ACL 2024
Annual Meeting of the Association for Computational Linguistics | Aug 11 – 16, 2024

The Association for Computational Linguistics (ACL)—a scientific and professional society for those working on computational problems involving human language—convenes an annual gathering with the latest research in the field. Computational linguistics and natural language processing (NLP) explore the development of computational models of various kinds of linguistic phenomena.
Discover Georgia Tech’s experts and their solutions in advancing NLP in the age of large language models and other rapidly evolving technologies.
Natural Language Processing (NLP) research helps computers understand and use human language. This allows AI systems to interact with people more naturally, like answering questions and translating language. Meet the Georgia Tech experts who are charting a path forward. #ACL2024
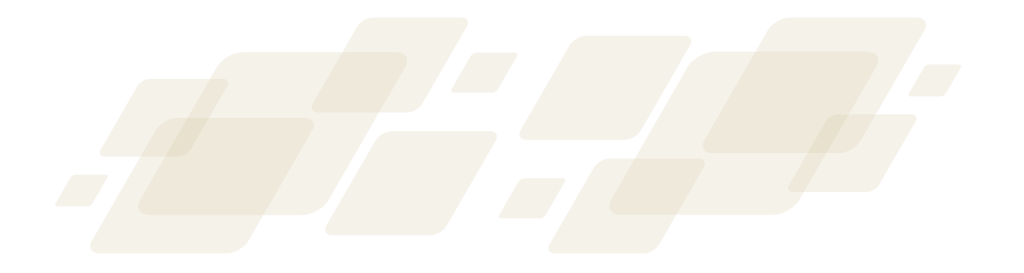
Georgia Tech at ACL 2024
Explore Georgia Tech’s experts and the organizations they are working with at ACL.

By the Numbers*
*Main and Findings Papers
Partner Organizations
Allen Institute for Artificial Intelligence • Amazon • Bloomberg • California Institute of Technology • Carnegie Mellon University • Cisco • Cornell University • Dartmouth College • East China Normal University • Emory University • Georgia Tech • Google • Harvard University • Heinrich-Heine University Düsseldorf • IBM • Inspir.ai • LG Corporation • Massachusetts Institute of Technology • Meta • Microsoft • Monash University • NAVER • Northeastern University • Ohio State University • Phillips-Universität Marburg • Portland State University • Rajiv Gandhi Institute of Technology • Renmin University of China • Seoul National University • Stanford University • The Chinese University of Hong Kong • Toyota Technological Institute at Chicago • Universidad de Vigo • University Hospital Essen • University of Arizona • University of California, Riverside • University of California, San Diego • University of Illinois at Urbana-Champaign • University of Mannheim • University of Texas at Austin • University of Texas at Dallas • University of Texas Southwestern Medical Center • University of Virginia • University of Washington • University of Wisconsin-Madison • Wesleyan University • West Virginia University • Yale University
Faculty with number of papers 
The Big Picture 

Global Program
Explore ACL in one single view. More than 7.4K authors contribute to nearly 2000 papers in the Main and Findings Programs.
Search for your organization in the chart (multiselect from search/drop down menu to capture a complete view of a single organization (e.g. Google, Google Deepmind, Google Research).
Also type in part of the organization name to see 1st authors from the organization.
FEATURE
Limiting Privacy Risks in the Age of AI
By Nathan Deen
A new large-language model (LLM) developed by Georgia Tech researchers detects content that could risk the privacy of social media users and offers alternative phrasing that keeps the context of their posts intact.
Researchers set out to study user awareness of self-disclosure privacy risks on Reddit. It led to users learning just how much personal information they revealed, and that’s when they asked the team to help them strike a balance between sharing and safeguarding.


FEATURE
Limiting Privacy Risks in the Age of AI
A new large-language model (LLM) developed by Georgia Tech researchers detects content that could risk the privacy of social media users and offers alternative phrasing that keeps the context of their posts intact.
Researchers set out to study user awareness of self-disclosure privacy risks on Reddit. It led to users learning just how much personal information they revealed, and that’s when they asked the team to help them strike a balance between sharing and safeguarding.
Pictured: Wei Xu and Alan Ritter, faculty in the School of Interactive Computing
Story by Nathan Deen
Photos by Kevin Beasley

NEWS
Study Highlights Challenges in Detecting Violent Speech Aimed at Asian Communities
By Bryant Wine
A research group is calling for internet and social media moderators to strengthen their detection and intervention protocols for violent speech.
Their study of language detection software found that algorithms struggle to differentiate anti-Asian violence-provoking speech from general hate speech. Left unchecked, threats of violence online can go unnoticed and turn into real-world attacks.
Researchers from Georgia Tech and the Anti-Defamation League (ADL) teamed together in the study. They made their discovery while testing natural language processing (NLP) models trained on data they crowdsourced from Asian communities.
Research Up Close 
Having Beer after Prayer? Measuring Cultural Bias in Large Language Models

Tarek Naous, Ph.D. student in machine learning
Language models (LMs) must be aware of the cultures of the communities they serve, but this is not the case. Our new paper shows that Arabic and Multilingual LMs exhibit bias toward Western culture – even in Arab cultural contexts.

The illustration shows example generated content from GPT-4 and JAIS-Chat—an Arabic-specific LLM—when asked to complete culturally-invoking prompts that are written in Arabic (English translations are shown for info only).
Language models often generate entities that fit in a Western culture (red) instead of the relevant Arab culture.

We find that LMs struggle to adapt to Arab cultural contexts, inappropriately choosing Western entities over relevant Arab ones (such as names, food dishes, locations, etc.) around 45-60% of the time.
Western bias in LMs is manifested in several manners, such as more frequent stereotypical linking of Arab names with poverty and traditionalism and a tendency to attribute negative sentiment to Arab entities.

LMs trained on Wikipedia and web-crawls are the worst at adapting to Arab cultural contexts. The prevalence of Western content in these sources calls for a rethinking of the pre-training data used for developing culturally-aware LMs.
THE METHOD
Our analyses are enabled by CAMeL, our novel resource of culturally-relevant entities and naturally-occurring prompts, which allow measurement of cultural biases in LMs through various setups. CAMeL entities cover both the most frequent and a large number of less-popular entities, enabling robust bias evaluation of LMs.
CAMeL prompts offer contexts grounded in Arab culture as well as neutral contexts, facilitating the evaluation of model cultural adaptation and testing their default cultural preferences.


CAMeL introduces a systematic way to assess LLMs’ favoritism towards Western culture. All LLMs (GPT-4, Aya, mT5, etc.) show favoritism, even when prompts are in non-English and pre-training fully on non-English data.
CAMeL allows:
- An effective way to construct Cultural Bias benchmarks
- 20k+ iconic and long-tail cultural items (food, clothing items, persons, religious sites, etc.) for Arabic vs. Western comparison
- Access LLM favoritism by naturally occurring prompts, story generation, sentiment, NER
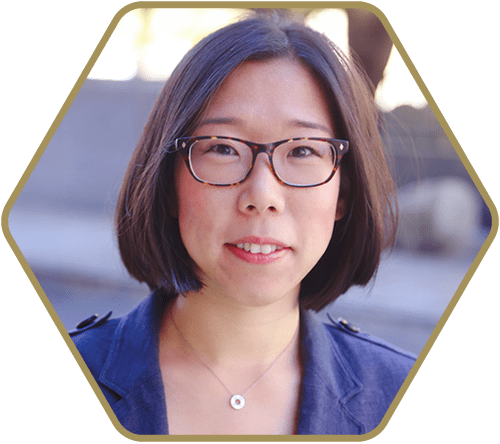
TEAM
- Tarek Naous, Georgia Tech
- Michael J. Ryan, Georgia Tech
- Alan Ritter, Georgia Tech
- Wei Xu, Georgia Tech
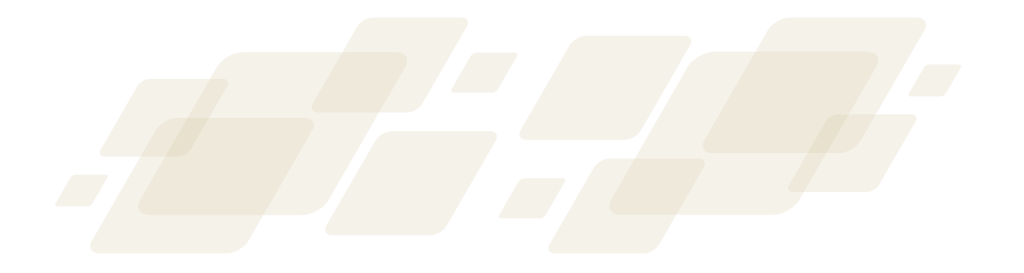

Gaurav Verma, Ph.D. candidate in computer science
One of the most interesting results of the work is that leading language models fail to detect community-identified violence-provoking speech. There are two parts to this:
- Violence-provoking speech, which is essentially speech that could lead to real-world violence;
- The community, identified to be as such in the sense that the data that our insights are based on was crowdsourced from the members of the community.
The emphasis on violence-provoking speech makes our study unique in that it does not treat hateful speech as a monolithic entity. On the other hand, the emphasis on taking a community-centric approach allows us to anchor the training and evaluation of these language models in the lived experiences of the community members.

Srijan Kumar, Asst. Professor, Computational Science and Engineering
Social media platforms often serve as the “ground-zero” where harmful narratives take shape and are amplified, sometimes leading to offline violence.
Our study on characterizing and detecting online violence-provoking speech addresses the need to understand and mitigate the real-world impacts of harmful online behaviors.
Expand
This project, supported by a CDC-funded collaboration with the Anti-Defamation League and Purdue University, emphasizes the importance of anchoring the advances in societal applications of AI in community-centric frameworks.
These frameworks ensure that our research is grounded in the lived experiences of those most affected, thereby enabling more empathetic interventions. We hope our research encourages other works to uncover data-driven insights in a community-centric manner.

Jaiwei Zhou, Ph.D. student in human-centered computing
We believe that we cannot tackle a problem that affects a community without involving the people who are directly impacted. In response, we carefully contextualized our codebook with real anti-Asian violence-provoking speech and involved both experts and insiders.
Expand
One unique aspect of our work lies in our community-centric approach. We recognized the challenge of defining and validating the harm caused by violence-provoking speech, especially when mixed with current and historical prejudices and differing insider-outsider perspectives.
This has been particularly evident in our case, where online harassment has been worsened by long-standing biases and emotional vulnerability during the pandemic, with many sadly translating into offline crimes.
We partnered with a leading non-governmental organization that specializes in countering hate and extremism, and grounded general guidelines on violence-provoking speech in actual instances of such expressions and lived experiences of Asian annotators. By collaborating with both experts and community members, we ensure our research builds on front-line efforts to combat violence-provoking speech while remaining rooted in real experiences and needs of the targeted community.

Rynaa Grover, MS CS student
To address the complexities of this data, we developed an innovative pipeline that deals with the scale of this data in a community-aware manner.
Expand
One of the major challenges in studying violence-provoking content online is effective data collection, as most platforms actively moderate and remove overtly hateful and violent material.
As part of our research, we took up the task of identifying tweets that incite violence against members of the Asian community from a dataset spanning three years.
Our pipeline utilizes a snowballing approach to identify relevant keywords through word co-occurrence and similarity analysis. With support from partners like the Anti-Defamation League (ADL), we also created a comprehensive codebook that breaks down the complex concept of violence-provoking speech into a structured set of criteria.
The methodologies we developed are adaptable to other research involving large-scale textual data and community-centered studies. To encourage further research in this critical area, we have made several key resources—including our codebook, keywords, and data—available on our website: https://claws-lab.github.io/violence-provoking-speech/.

Munmun De Choudhury, Assoc. Professor, Interactive Computing
Hate speech and its impacts are often paradoxical — hate speech may surface in public online platforms, but how victims perceive it can be incredibly personal.
With our work, we hope social media platforms and caregivers can adopt a more trauma-informed, victim-first approach to interventions that protect, support, and empower targeted minoritized communities.
Expand
While most would recognize hate speech detection is a critical component of the healthy functioning of today’s social media sites, few have taken an approach to detect hate speech—especially the kind that may provoke violence—by listening to victims’ unique, subjective lived experiences. This is a salient point as the intent of most violence-provoking speech is to blame, target, “other”, or even threaten specific minoritized groups, and a lack of nuance in detecting such harms can lead to contextually uninformed decisions around how we care for those victimized.
Our work is the first to our knowledge that adopts a community-sensitive, community-aware, and community-centered approach to detecting violence-provoking anti-Asian speech in the context of the COVID-19 pandemic.
RESEARCH 
Main Papers
A Community-Centric Perspective for Characterizing and Detecting Anti-Asian Violence-Provoking Speech
Gaurav Verma; Rynaa Grover; Jiawei Zhou; Binny Mathew; Jordan Kraemer; Munmun De Choudhury; Srijan Kumar
ARL2: Aligning Retrievers with Black-box Large Language Models via Self-guided Adaptive Relevance Labeling
LingXi Zhang; Yue Yu; Kuan Wang; Chao Zhang
Cross-Modal Projection in Multimodal LLMs Doesn’t Really Project Visual Attributes to Textual Space
Gaurav Verma; Minje Choi; Kartik Sharma; Jamelle Watson-Daniels; Sejoon Oh; Srijan Kumar
Explanation-aware Soft Ensemble Empowers Large Language Model In-context Learning
Yue Yu; Jiaming Shen; Tianqi Liu; Zhen Qin; Jing Nathan Yan; Jialu Liu; Chao Zhang; Michael Bendersky
FactPICO: Factuality Evaluation for Plain Language Summarization of Medical Evidence
Sebastian Antony Joseph; Lily Chen; Jan Trienes; Hannah Louisa Göke; Monika Coers; Wei Xu; Byron C Wallace; Junyi Jessy Li
Harnessing the Power of Large Language Models for Natural Language to First-Order Logic Translation
Yuan Yang; Siheng Xiong; Ali Payani; Ehsan Shareghi; Faramarz Fekri
Having Beer after Prayer? Measuring Cultural Bias in Large Language Models
Tarek Naous; Michael J Ryan; Alan Ritter; Wei Xu
InfoLossQA: Characterizing and Recovering Information Loss in Text Simplification
Jan Trienes; Sebastian Antony Joseph; Jörg Schlötterer; Christin Seifert; Kyle Lo; Wei Xu; Byron C Wallace; Junyi Jessy Li
Large Language Models Can Learn Temporal Reasoning
Siheng Xiong; Ali Payani; Ramana Rao Kompella; Faramarz Fekri
Leveraging Codebook Knowledge with NLI and ChatGPT for Zero-Shot Political Relation Classification
Yibo Hu; Erick Skorupa Parolin; Latifur Khan; Patrick Brandt; Javier Osorio; Vito D’Orazio
Machine Unlearning of Pre-trained Large Language Models
Jin Yao; Eli Chien; Minxin Du; Xinyao Niu; Tianhao Wang; Zezhou Cheng; Xiang Yue
MAP’s not dead yet: Uncovering true language model modes by conditioning away degeneracy
Davis Yoshida; Kartik Goyal; Kevin Gimpel
Meta-Tuning LLMs to Leverage Lexical Knowledge for Generalizable Language Style Understanding
Ruohao Guo; Wei Xu; Alan Ritter
Multi-Level Feedback Generation with Large Language Models for Empowering Novice Peer Counselors
Alicja Chaszczewicz; Raj Sanjay Shah; Ryan Louie; Bruce A Arnow; Robert Kraut; Diyi Yang
NEO-BENCH: Evaluating Robustness of Large Language Models with Neologisms
Jonathan Zheng; Alan Ritter; Wei Xu
Predicting Text Preference Via Structured Comparative Reasoning
Jing Nathan Yan; Tianqi Liu; Justin T Chiu; Jiaming Shen; Zhen Qin; Yue Yu; Charumathi Lakshmanan; Yair Kurzion; Alexander M Rush; Jialu Liu; Michael Bendersky
Prototypical Reward Network for Data-Efficient Model Alignment
Jinghan Zhang; Xiting Wang; Yiqiao Jin; Changyu Chen; Xinhao Zhang; Kunpeng Liu
RAM-EHR: Retrieval Augmentation Meets Clinical Predictions on Electronic Health Records
Ran Xu; Wenqi Shi; Yue Yu; Yuchen Zhuang; Bowen Jin; May Dongmei Wang; Joyce C. Ho; Carl Yang
Reducing Privacy Risks in Online Self-Disclosures with Language Models
Yao Dou; Isadora Krsek; Tarek Naous; Anubha Kabra; Sauvik Das; Alan Ritter; Wei Xu
Silent Signals, Loud Impact: LLMs for Word-Sense Disambiguation of Coded Dog Whistles
Julia Kruk; Michela Marchini; Rijul Magu; Caleb Ziems; David Muchlinski; Diyi Yang
Unintended Impacts of LLM Alignment on Global Representation
Michael J Ryan; William Barr Held; Diyi Yang
Who Wrote this Code? Watermarking for Code Generation
Taehyun Lee; Seokhee Hong; Jaewoo Ahn; Ilgee Hong; Hwaran Lee; Sangdoo Yun; Jamin Shin; Gunhee Kim
Findings Papers
A Mechanistic Analysis of a Transformer Trained on a Symbolic Multi-Step Reasoning Task
Jannik Brinkmann; Abhay Sheshadri; Victor Levoso; Paul Swoboda; Christian Bartelt
An Experimental Design Framework for Label-Efficient Supervised Finetuning of Large Language Models
Gantavya Bhatt; Yifang Chen; Arnav Mohanty Das; Jifan Zhang; Sang T. Truong; Stephen Mussmann; Yinglun Zhu; Jeff Bilmes; Simon Shaolei Du; Kevin Jamieson; Jordan T. Ash; Robert D Nowak
Better Late Than Never: Model-Agnostic Hallucination Post-Processing Framework Towards Clinical Text Summarization
Songda Li; Yunqi Zhang; Chunyuan Deng; Yake Niu; Hui Zhao
Everything of Thoughts: Defying the Law of Penrose Triangle for Thought Generation
Ruomeng Ding; Chaoyun Zhang; Lu Wang; Yong Xu; Minghua Ma; Wei Zhang; Si Qin; Saravan Rajmohan; Qingwei Lin; Dongmei Zhang
Knowledge-Infused Prompting: Assessing and Advancing Clinical Text Data Generation with Large Language Models
Ran Xu; Hejie Cui; Yue Yu; Xuan Kan; Wenqi Shi; Yuchen Zhuang; May Dongmei Wang; Wei Jin; Joyce C. Ho; Carl Yang
LSTPrompt: Large Language Models as Zero-Shot Time Series Forecasters by Long-Short-Term Prompting
Haoxin Liu; Zhiyuan Zhao; Jindong Wang; Harshavardhan Kamarthi; B. Aditya Prakash
Measuring and Addressing Indexical Bias in Information Retrieval
Caleb Ziems; William Barr Held; Jane Dwivedi-Yu; Diyi Yang
MM-SOC: Benchmarking Multimodal Large Language Models in Social Media Platforms
Yiqiao Jin; Minje Choi; Gaurav Verma; Jindong Wang; Srijan Kumar
Perceptions of Language Technology Failures from South Asian English Speakers
Faye Holt; William Barr Held; Diyi Yang
PLaD: Preference-based Large Language Model Distillation with Pseudo-Preference Pairs
Rongzhi Zhang; Jiaming Shen; Tianqi Liu; Haorui Wang; Zhen Qin; feng han; Jialu Liu; Simon Baumgartner; Michael Bendersky; Chao Zhang
ProgGen: Generating Named Entity Recognition Datasets Step-by-step with Self-Reflexive Large Language Models
Yuzhao Heng; Chunyuan Deng; Yitong Li; Yue Yu; Yinghao Li; Rongzhi Zhang; Chao Zhang
Self-Specialization: Uncovering Latent Expertise within Large Language Models
Junmo Kang; Hongyin Luo; Yada Zhu; Jacob A Hansen; James R. Glass; David Daniel Cox; Alan Ritter; Rogerio Feris; Leonid Karlinsky
Simulated Misinformation Susceptibility (SMISTS): Enhancing Misinformation Research with Large Language Model Simulations
Weicheng Ma; Chunyuan Deng; Aram Moossavi; Lili Wang; Soroush Vosoughi; Diyi Yang
Token Alignment via Character Matching for Subword Completion
Ben Athiwaratkun; Shiqi Wang; Mingyue Shang; YUCHEN TIAN; Zijian Wang; Sujan Kumar Gonugondla; Sanjay Krishna Gouda; Robert Kwiatkowski; Ramesh Nallapati; Parminder Bhatia; Bing Xiang
Unveiling the Spectrum of Data Contamination in Language Model: A Survey from Detection to Remediation
Chunyuan Deng; Yilun Zhao; Yuzhao Heng; Yitong Li; Jiannan Cao; Xiangru Tang; Arman Cohan
Demo Papers
Wordflow: Social Prompt Engineering for Large Language Models
Zijie (Jay) Wang; Aishwarya Chakravarthy; David Munechika; Polo Chau
Tutorials
Automatic and Human-AI Interactive Text Generation (with a focus on Text Simplification and Revision)
Yao Dou; Philippe Laban; Claire Gardent; Wei Xu
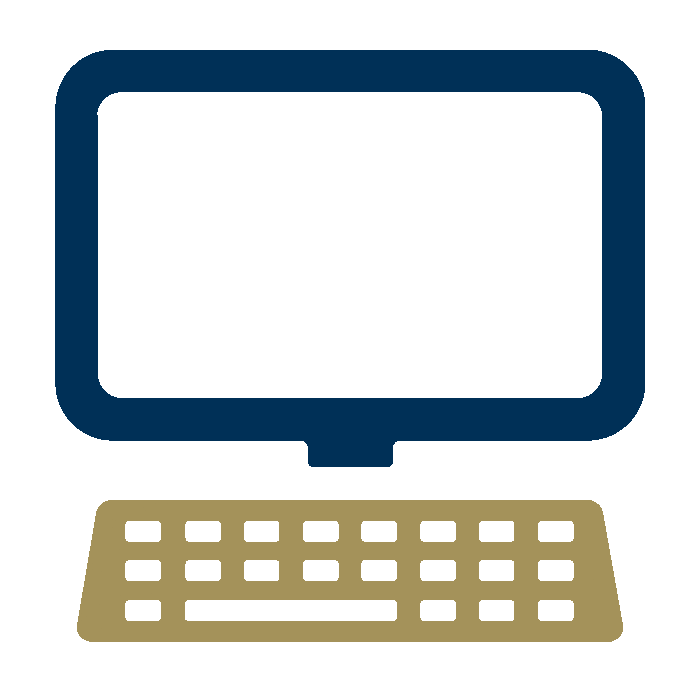
See you in Bangkok!

A Community-Centric Perspective for Characterizing and Detecting Anti-Asian Violence-Provoking Speech
Gaurav Verma, Ph.D. candidate in computer science
Sed ut perspiciatis unde omnis iste natus error sit voluptatem accusantium doloremque laudantium, totam rem Sed ut perspiciatis unde omnis iste natus error sit voluptatem accusantium doloremque laudantium, totam rem Sed ut perspiciatis unde omnis iste natus error sit voluptatem accusantium doloremque laudantium, totam rem
Sed ut perspiciatis unde omnis iste natus error sit voluptatem accusantium doloremque laudantium, totam rem Sed ut perspiciatis unde omnis iste natus error sit voluptatem accusantium doloremque laudantium, totam rem Sed ut perspiciatis unde omnis iste natus error sit voluptatem accusantium doloremque laudantium, totam rem
Expand
Sed ut perspiciatis unde omnis iste natus error sit voluptatem accusantium doloremque laudantium, totam rem aperiam, eaque ipsa quae ab illo inventore veritatis et quasi architecto beatae vitae dicta sunt explicabo. Nemo enim ipsam voluptatem quia voluptas sit aspernatur aut odit aut fugit, sed quia consequuntur magni dolores eos qui ratione voluptatem sequi nesciunt. Neque porro quisquam est, qui dolorem ipsum quia dolor sit amet, consectetur, adipisci velit, sed quia non numquam eius modi tempora incidunt ut labore et dolore magnam aliquam quaerat voluptatem. Ut enim ad minima veniam, quis nostrum exercitationem ullam corporis suscipit laboriosam, nisi ut aliquid ex ea commodi consequatur? Quis autem vel eum iure reprehenderit qui in ea voluptate velit esse quam nihil molestiae consequatur, vel illum qui dolorem eum fugiat quo voluptas nulla pariatur?
Sed ut perspiciatis unde omnis iste natus error sit voluptatem accusantium doloremque laudantium, totam rem aperiam, eaque ipsa quae ab illo inventore veritatis et quasi architecto beatae vitae dicta sunt explicabo. Nemo enim ipsam voluptatem quia voluptas sit aspernatur aut odit aut fugit, sed quia consequuntur magni dolores eos qui ratione voluptatem sequi nesciunt. Neque porro quisquam est, qui dolorem ipsum quia dolor sit amet, consectetur, adipisci velit, sed quia non numquam eius modi tempora incidunt ut labore et dolore magnam aliquam quaerat voluptatem. Ut enim ad minima veniam, quis nostrum exercitationem ullam corporis suscipit laboriosam, nisi ut aliquid ex ea commodi consequatur? Quis autem vel eum iure reprehenderit qui in ea voluptate velit esse quam nihil molestiae consequatur, vel illum qui dolorem eum fugiat quo voluptas nulla pariatur?

Srijan Kumar, Asst. Professor, Computational Science and Engineering
Sed ut perspiciatis unde omnis iste natus error sit voluptatem accusantium doloremque laudantium, totam rem Sed ut perspiciatis unde omnis iste natus error sit voluptatem
Expand
Sed ut perspiciatis unde omnis iste natus error sit voluptatem accusantium doloremque laudantium, totam rem Sed ut perspiciatis unde omnis iste natus error sit voluptatem accusantium doloremque laudantium, totam rem Sed ut perspiciatis unde omnis iste natus error sit voluptatem accusantium doloremque laudantium, totam rem
Sed ut perspiciatis unde omnis iste natus error sit voluptatem accusantium doloremque laudantium, totam rem Sed ut perspiciatis unde omnis iste natus error sit voluptatem accusantium doloremque laudantium, totam rem Sed ut perspiciatis unde omnis iste natus error sit voluptatem accusantium doloremque laudantium, totam rem

Jaiwei Zhou, Ph.D. student in human-centered computing
Sed ut perspiciatis unde omnis iste natus error sit voluptatem accusantium doloremque laudantium, totam rem aperiam, eaque ipsa quae ab illo inventore veritatis et quasi architecto beatae vitae dicta sunt explicabo.
Expand
Sed ut perspiciatis unde omnis iste natus error sit voluptatem accusantium doloremque laudantium, totam rem Sed ut perspiciatis unde omnis iste natus error sit voluptatem accusantium doloremque laudantium, totam rem Sed ut perspiciatis unde omnis iste natus error sit voluptatem accusantium doloremque laudantium, totam rem
Sed ut perspiciatis unde omnis iste natus error sit voluptatem accusantium doloremque laudantium, totam rem Sed ut perspiciatis unde omnis iste natus error sit voluptatem accusantium doloremque laudantium, totam rem Sed ut perspiciatis unde omnis iste natus error sit voluptatem accusantium doloremque laudantium, totam rem

Rynaa Grover, MS CS student
Sed ut perspiciatis unde omnis iste natus error sit voluptatem accusantium doloremque laudantium, totam rem aperiam, eaque ipsa quae ab illo inventore veritatis et quasi architecto beatae vitae dicta sunt explicabo.
Expand
Sed ut perspiciatis unde omnis iste natus error sit voluptatem accusantium doloremque laudantium, totam rem Sed ut perspiciatis unde omnis iste natus error sit voluptatem accusantium doloremque laudantium, totam rem Sed ut perspiciatis unde omnis iste natus error sit voluptatem accusantium doloremque laudantium, totam rem
Sed ut perspiciatis unde omnis iste natus error sit voluptatem accusantium doloremque laudantium, totam rem Sed ut perspiciatis unde omnis iste natus error sit voluptatem accusantium doloremque laudantium, totam rem Sed ut perspiciatis unde omnis iste natus error sit voluptatem accusantium doloremque laudantium, totam rem

Munmun De Choudhury, Assoc. Professor, Interactive Computing
Sed ut perspiciatis unde omnis iste natus error sit voluptatem accusantium doloremque laudantium, totam rem aperiam, eaque ipsa quae ab illo inventore veritatis et quasi architecto beatae vitae dicta sunt explicabo.
Expand
Sed ut perspiciatis unde omnis iste natus error sit voluptatem accusantium doloremque laudantium, totam rem Sed ut perspiciatis unde omnis iste natus error sit voluptatem accusantium doloremque laudantium, totam rem Sed ut perspiciatis unde omnis iste natus error sit voluptatem accusantium doloremque laudantium, totam rem
Sed ut perspiciatis unde omnis iste natus error sit voluptatem accusantium doloremque laudantium, totam rem Sed ut perspiciatis unde omnis iste natus error sit voluptatem accusantium doloremque laudantium, totam rem Sed ut perspiciatis unde omnis iste natus error sit voluptatem accusantium doloremque laudantium, totam rem
Development: College of Computing
Project Lead/Data Graphics: Joshua Preston
News: Nathan Deen, Joshua Preston, Bryant Wine
Select Photos: Kevin Beasley
Data Management: Joni Isbell