this summer’s Computer Vision and Pattern Recognition Conference (CVPR), an international gathering of researchers in computer vision, a subfield of artificial intelligence that, at its simplest, is about training computers to process image and video pixels so they “see” the world as people do.
Over the past 12 months, Hoffman, an assistant professor in the School of Interactive Computing at Georgia Tech, has helped engineer a global production as program co-chair for CVPR. A sampling of her duties includes: coordinating with experts across industries, universities, and national labs; guiding 400+ research area chairs in charge of the peer-review process; and defining the scope and shape of the technical program for the conference. Starting June 18, Hoffman will see the results of the team’s year-long effort when 8,000+ researchers descend on Vancouver.
“It’s been a lot, but well worth it,” says Hoffman. “The research community is producing work that is pretty striking, especially when you start to see the basic research making its way into mainstream applications.”
As a byproduct of her role, Hoffman is helping shape computer vision at a time when the broader field of artificial intelligence is getting more attention. AI captured the public’s imagination late last year with the release of “generative AI” bots for web browsers. A person types a request into the web browser and these bots, such as ChatGPT, can within seconds produce high-fidelity text content (think essays or emails) or photo-realistic art. Seeing the results firsthand is often enough to convince skeptics.
Predictions abound that AI will become a major disruptor, and critical applications, such as self-driving vehicles, will now be closer on the horizon. But for Hoffman and her peers in the field, they understand the level of complexity at play and what must be considered before humanity steps into this science fiction future.

Judy’s Journey →
Research News 

Team First to Successfully Scale Deep Neural Network Models for Federated Learning Framework
By Ben Snedeker
A new machine-learning (ML) framework for clients with varied computing resources is the first of its kind to successfully scale deep neural network (DNN) models like those used to detect and recognize objects in still and video images.
The ability to uniformly scale the width (number of neurons) and depth (number of neural layers) of a DNN model means that remote clients can equitably participate in distributed, real-time training regardless of their computing resources. Resulting benefits include improved accuracy, increased efficiency, and reduced computational costs.
Developed by a team of Georgia Tech researchers led by Ph.D. student Fatih Ilhan, the ScaleFL framework advances federated learning, which is an ML approach inspired by the personal data scandals of the past decade.
CVPR ‘Highlight’ Papers
Highlight Papers — along with Award Papers — recognize notable contributions to CVPR. Highlights represent the top 10 percent (235 papers) of accepted papers; Georgia Tech is included on four Highlight Papers.

Austin Xu
Ph.D. student in Electrical and Computer Engineering
Training Computers with Machine-Generated Examples
Synthetic datasets, which consist of artificially generated data and corresponding annotations, are increasingly used in the development of machine learning models. HandsOff is a GAN-based synthetic-dataset-generating framework capable of producing infinitely many images with corresponding pixel-wise annotations. Unlike existing methods, HandsOff does not require a human in the loop to provide new annotations to train a network to synthesize labels. Instead, HandsOff is trained on a small number (< 50) of real images with existing labels by unifying the fields of GAN inversion and synthetic dataset generation.
By training on real labeled images, HandsOff unlocks several capabilities not found in existing methods, such as the ability to synthesize continuous valued labels and the ability for the practitioner to control the composition of the training set. This latter allows us to tackle the challenging long-tail problem for semantic segmentation. More information about the project can be found at austinxu87.github.io/handsoff.
“Labeled training data underpins much of the success of contemporary machine learning. HandsOff harnesses the expressive power of generative models to unlock an infinite amount of such labeled training examples, leading to more generalizable and accurate model development.” -Austin Xu
WHY IT MATTERS
- HandsOff is trained on real labeled images which leads to higher quality labels when compared to being trained by synthetic labeled images.
- The practitioner has full control over the composition of the HandsOff training set, which is key to addressing the long-tail phenomena.
- The ability to synthesize continuous valued labels is unlocked by avoiding a human-in-the-loop training procedure.
- Start-up costs and infrastructure associated with collecting labels are eliminated: HandsOff can seamlessly be applied in settings where labeled images already exist.

RESEARCH TEAM: Austin Xu, Mariya Vasileva, Achal Dave, and Arjun Seshadri
The Big Picture
The Machine Learning Center at Georgia Tech has developed an interactive visual analysis of the entire technical papers program in cooperation with the CVPR organizing committee. Explore all the papers by institution and main topic.

See you in Vancouver!
Project Lead and Web Development: Joshua Preston
Writers: Joshua Preston, Albert Snedeker
Photography and Visual Media: Kevin Beasley, Joshua Preston
Special Thanks to: Michael Brown, Andreas Geiger, Eric Mortensen, David Hafner, Lee Campbell