Annotating and labeling datasets for machine learning problems is an expensive and time-consuming process for computer vision and natural language scientists. However, a new deep learning approach is being used to decode, localize, and reconstruct image and video captions in seconds, making the machine-generated captions more reliable and trustworthy.
To solve this problem, researchers at the Machine Learning Center at Georgia Tech (ML@GT) and Facebook have created the first cyclical algorithm that can be applied to visual captioning models. The model is able to use the three-step processing during training to make the model more visually-grounded without human annotations or introducing additional computations when deployed, saving researchers time and money on their datasets.
The algorithm employs attention mechanisms, an intuitive concept for humans, when looking at a photo or video. This means that it tries to determine what aspects are important in an image and sequentially create a sentence explaining the visual.
This new model helps solve issues with previous attempts where an algorithm would make its decision based on prior linguistic biases instead of what it is actually “seeing.” This would lead to algorithms having what researchers refer to as object hallucinations. Object hallucinations occur when an algorithmic model assumes an object like a table is in a photo because in previous images, someone with a laptop was always sitting at a table. In this instance, the model is unable to understand a situation where a person has a laptop on their lap instead of a table. This new model helps alleviate the object hallucination problem, thus making the model more reliable and trustworthy.
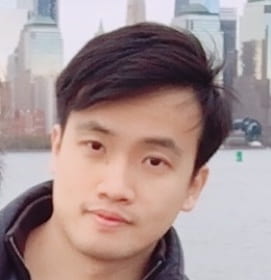
Chih-Yao Ma, a Ph.D. student in the School of Electrical and Computer Engineering, envisions this model being used in situations like describing what happens in the scene as a technology to assist people who are visually impaired to overcome their real daily visual challenges. The model would be a good fit in such instances, because it can alleviate the linguistic bias and object hallucination issues in existing visual captioning models.
This work has been accepted to the European Conference on Computer Vision (ECCV), which takes place virtually August 23-28, 2020.
Paper Information:
Learning to Generate Grounded Visual Captions without Localization Supervision
By Chih-Yao Ma, Yannis Kalantidis, Ghassan AlRegib, Peter Vajda, Marcus Rohrbach, Zsolt Kira
Georgia Tech, NAVER LABS Europe, Facebook
Press Contact:
Allie McFadden
Communications Officer | allie.mcfadden@cc.gatech.edu