CVPR 2024
IEEE / CVF Computer Vision and Pattern Recognition Conference | June 17 – 21, 2024

The IEEE / CVF Computer Vision and Pattern Recognition Conference (CVPR) is the premier annual research event for the field of computer vision, a discipline that enables computers to derive information from images, videos and other inputs. Discover Georgia Tech’s experts and their contributions shaping computer vision in the era of artificial intelligence.
Robotics research is part of the next wave of computing innovation and is key to shaping how artificial intelligence will become part of our lives. Meet the Georgia Tech experts who are charting a path forward. #ICRA2024
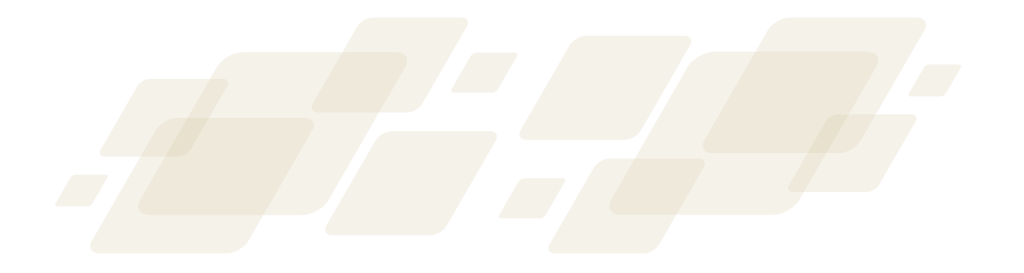
Georgia Tech at CVPR 2024
Explore Georgia Tech’s experts and the organizations they are working with at CVPR.
By the Numbers
Partner Organizations
Adobe • Allen Institute for Artificial Intelligence • Amazon • Beijing University of Aeronautics and Astronautics • Carnegie Mellon University • Georgia Tech • Google • IBM • IIIT-Hyderabad • Indiana University • King Abdullah University of Science and Technology (KAUST) • Koc University • Lepton AI Inc. • Massachusetts Institute of Technology • Meta • Microsoft Research • Mohamed bin Zayed University of Artificial Intelligence • National University of Singapore • NVIDIA • Picsart AI Research • Princeton University • Qualcomm Inc • Reblink • Simon Fraser University • Snap Inc • Stanford University • State University of New York – Binghamton • State University of New York – Buffalo • Tel Aviv University • Terminus Group • Torc Robotics • Tsinghua University • Universidad de Los Andes • University of Bristol • University of British Columbia • University of California – Berkeley • University of California – Los Angeles • University of Catania • University of Illinois – Urbana-Champaign • University of Louisiana – Lafayette • University of Maryland – College Park • University of Minnesota • University of North Carolina – Chapel Hill • University of Oxford • University of Pennsylvania • University of Science and Technology of China • University of Texas – Austin • University of Tokyo • University of Trento • Virginia Polytechnic Institute and State University • Zhejiang University
Topics with Georgia Tech
- 3D from multi-view and sensors
- 3D from single images
- Datasets and evaluation
- Efficient and scalable vision
- Embodied vision: Active agents, simulation
- Humans: Face, body, pose, gesture, movement
- Image and video synthesis and generation
- Multi-modal learning
- Recognition: Categorization, detection, retrieval
- Robotics
- Scene analysis and understanding
- Segmentation, grouping and shape analysis
- Transfer, meta, low-shot, continual, or long-tail learning
- Transparency, fairness, accountability, privacy, ethics in vision
- Vision applications and systems
- Vision, language, and reasoning
Faculty
The Big Picture

Georgia Tech Teams
Georgia Tech’s 29 papers in the technical program include two that are Top 5% papers (oral) and four that are Top 10% papers (highlight). Female faculty members are the majority of institute faculty on these top papers, with two women—Judy Hoffman and Devi Parikh—included. Humphrey Shi also co-authors a top paper, and has the most acceptances overall with seven papers. These three faculty are from the School of Interactive Computing.

Search for people and topics in the chart. The first column shows Georgia Tech-led teams. Each row is an entire team. Explore more now.
Global Program
Explore the entire CVPR 2024 program by primary paper topic and the accompanying tiers for papers — Top 5% Papers (ORAL), Top 10% Papers (HIGHLIGHT), and Poster Papers.
Hover on chart elements to highlight topics of interest. Use the dropdown menu to filter the conference program by organization and see their contributions. Toggle the tab for Paper Listing in the top left corner for paper details. Check out the Georgia Tech work here!


Episode of ‘Friends’ Inspires New Tool that Provides Human-like Perception to MLLMs 🔗
By Nathan Deen

For Jitesh Jain, conducting a simple experiment while watching one of his favorite TV series became the genesis of a paper accepted into CVPR 2024.
Jain is the creator of VCoder, a new tool that enhances the visual perception capabilities of multimodal large language models (MLLMs). Jain said MLLMs like GPT-4 with vision (GPT-4V) are prone to miss obscure objects that blend in with other objects in an image.
Jain paused his TV as he watched The One with the Halloween Party episode of the popular TV Series Friends.
Chandler stood out the most in a pink bunny costume while holding hands with Ross in a potato costume. As the two prepared for an arm-wrestling match with Joey and Phoebe, multiple groups socialized behind them.
Jain wondered how accurate GPT-4V would be if he prompted it to describe everything happening in this image.
Research
3D from multi-view and sensors
Ziyi Chen, Xiaolong Wu, Yu Zhang
State-of-the-art neural implicit surface representations have achieved impressive results in indoor scene reconstruction by incorporating monocular geometric priors as additional supervision. However, we have observed that multi-view inconsistency between such priors poses a challenge for high-quality reconstructions. In response, we present NC-SDF, a neural signed distance field (SDF) 3D reconstruction framework with view-dependent normal compensation (NC). Specifically, we integrate view-dependent biases in monocular normal priors into the neural implicit representation of the scene. By adaptively learning and correcting the biases, our NC-SDF effectively mitigates the adverse impact of inconsistent supervision, enhancing both the global consistency and local details in the reconstructions. To further refine the details, we introduce an informative pixel sampling strategy to pay more attention to intricate geometry with higher information content. Additionally, we design a hybrid geometry modeling approach to improve the neural implicit representation. Experiments on synthetic and real-world datasets demonstrate that NC-SDF outperforms existing approaches in terms of reconstruction quality.
3D from single images
ZeroShape: Regression-based Zero-shot Shape Reconstruction
Zixuan Huang, Stefan Stojanov, Anh Thai, Varun Jampani, James Rehg
We study the problem of single-image zero-shot 3D shape reconstruction. Recent works learn zero-shot shape reconstruction through generative modeling of 3D assets, but these models are computationally expensive at train and inference time. In contrast, the traditional approach to this problem is regression-based, where deterministic models are trained to directly regress the object shape. Such regression methods possess much higher computational efficiency than generative methods. This raises a natural question: is generative modeling necessary for high performance, or conversely, are regression-based approaches still competitive? To answer this, we design a strong regression-based model, called ZeroShape, based on the converging findings in this field and a novel insight. We also curate a large real-world evaluation benchmark, with objects from three different real-world 3D datasets. This evaluation benchmark is more diverse and an order of magnitude larger than what prior works use to quantitatively evaluate their models, aiming at reducing the evaluation variance in our field. We show that ZeroShape not only achieves superior performance over state-of-the-art methods, but also demonstrates significantly higher computational and data efficiency.
Datasets and evaluation
Ego-Exo4D: Understanding Skilled Human Activity from First- and Third-Person Perspectives
Kristen Grauman, Andrew Westbury, Lorenzo Torresani, Kris Kitani, Jitendra Malik, Triantafyllos Afouras, Kumar Ashutosh, Vijay Baiyya, Siddhant Bansal, Bikram Boote, Eugene Byrne, Zachary Chavis, Joya Chen, Feng Cheng, Fu-Jen Chu, Sean Crane, Avijit Dasgupta, Jing Dong, Maria Escobar, Cristhian David Forigua Diaz, Abrham Gebreselasie, Sanjay Haresh, Jing Huang, Md Mohaiminul Islam, Suyog Jain, Rawal Khirodkar, Devansh Kukreja, Kevin Liang, Jia-Wei Liu, Sagnik Majumder, Yongsen Mao, Miguel Martin, Effrosyni Mavroudi, Tushar Nagarajan, Francesco Ragusa, Santhosh Kumar Ramakrishnan, Luigi Seminara, Arjun Somayazulu, Yale Song, Shan Su, Zihui Xue, Edward Zhang, Jinxu Zhang, Angela Castillo, Changan Chen, Fu Xinzhu, Ryosuke Furuta, Cristina González, Gupta, Jiabo Hu, Yifei Huang, Yiming Huang, Weslie Khoo, Anush Kumar, Robert Kuo, Sach Lakhavani, Miao Liu, Mi Luo, Zhengyi Luo, Brighid Meredith, Austin Miller, Oluwatumininu Oguntola, Xiaqing Pan, Penny Peng, Shraman Pramanick, Merey Ramazanova, Fiona Ryan, Wei Shan, Kiran Somasundaram, Chenan Song, Audrey Southerland, Masatoshi Tateno, Huiyu Wang, Yuchen Wang, Takuma Yagi, Mingfei Yan, Xitong Yang, Zecheng Yu, Shengxin Zha, Chen Zhao, Ziwei Zhao, Zhifan Zhu, Jeff Zhuo, Pablo ARBELAEZ, Gedas Bertasius, Dima Damen, Jakob Engel, Giovanni Maria Farinella, Antonino Furnari, Bernard Ghanem, Judy Hoffman, C.V. Jawahar, Richard Newcombe, Hyun Soo Park, James Rehg, Yoichi Sato, Manolis Savva, Jianbo Shi, Mike Zheng Shou, Michael Wray
We present Ego-Exo4D, a diverse, large-scale multimodal multiview video dataset and benchmark challenge. Ego-Exo4D centers around simultaneously-captured egocentric and exocentric video of skilled human activities (e.g., sports, music, dance, bike repair). More than 800 participants from 13 cities worldwide performed these activities in 131 different natural scene contexts, yielding long-form captures from 1 to 42 minutes each and 1,422 hours of video combined. The multimodal nature of the dataset is unprecedented: the video is accompanied by multichannel audio, eye gaze, 3D point clouds, camera poses, IMU, and multiple paired language descriptions—including a novel “expert commentary” done by coaches and teachers and tailored to the skilled-activity domain. To push the frontier of first-person video understanding of skilled human activity, we also present a suite of benchmark tasks and their annotations, including fine-grained activity understanding, proficiency estimation, cross-view translation, and 3D hand/body pose. All resources will be open sourced to fuel new research in the community.
TRINS: Towards Multimodal Language Models That Can Read
Ruiyi Zhang, Yanzhe Zhang, Jian Chen, Yufan Zhou, Jiuxiang Gu, Changyou Chen, Tong Sun
Large multimodal language models have shown remarkable proficiency in understanding and editing images. However, a majority of these visually-tuned models struggle to comprehend the textual content embedded in images, primarily due to the limitation of training data. In this work, we introduce TRINS: a Text-Rich image\footnote{In this work, we use the phrase “text-rich images” to describe images with rich textual information, such as posters and book covers.} INStruction dataset, with the objective of enhancing the reading ability of the multimodal large language model. TRINS is built using hybrid data annotation strategies including machine-assisted and human-assisted annotation process. It contains 39,153 text-rich images, captions and 102,437 questions. Specifically, we show that the number of words per annotation in TRINS is significantly longer than that of related datasets, providing new challenges. Furthermore, we introduce a simple and effective architecture, called Language-vision Reading Assistant (LaRA), that is good at understanding textual contents within images. LaRA outperforms existing state-of-the-art multimodal large language models on the TRINS dataset as well as other classical benchmarks. Lastly, we conducted a comprehensive evaluation with TRINS on various text-rich image understanding and generation tasks, demonstrating its effectiveness.
Efficient and scalable vision
Resource-Efficient Transformer Pruning for Finetuning of Large Models
Fatih Ilhan, Gong Su, Selim Tekin, Tiansheng Huang, Sihao Hu, Ling Liu
With the recent advances in vision transformers and large language models (LLMs), finetuning costly large models on downstream learning tasks poses significant challenges under limited computational resources. This paper presents a REsource and ComputAtion-efficient Pruning framework (RECAP) for the finetuning of transformer-based large models. RECAP by design bridges the gap between efficiency and performance through an iterative process cycling between pruning, finetuning, and updating stages to explore different chunks of the given large-scale model. At each iteration, we first prune the model with Taylor-approximation-based importance estimation and then only update a subset of the pruned model weights based on the Fisher-information criterion. In this way, RECAP achieves two synergistic and yet conflicting goals: reducing the GPU memory footprint while maintaining model performance, unlike most existing pruning methods that require the model to be finetuned beforehand for better preservation of model performance. We perform extensive experiments with a wide range of large transformer-based architectures on various computer vision and natural language understanding tasks. Compared to recent pruning techniques, we demonstrate that RECAP offers significant improvements in GPU memory efficiency, capable of reducing the footprint by up to 65\%.
Embodied vision: Active agents, simulation
GOAT-Bench: A Benchmark for Multi-modal Lifelong Navigation
Mukul Khanna, Ram Ramrakhya, Gunjan Chhablani, Sriram Yenamandra, Theo Gervet, Matthew Chang, Zsolt Kira, Devendra Singh Chaplot, Dhruv Batra, Roozbeh Mottaghi
The Embodied AI community has recently made significant strides in visual navigation tasks, exploring targets from 3D coordinates, objects, language description, and images. However, these navigation models often handle only a single input modality as the target. With the progress achieved so far, it is time to move towards universal navigation models capable of handling various goal types, enabling more effective user interaction with robots. To facilitate this goal, we propose GOAT-Bench, a benchmark for the universal navigation task referred to as GO to AnyThing (GOAT). In this task, the agent is directed to navigate to a sequence of targets specified by the category name, language description, or instance image in an open-vocabulary fashion. We benchmark monolithic RL and modular methods on the GOAT task, analyzing their performance across modalities, the role of explicit and implicit scene memories, their robustness to noise in goal specifications, and the impact of memory in lifelong scenarios.
Mukul Khanna, Yongsen Mao, Hanxiao Jiang, Sanjay Haresh, Brennan Shacklett, Dhruv Batra, Alexander William Clegg, Eric Undersander, Angel Xuan Chang, Manolis Savva
We contribute the Habitat Synthetic Scene Dataset, a dataset of 211 high-quality 3D scenes, and use it to test navigation agent generalization to realistic 3D environments. Our dataset represents real interiors and contains a diverse set of 18,656 models of real-world objects. We investigate the impact of synthetic 3D scene dataset scale and realism on the task of training embodied agents to find and navigate to objects (ObjectGoal navigation). By comparing to synthetic 3D scene datasets from prior work, we find that scale helps in generalization, but the benefits quickly saturate, making visual fidelity and correlation to real-world scenes more important. Our experiments show that agents trained on our smaller-scale dataset can match or outperform agents trained on much larger datasets. Surprisingly, we observe that agents trained on just 122 scenes from our dataset outperform agents trained on 10,000 scenes from the ProcTHOR-10K dataset in terms of zero-shot generalization in real-world scanned environments.
OpenEQA: Embodied Question Answering in the Era of Foundation Models
Arjun Majumdar, Anurag Ajay, Xiaohan Zhang, Sriram Yenamandra, Mikael Henaff, Alexander Sax, Sneha Silwal, Paul McVay, Oleksandr Maksymets, Sergio Arnaud, Pranav Putta, Karmesh Yadav, Qiyang Li, Benjamin Newman, Mohit Sharma, Vincent-Pierre Berges, Shiqi Zhang, Pulkit Agrawal, Dhruv Batra, Yonatan Bisk, Mrinal Kalakrishnan, Franziska Meier, Chris Paxton, Aravind Rajeswaran
We present a modern formulation of Embodied Question Answering (EQA) as the task of understanding an environment well enough to answer questions about it in natural language. An agent can achieve such an understanding by either drawing upon episodic memory, exemplified by agents on smart glasses, or by actively exploring the environment, as in the case of mobile robots. We accompany our formulation with OpenEQA — the first open-vocabulary benchmark dataset for EQA supporting both episodic memory and active exploration use cases. OpenEQA contains over 1600 high-quality human generated questions drawn from over 180 real-world environments. In addition to the dataset, we also provide an automatic LLM-powered evaluation protocol that has excellent correlation with human judgement. Using this dataset and evaluation protocol, we evaluate several state-of-the-art foundation models including GPT-4V, and find that they significantly lag behind human-level performance. Consequently, OpenEQA stands out as a straightforward, measurable, and practically relevant benchmark that poses a considerable challenge to current generation of foundation models. We hope this inspires and stimulates future research at the intersection of Embodied AI, conversational agents, and world models.
Seeing the Unseen: Visual Common Sense for Semantic Placement
Ram Ramrakhya, Aniruddha Kembhavi, Dhruv Batra, Zsolt Kira, Kuo-Hao Zeng, Luca Weihs
Computer vision tasks typically involve describing what is visible in an image (e.g. classification, detection, segmentation, and captioning). We study a visual common sense task that requires understanding ‘what is not visible’. Specifically, given an image (e.g. of a living room) and a name of an object (“cushion”), a vision system is asked to predict semantically-meaningful regions (masks or bounding boxes) in the image where that object could be placed or is likely be placed by humans (e.g. on the sofa). We call this task: Semantic Placement (SP) and believe that such common-sense visual understanding is critical for assitive robots (tidying a house), AR devices (automatically rendering an object in the user’s space), and visually-grounded chatbots with common sense. Studying the invisible is hard. Datasets for image description are typically constructed by curating relevant images (e.g. via image search with object names) and asking humans to annotate the contents of the image; neither of those two steps are straightforward for objects not present in the image. We overcome this challenge by operating in the opposite direction: we start with an image of an object in context (which is easy to find online) and remove that object from the image via inpainting. This automated pipeline converts unstructured web data into a paired with/without object dataset. With this proposed data generation pipeline, we collect a novel dataset, containing ~1.3M images across 9 object categories. We then train a SP prediction model, called CLIP-UNet, on our dataset. The CLIP-UNet outperforms existing VLMs and baselines that combine semantic priors with object detectors, generalizes well to real-world and simulated images, exhibits semantics-aware reasoning for object placement, and enables downstream applications like tidying robots in indoor environments.
Humans: Face, body, pose, gesture, movement
AAMDM: Accelerated Auto-regressive Motion Diffusion Model
Tianyu Li, Calvin Zhuhan Qiao, Ren Guanqiao, KangKang Yin, Sehoon Ha
Interactive motion synthesis is essential in creating immersive experiences in entertainment applications, such as video games and virtual reality. However, generating animations that are both high-quality and contextually responsive remains a challenge. Traditional techniques in the game industry can produce high-fidelity animations but suffer from high computational costs and poor scalability. Trained neural network models alleviate the memory and speed issues, yet fall short on generating diverse motions. Diffusion models offer diverse motion synthesis with low memory usage, but require expensive reverse diffusion processes. This paper introduces the Accelerated Auto-regressive Motion Diffusion Model (AAMDM), a novel motion synthesis framework designed to achieve quality, diversity, and efficiency all together. AAMDM integrates Denoising Diffusion GANs as a fast Generation Module, and an Auto-regressive Diffusion Model as a Polishing Module. Furthermore, AAMDM operates in a lower-dimensional embedded space rather than the full-dimensional pose space, which reduces the training complexity as well as further improves the performance. We show that AAMDM outperforms existing methods in motion quality, diversity, and runtime efficiency, through comprehensive quantitative analyses and visual comparisons. We also demonstrate the effectiveness of each algorithmic component through ablation studies.
Image and video synthesis and generation
DistriFusion: Distributed Parallel Inference for High-Resolution Diffusion Models
Muyang Li, Tianle Cai, Jiaxin Cao, Qinsheng Zhang, Han Cai, Junjie Bai, Yangqing Jia, Kai Li, Song Han
Diffusion models have achieved great success in synthesizing high-quality images. However, generating high-resolution images with diffusion models is still challenging due to the enormous computational costs, resulting in a prohibitive latency for interactive applications. In this paper, we propose DistriFusion to tackle this problem by leveraging parallelism across multiple GPUs. Our method splits the model input into multiple patches and assigns each patch to a GPU. However, naively implementing such an algorithm breaks the interaction between patches and loses fidelity, while incorporating such an interaction will incur tremendous communication overhead. To overcome this dilemma, we observe the high similarity between the input from adjacent diffusion steps and propose Displaced Patch Parallelism, which takes advantage of the sequential nature of the diffusion process by reusing the pre-computed feature maps from the previous timestep to provide context for the current step. Therefore, our method supports asynchronous communication, which can be pipelined by computation. Extensive experiments show that our method can be applied to recent Stable Diffusion XL with no quality degradation and achieve up to a 6.1$\times$ speedup on eight NVIDIA A100s compared to one. Our code is publicly available at https://github.com/mit-han-lab/distrifuser.
Precise Image Editing via Recognition and Generation Tasks
Shelly Sheynin, Adam Polyak, Uriel Singer, Yuval Kirstain, Amit Zohar, Oron Ashual, Devi Parikh, Yaniv Taigman
Instruction-based image editing holds immense potential for a variety of applications, as it enables users to perform any editing operation using a natural language instruction. However, current models in this domain often struggle with accurately executing user instructions. We present IEdit, a multi-task image editing model which sets state-of-the-art results in instruction-based image editing. To develop IEdit we train it to multi-task across an unprecedented range of tasks, such as region-based editing, free-form editing, and Computer Vision tasks, all of which are formulated as generative tasks. Additionally, to enhance IEdit’s multi-task learning abilities, we provide it with learned task embeddings which guide the generation process towards the correct edit type. Both these elements are essential for IEdit’s outstanding performance. Furthermore, we show that IEdit can generalize to new tasks, such as image inpainting, super-resolution, and compositions of editing tasks, with just a few labeled examples. This capability offers a significant advantage in scenarios where high-quality samples are scarce. Lastly, to facilitate a more rigorous and informed assessment of instructable image editing models, we release a new challenging and versatile benchmark that includes seven different image editing tasks.
RAVE: Randomized Noise Shuffling for Fast and Consistent Video Editing with Diffusion Models
Ozgur Kara, Bariscan Kurtkaya, Hidir Yesiltepe, James Rehg, Pinar Yanardag
Recent advancements in diffusion-based models have demonstrated significant success in generating images from text. However, video editing models have not yet reached the same level of visual quality and user control. To address this, we introduce RAVE, a zero-shot video editing method that leverages pre-trained text-to-image diffusion models without additional training. RAVE takes an input video and a text prompt to produce high-quality videos while preserving the original motion and semantic structure. It employs a novel noise shuffling strategy, leveraging spatio-temporal interactions between frames, to produce temporally consistent videos faster than existing methods. It is also efficient in terms of memory requirements, allowing it to handle longer videos. RAVE is capable of a wide range of edits, from local attribute modifications to shape transformations. In order to demonstrate the versatility of RAVE, we create a comprehensive video evaluation dataset ranging from object-focused scenes to complex human activities like dancing and typing, and dynamic scenes featuring swimming fish and boats. Our qualitative and quantitative experiments highlight the effectiveness of RAVE in diverse video editing scenarios compared to existing methods. Our code, dataset and videos can be found in our supplementary materials.
Condition-Aware Neural Network for Controlled Image Generation
Han Cai, Muyang Li, Qinsheng Zhang, Ming-Yu Liu, Song Han
We present Condition-Aware Neural Network (CAN), a new method for adding control to image generative models. In parallel to prior conditional control methods, CAN controls the image generation process by dynamically manipulating the weight of the neural network. This is achieved by introducing a condition-aware weight generation module that generates conditional weight for convolution/linear layers based on the input condition. We test CAN on class-conditional image generation on ImageNet and text-to-image generation on COCO. CAN consistently delivers significant improvements for diffusion transformer models, including DiT and UViT. In particular, CAN combined with EfficientViT (CaT) achieves 2.78 FID on ImageNet 512×512, surpassing DiT-XL/2 while requiring 52x fewer MACs per sampling step.
PAIR Diffusion: A Comprehensive Multimodal Object-Level Image Editor
Vidit Goel, Elia Peruzzo, Yifan Jiang, Dejia Xu, Xingqian Xu, Nicu Sebe, Trevor Darrell, Zhangyang Wang, Humphrey Shi
Generative image editing has recently witnessed extremely fast-paced growth.Some works use high-level conditioning such as text, while others use low-levelconditioning. Nevertheless, most of them lack fine-grained control over the properties of the different objects present in the image, i.e. object-level image editing. In this work, we tackle the task by perceiving the images as an amalgamation ofvarious objects and aim to control the properties of each object in a fine-grainedmanner. Out of these properties, we identify structure and appearance as the mostintuitive to understand and useful for editing purposes. We propose PAIR Diffusion, a generic framework that can enable a diffusion model to control the structure and appearance properties of each object in the image. We show that having controlover the properties of each object in an image leads to comprehensive editingcapabilities. Our framework allows for various object-level editing operations onreal images such as reference image-based appearance editing, free-form shapeediting, adding objects, and variations. Thanks to our design, we do not requireany inversion step. Additionally, we propose multimodal classifier-free guidancewhich enables editing images using both reference images and text when usingour approach with foundational diffusion models. We validate the above claimsby extensively evaluating our framework on both unconditional and foundationaldiffusion models.
Personalized Residuals for Concept-Driven Text-to-Image Generation
Cusuh Ham, Matthew Fisher, James Hays, Nicholas Kolkin, Yuchen Liu, Richard Zhang, Tobias Hinz
We present personalized residuals and localized attention-guided sampling for efficient concept-driven generation using text-to-image diffusion models. Our method first represents concepts by freezing the weights of a pretrained text-conditioned diffusion model and learning low-rank residuals for a small subset of the model’s layers. The residual-based approach then directly enables application of our proposed sampling technique, which applies the learned residuals only in areas where the concept is localized via cross-attention and applies the original diffusion weights in all other regions. Localized sampling therefore combines the learned identity of the concept with the existing generative prior of the underlying diffusion model. We show that personalized residuals effectively capture the identity of a concept in ~3 minutes on a single GPU without the use of regularization images and with fewer parameters than previous models, and localized sampling allows using the original model as strong prior for large parts of the image.
Prompt-Free Diffusion: Taking “Text” out of Text-to-Image Diffusion Models
Xingqian Xu, Jiayi Guo, Zhangyang Wang, Gao Huang, Irfan Essa, Humphrey Shi
Text-to-image (T2I) research has grown explosively in the past year, owing to the large-scale pre-trained diffusion models and many emerging personalization and editing approaches. Yet, one pain point persists: the text prompt engineering, and searching high-quality text prompts for customized results is more art than science. Moreover, as commonly argued: “an image is worth a thousand words” – the attempt to describe a desired image with texts often ends up being ambiguous and cannot comprehensively cover delicate visual details, hence necessitating more additional controls from the visual domain. In this paper, we take a bold step forward: taking “Text” out of a pretrained T2I diffusion model, to reduce the burdensome prompt engineering efforts for users. Our proposed framework, Prompt-Free Diffusion, relies on only visual inputs to generate new images: it takes a reference image as “context”, an optional image structural conditioning, and an initial noise, with absolutely no text prompt. The core architecture behind the scene is Semantic Context Encoder(SeeCoder), substituting the commonly used CLIP-based or LLM-based text encoder. The reusability of SeeCoder also makes it a convenient drop-in component: one can also pre-train a SeeCoder in one T2I model and reuse it for another. Through extensive experiments, Prompt-Free Diffusion is experimentally found to (i) outperform prior exemplar-based image synthesis approaches; (ii) perform on par with state-of-the-art T2I models using prompts following the best practice; and (iii) be naturally extensible to other downstream applications such as anime figure generation and virtual try-on, with promising quality. Our code and models will be open-sourced.
Smooth Diffusion: Crafting Smooth Latent Spaces in Diffusion Models
Jiayi Guo, Xingqian Xu, Yifan Pu, Zanlin Ni, Chaofei Wang, Manushree Vasu, Shiji Song, Gao Huang, Humphrey Shi
Recently, diffusion models have made remarkable progress in text-to-image (T2I) generation, synthesizing images with high fidelity and diverse contents. Despite this advancement, latent space smoothness within diffusion models remains largely unexplored. Smooth latent spaces ensure that a perturbation on an input latent corresponds to a steady change in the output image. This property proves beneficial in downstream tasks, including image interpolation, inversion, and editing. In this work, we expose the non-smoothness of diffusion latent spaces by observing noticeable visual fluctuations resulting from minor latent variations. To tackle this issue, we propose Smooth Diffusion, a new category of diffusion models that can be simultaneously high-performing and smooth. Specifically, we introduce Step-wise Variation Regularization to enforce the proportion between the variations of an arbitrary input latent and that of the output image is a constant at any diffusion training step. In addition, we devise an interpolation standard deviation (ISTD) metric to effectively assess the latent space smoothness of a diffusion model. Extensive quantitative and qualitative experiments demonstrate that Smooth Diffusion stands out as a more desirable solution not only in T2I generation but also across various downstream tasks. Smooth Diffusion is implemented as a plug-and-play Smooth-LoRA to work with various community models. Code is available at https://github.com/SHI-Labs/Smooth-Diffusion.
Zero-Painter: Training-Free Layout Control for Text-to-Image Synthesis
Marianna Ohanyan, Hayk Manukyan, Zhangyang Wang, Shant Navasardyan, Humphrey Shi
We present Zero-Painter, a novel training-free framework for layout-conditional text-to-image synthesis that facilitates the creation of detailed and controlled imagery from textual prompts. Our method utilizes object masks and individual descriptions, coupled with a global text prompt, to generate images with high fidelity. Zero-Painter employs a two-stage process involving our novel Prompt-Adjusted Cross-Attention (PACA) and Region-Grouped Cross-Attention (ReGCA) blocks, ensuring precise alignment of generated objects with textual prompts and mask shapes. Our extensive experiments demonstrate that Zero-Painter surpasses current state-of-the-art methods in preserving textual details and adhering to mask shapes. We will make the codes and the models publicly available.
Multi-modal learning
Yusheng Dai, HangChen , Jun Du, Ruoyu Wang, shihao chen, Haotian Wang, Chin-Hui Lee
Advanced Audio-Visual Speech Recognition (AVSR) systems have been observed to be sensitive to missing video frames, performing even worse than single-modality models. While applying the common dropout techniques to the video modality enhances robustness to missing frames, it simultaneously results in a performance loss when dealing with complete data input. In this study, we delve into this contrasting phenomenon through the lens of modality bias and uncover that an excessive modality bias towards the audio modality induced by dropout constitutes the fundamental cause. Next, we present the Modality Bias Hypothesis (MBH) to systematically describe the relationship between the modality bias and the robustness against missing modality in multimodal systems. Building on these findings, we propose a novel Multimodal Distribution Approximation with Knowledge Distillation (MDA-KD) framework to reduce over-reliance on the audio modality, maintaining performance and robustness simultaneously. Finally, to address an entirely missing modality, we adopt adapters to dynamically switch decision strategies. The effectiveness of our proposed approach is evaluated through comprehensive experiments on the MISP2021 and MISP2022 datasets. Our code is available at https://github.com/dalision/ModalBiasAVSR.
The Audio-Visual Conversational Graph: From an Egocentric-Exocentric Perspective
Wenqi Jia, Miao Liu, Hao Jiang, Ishwarya Ananthabhotla, James Rehg, Vamsi Krishna Ithapu, Ruohan Gao
In recent years, the thriving development of research related to egocentric videos has provided a unique perspective for the study of conversational interactions, where both visual and audio signals play a crucial role. While most prior work focus on learning about behaviors that directly involve the camera wearer, we introduce the Ego-Exocentric Conversational Graph Prediction problem, marking the first attempt to infer exocentric conversational interactions from egocentric videos. We propose a unified multi-modal framework—Audio-Visual Conversational Attention (AV-CONV), for the joint prediction of conversation behaviors—speaking and listening—for both the camera wearer as well as all other social partners present in the egocentric video. Specifically, we adopt the self-attention mechanism to model the representations across-time, across-subjects, and across-modalities. To validate our method, we conduct experiments on a challenging egocentric video dataset that includes multi-speaker and multi-conversation scenarios. Our results demonstrate the superior performance of our method compared to a series of baselines. We also present detailed ablation studies to assess the contribution of each component in our model.
Recognition: Categorization, detection, retrieval
Fan Zhang, Xian-Sheng Hua, Chong Chen, Xiao Luo
This paper studies the problem of semi-supervised 2D-3D retrieval, which aims to align both labeled and unlabeled 2D and 3D data into the same embedding space. The problem is challenging due to the complicated heterogeneous relationships between 2D and 3D data. Moreover, label scarcity in real-world applications hinders from generating discriminative representations. In this paper, we propose a semi-supervised approach named Fine-grained Prototypcical Voting with Heterogeneous Mixup (FIVE), which maps both 2D and 3D data into a common embedding space for cross-modal retrieval. Specifically, we generate fine-grained prototypes to model inter-class variation for both 2D and 3D data. Then, considering each unlabeled sample as a query, we retrieve relevant prototypes to vote for reliable and robust pseudo-labels, which serve as guidance for discriminative learning under label scarcity. Furthermore, to bridge the semantic gap between two modalities, we mix cross-modal pairs with similar semantics in the embedding space and then perform similarity learning for cross-modal discrepancy reduction in a soft manner. The whole FIVE is optimized with the consideration of sharpness to mitigate the impact of potential label noise. Extensive experiments on benchmark datasets validate the superiority of FIVE compared with a range of baselines in different settings. On average, FIVE outperforms the second-best approach by 4.74% on 3D MNIST, 12.94% on ModelNet10, and 22.10% on ModelNet40.
Robotics
Neural Visibility Field for Uncertainty-Driven Active Mapping
Shangjie Xue, Jesse Dill, Pranay Mathur, Frank Dellaert, Panagiotis Tsiotras, Danfei Xu
This paper presents Neural Visibility Field (NVF), a novel uncertainty quantification method for Neural Radiance Fields (NeRF) applied to active mapping. Our key insight is that regions not visible in the training views lead to inherently unreliable color predictions by NeRF at this region, resulting in increased uncertainty in the synthesized views. To address this, we propose to use Bayesian Networks to composite position-based field uncertainty into ray-based uncertainty in camera observations. Consequently, NVF naturally assigns higher uncertainty to unobserved regions, aiding robots to select the most informative next viewpoints. Extensive evaluations show that NVF excels not only in uncertainty quantification but also in scene reconstruction for active mapping, outperforming existing methods. More details can be found at https://sites.google.com/view/nvf-cvpr24/ .
Scene analysis and understanding
VCoder: Versatile Vision Encoders for Multimodal Large Language Models
Jitesh Jain, Jianwei Yang, Humphrey Shi
Humans possess the remarkable skill of Visual Perception, the ability to see and understand the seen, helping them make sense of the visual world and, in turn, reason. Multimodal Large Language Models (MLLM) have recently achieved impressive performance on vision-language tasks ranging from visual question-answering and image captioning to visual reasoning and image generation. However, when prompted to identify or count (perceive) the entities in a given image, existing MLLM systems fail. Working towards developing an accurate MLLM system for perception and reasoning, we propose using Versatile vision enCoders (VCoder) as perception eyes for Multimodal LLMs. We feed the VCoder with perception modalities such as segmentation or depth maps, improving the MLLM’s perception abilities. Secondly, we leverage the images from COCO and outputs from off-the-shelf vision perception models to create our COCO Segmentation Text (COST) dataset for training and evaluating MLLMs on the object perception task. Thirdly, we introduce metrics to assess the object perception abilities in MLLMs on our COST dataset. Lastly, we provide extensive experimental evidence proving the VCoder’s improved object-level perception skills over existing Multimodal LLMs, including GPT-4V. We open-source our dataset, code, and models to promote research.
Segmentation, grouping and shape analysis
Diffuse, Attend, and Segment: Unsupervised Zero-Shot Segmentation using Stable Diffusion
Junjiao Tian, Lavisha Aggarwal, Andrea Colaco, Zsolt Kira, Mar Gonzalez-Franco
Producing quality segmentation masks for images is a fundamental problem in computer vision. Recent research has explored large-scale supervised training to enable zero-shot segmentation on virtually any image style and unsupervised training to enable segmentation without dense annotations. However, constructing a model capable of segmenting anything in a zero-shot manner without any annotations is still challenging. In this paper, we propose to utilize the self-attention layers in stable diffusion models to achieve this goal because the pre-trained stable diffusion model has learned inherent concepts of objects within its attention layers. Specifically, we introduce a simple yet effective iterative merging process based on measuring KL divergence among attention maps to merge them into valid segmentation masks. The proposed method does not require any training or language dependency to extract quality segmentation for any images. On COCO-Stuff-27, our method surpasses the prior unsupervised zero-shot SOTA method by an absolute $26\%$ in pixel accuracy and $17\%$ in mean IoU.
Transfer, meta, low-shot, continual, or long-tail learning
NICE: Neurogenesis Inspired Contextual Encoding for Replay-free Class Incremental Learning
Mustafa B Gurbuz, Jean Moorman, Constantine Dovrolis
Deep neural networks (DNNs) struggle to learn in dynamic settings because they mainly rely on static datasets. Continual learning (CL) aims to overcome this limitation by enabling DNNs to incrementally accumulate knowledge. A widely adopted scenario in CL is class-incremental learning (CIL), where DNNs are required to sequentially learn more classes. Among the various strategies in CL, replay methods, which revisit previous classes, stand out as the only effective ones in CIL. Other strategies, such as architectural modifications to segregate information across weights and protect them from change, are ineffective in CIL. This is because they need additional information during testing to select the correct network parts to use. In this paper, we propose NICE, Neurogenesis Inspired Contextual Encoding, a replay-free architectural method inspired by adult neurogenesis in the hippocampus. NICE groups neurons in the DNN based on different maturation stages and infers which neurons to use during testing without any additional signal. Through extensive experiments across 6 datasets and 3 architectures, we show that NICE performs on par with or often outperforms replay methods. We also make the case that neurons exhibit highly distinctive activation patterns for the classes in which they specialize, enabling us to determine when they should be used. The code is available at https://github.com/BurakGurbuz97/NICE.
Transparency, fairness, accountability, privacy, ethics in vision
OpenBias: Open-set Bias Detection in Text-to-Image Generative Models
Moreno D’Incà, Elia Peruzzo, Massimiliano Mancini, Dejia Xu, Vidit Goel, Xingqian Xu, Zhangyang Wang, Humphrey Shi, Nicu Sebe
Text-to-image generative models are becoming increasingly popular and accessible to the general public. As these models see large-scale deployments, it is necessary to deeply investigate their safety and fairness to not disseminate and perpetuate any kind of biases. However, existing works focus on detecting closed sets of biases defined a priori, limiting the studies to well-known concepts. In this paper, we tackle the challenge of open-set bias detection in text-to-image generative models presenting OpenBias, a new pipeline that identifies and quantifies the severity of biases agnostically, without access to any precompiled set. OpenBias has three stages. In the first phase, we leverage a Large Language Model (LLM) to propose biases given a set of captions. Secondly, the target generative model produces images using the same set of captions. Lastly, a Vision Question Answering model recognizes the presence and extent of the previously proposed biases. We study the behavior of Stable Diffusion 1.5, 2, and XL emphasizing new biases, never investigated before. Via quantitative experiments, we demonstrate that OpenBias agrees with current closed-set bias detection methods and human judgement.
Vision applications and systems
Brush2Prompt: Contextual Prompt Generator for Object Inpainting
Mang Tik Chiu, Yuqian Zhou, Lingzhi Zhang, Zhe Lin, Connelly Barnes, Sohrab Amirghodsi, Eli Shechtman, Humphrey Shi
Object inpainting is a task that involves adding objects to real images and seamlessly compositing them. With the recent commercialization of products like Stable Diffusion and Generative Fill, inserting objects into images by using prompts has achieved impressive visual results. In this paper, we propose a prompt suggestion model to simplify the process of prompt input. When the user provides an image and a mask, our model predicts suitable prompts based on the partial contextual information in the masked image, and the shape and location of the mask. Specifically, we introduce a concept-diffusion in the CLIP space that predicts CLIP-text embeddings from a masked image. These diffused embeddings can be directly injected into open-source inpainting models like Stable Diffusion and its variants. Alternatively, they can be decoded into natural language for use in other publicly available applications such as Generative Fill. Our prompt suggestion model demonstrates a balanced accuracy and diversity, showing its capability to be both contextually aware and creatively adaptive.
Vision, language, and reasoning
Sangmin Lee, Bolin Lai, Fiona Ryan, Bikram Boote, James Rehg
Understanding social interactions involving both verbal (e.g., language) and non-verbal (e.g., gaze, gesture) cues is crucial for developing social artificial intelligence that can engage alongside humans. However, most prior works on multimodal social behaviors focus predominantly on single-person behaviors or rely on holistic visual representations that are not densely aligned to utterances in multi-party environments. They are limited in modeling the intricate dynamics of multi-party interactions. In this paper, we introduce three new challenging tasks to model the fine-grained dynamics between multiple people: speaking target identification, pronoun coreference resolution, and mentioned player prediction. We contribute extensive data annotations to curate these new challenges in social deduction game settings. We further propose a novel multimodal baseline that leverages densely aligned language-visual representations by synchronizing visual features with their corresponding utterances. This facilitates concurrently capturing verbal and non-verbal signals pertinent to social reasoning. Experiments demonstrate the effectiveness of the proposed approach with densely aligned multimodal representations for modeling social interactions. We will release our benchmarks and source code to facilitate further research.
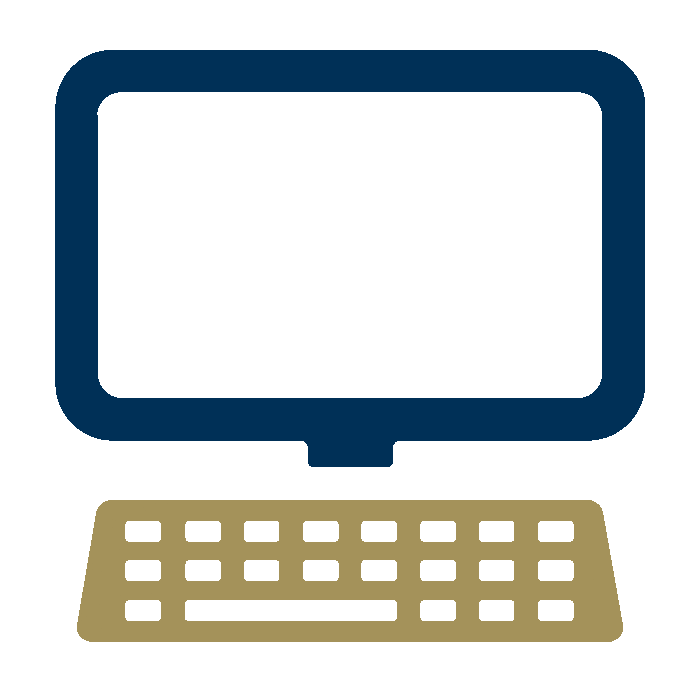
See you in Seattle!
Development: College of Computing
Project Lead/Data Graphics: Joshua Preston
Select Photos: Kevin Beasley
Data Management: Joni Isbell