ICLR 2025
International Conference on Learning Representations | April 24–28, 2025 | Singapore
FEATURED
New Approach for Easily Merging Data Models Brings Adaptable, Multi-Tasking AIs Closer to Reality
Georgia Tech and IBM research ‘significantly enhances existing merging techniques’ for data tasks necessary to advance self-driving vehicles, chat assistants, and other AI applications

FEATURED
New Approach for Easily Merging Data Models Brings Adaptable, Multi-Tasking AIs Closer to Reality
Georgia Tech and IBM research ‘significantly enhances existing merging techniques’ for data tasks necessary to advance self-driving vehicles, chat assistants, and other AI applications

Pictured on the right are the primary investigators Pratik Ramesh and George Stoica. Inset clockwise from left: Leshem Choshen, Judy Hoffman, and Boglárka Ecsedi.
FEATURED
New technique in merging small data models creates pathway for more robust AI applications
Sed ut perspiciatis unde omnis iste natus error sit voluptatem accusantium doloremque la Sed ut perspiciatis unde omnis iste natus error sit voluptatem accusantium doloremque la



By Joshua Preston, College of Computing
A new artificial intelligence innovation developed by Georgia Tech is aiming to create better alignment in specialized data models when combining them to create a single, smarter AI that can do many kinds of tasks well.
The new research tackles the challenge of how to effectively merge existing specialized models that are fine-tuned—or optimized for a particular dataset—and do so using a broadly adopted fine-tuning method called a LoRA. LoRA-based fine-tuning is attractive in part because it changes only a small part of the model, making it cost- and time-effective. They can be applied to a dataset of cars, for example, to fine-tune it to recognize trucks.
“The challenge is that different LoRA fine-tuned models transform data in very conflicting ways, creating a key obstacle to effective merging,” said George Stoica, a Ph.D. student and co-lead investigator for the team. “To address this, we introduced KnOTS, a novel approach that explicitly aligns task-expert models without requiring any data or training.”
The team’s results show KnOTS boosts the performance of existing model-merging methods by up to 4.3% when merging eight vision-based classification models, used for images and video, fine-tuned with LoRA.
“KnOTS significantly enhances existing merging techniques, offering a plug-and-play solution for building smarter, more adaptable AI systems,” said Pratik Ramesh, Ph.D. student and the team’s other co-lead.
“A helpful analogy is to think about comparing and combining various color shades: it’s hard to directly compare them, but if you break each down into their red, green, and blue (RGB) components, they become much easier to compare, adjust and blend.”
At its core, KnOTS makes comparing datasets easier and more accurate. It substantially reduces the conflicts between existing LoRA models and makes it possible to apply other merging algorithms more effectively.
Our method makes this future more realistic by taking a step into the direction of enabling the fusion of specialized AIs into one super-assistant.
Pratik Ramesh
Ph.D. Student, Machine Learning
Merging data models allows for the development of AI apps that can multi-task well. With current AI capabilities, Ramesh said it often feels like people are forced to juggle multiple apps or assistants—”one helps you write, another helps with your taxes, and another translates languages.”
But imagine having one AI that can do all of that effortlessly.
“Our method makes this future more realistic by taking a step into the direction of enabling the fusion of specialized AIs into one super-assistant. This could mean faster, more versatile AI on your phone, smarter tools at work, or even more capable home assistants that understand and adapt to a wide range of needs—all without having to constantly switch between models.”
The team—from the School of Interactive Computing at Georgia Tech, along with a coauthor from IBM and MIT—also developed a new joint-task benchmark combining eight diverse datasets into a single unified task, where “KnOTS outperforms all baselines by up to 3.2%—demonstrating its ability to build truly general models.”
Stoica summarizes the team’s journey in tackling this broad challenge in the current AI landscape:
“From the inception of this project, it felt obvious to us that something was missing or an assumption was being violated when applying existing merging methods on LoRA models. We were really motivated throughout our research to uncover overarching issues shared by these approaches.”
Stoica said that it was very fulfilling to identify a solution that is ultimately both simple and light-weight.
The research paper, “KnOTS: SVD Alignment for LoRA Model Merging,” is part of the proceedings at the International Conference on Learning Representations, April 24-28, 2025, in Singapore. Georgia Tech team members include Stoica and Ramesh, computer science undergrad Boglarka Ecsedi, and Associate Professor Judy Hoffman. IBM and MIT Postdoctoral Researcher Leshem Choshen rounds out the team.
Meet the Team

George Stoica
Ph.D. Student, Machine Learning

Pratik Ramesh
Ph.D. Student, Machine Learning

Boglárka Ecsedi
Computer Science Student in AI, ML, and Theory

Leshem Choshen
Postdoctoral Researcher, IBM and MIT

Judy Hoffman
Associate Professor, Georgia Tech
Georgia Tech at ICLR 2025
By the Numbers
Tech Faculty by Unit
College of Computing
Animesh Garg (IC)
Matthew Gombolay (IC)
Sehoon Ha (IC)
Judy Hoffman (IC)
Zsolt Kira (IC)
Alan Ritter (IC)
Sanne van Waveren (IC)
Danfei Xu (IC)
Wei Xu (IC)
Peng Chen (CSE)
Bo Dai (CSE)
Yunan Luo (CSE)
Lu Mi (CSE)
Qi Tang (CSE)
Chao Zhang (CSE)
Yingyan Celine Lin (CS)
Ling Liu (CS)
Prasad Tetali (Math, CS)
College of Sciences
Partner Organizations
A*STAR • Allen Institute • Allen Institute for Brain Science • Amazon • Apple • Boston University • California Institute of Technology • Carnegie Mellon University • CISPA • Clemson University • Columbia University • Cornell University • EPF Lausanne • Georgia Tech • Google • Harvard University • Hong Kong Polytechnic University • Hong Kong University of Science and Technology • Hunan University • IBM Research AI • Institut de Physique du Globe • IST Audtria • Johannes Kepler University Linz • KAIST • Korea Institute for Advanced Study • Linz University • Massachusetts Institute of Technology • McGill University • Meta • Microsoft Research • Mila • MiraclePlus • MRC Cognition and Brain Sciences Unit • Nankai University • Nanyang Technological University • National Taiwan University • National University of Singapore • New York University • Northeastern University • Northwestern University • NVIDIA • Oklahoma State University • Peking University • Pr(Ai)²R Group • Princeton University • Rutgers University • Salesforce • Samsung • Shanghai Jiao Tong University • Simon Fraser University • Skolkovo Institute of Science and Technology • South China University of Technology • Stanford University • The Chinese University of Hong Kong • The Ohio State University • The Skolkovo Institute of Science and Technology • The University of Edinburgh • Tsinghua University • Universiteit Gent • Universities and Colleges Admissions Service • University College London • University of California Berkeley • University of California San Diego • University of California Los Angeles • University of Central Florida • University of Electronic Science and Technology of China • University of Illinois at Urbana-Champaign • University of Maryland • University of Oregon • University of Oxford • University of Pittsburgh • University of Southern California • University of Texas at Austin • University of Toronto • University of Utah • University of Washington • University of Wuppertal • Vector Institute • Wuhan University • Yale University • Zhejiang University
The Big Picture 
NEWS
Machine Learning Encoder Improves Weather Forecasting and Tsunami Prediction
By Bryant Wine, School of Computational Science and Engineering

Successful test results of a new machine learning (ML) technique developed at Georgia Tech could help communities prepare for extreme weather and coastal flooding. The approach could also be applied to other models that predict how natural systems impact society.
Ph.D. student Phillip Si and Assistant Professor Peng Chen developed Latent-EnSF, a technique that improves how ML models assimilate data to make predictions.
In experiments predicting medium-range weather forecasting and shallow water wave propagation, Latent-EnSF demonstrated higher accuracy, faster convergence, and greater efficiency than existing methods for sparse data assimilation.
“We are currently involved in an NSF-funded project aimed at providing real-time information on extreme flooding events in Pinellas County, Florida,” said Si, who studies computational science and engineering (CSE).
“We’re actively working on integrating Latent-EnSF into the system, which will facilitate accurate and synchronized modeling of natural disasters. This initiative aims to enhance community preparedness and safety measures in response to flooding risks.”
Latent-EnSF outperformed three comparable models in assimilation speed, accuracy, and efficiency in shallow water wave propagation experiments. These tests show models can make better and faster predictions of coastal flood waves, tides, and tsunamis.
In experiments on medium-range weather forecasting, Latent-EnSF surpassed the same three control models in accuracy, convergence, and time. Additionally, this test demonstrated Latent-EnSF’s scalability compared to other methods.
These promising results support using ML models to simulate climate, weather, and other complex systems.
Traditionally, such studies require employment of large, energy-intensive supercomputers. However, advances like Latent-EnSF are making smaller, more efficient ML models feasible for these purposes.



The Georgia Tech team mentioned this comparison in its paper. It takes hours for the European Center for Medium-Range Weather Forecasts computer to run its simulations. Conversely, the ML model FourCastNet calculated the same forecast in seconds.
“Resolution, complexity, and data-diversity will continue to increase into the future,” said Chen, an assistant professor in the School of CSE.
“To keep pace with this trend, we believe that ML models and ML-based data assimilation methods will become indispensable for studying large-scale complex systems.”
Data assimilation is the process by which models continuously ingest new, real-world data to update predictions. This data is often sparse, meaning it is limited, incomplete, or unevenly distributed over time.
Latent-EnSF builds on the Ensemble Filter Scores (EnSF) model developed by Florida State University and Oak Ridge National Laboratory researchers.
EnSF’s strength is that it assimilates data with many features and unpredictable relationships between data points. However, integrating sparse data leads to lost information and knowledge gaps in the model. Also, such large models may stop learning entirely from small amounts of sparse data.

Resolution, complexity, and data-diversity will continue to increase into the future. To keep pace with this trend, we believe that ML models and ML-based data assimilation methods will become indispensable for studying large-scale complex systems.
Peng Chen
Asst. Professor, School of Computational Science and Engineering at Georgia Tech
The Georgia Tech researchers employ two variational autoencoders (VAEs) in Latent-EnSF to help ML models integrate and use real-world data. The VAEs encode sparse data and predictive models together in the same space to assimilate data more accurately and efficiently.
Integrating models with new methods, like Latent-EnSF, accelerates data assimilation. Producing accurate predictions more quickly during real-world crises could save lives and property for communities.
To share Latent-EnSF to the broader research community, Chen and Si presented their paper at the SIAM Conference on Computational Science and Engineering (CSE25). The Society of Industrial and Applied Mathematics (SIAM) organized CSE25, held March 3-7 in Fort Worth, Texas.
Chen was one of ten School of CSE faculty members who presented research at CSE25, representing one-third of the School’s faculty body. Latent-EnSF was one of 15 papers by School of CSE authors and one of 23 Georgia Tech papers presented at the conference.
The pair will also present Latent-EnSF at the upcoming International Conference on Learning Representations (ICLR 2025). Occurring April 24-28 in Singapore, ICLR is one of the world’s most prestigious conferences dedicated to artificial intelligence research.
“We hope to bring attention to experts and domain scientists the exciting area of ML-based data assimilation by presenting our paper,” Chen said. “Our work offers a new solution to address some of the key shortcomings in the area for broader applications.”
RESEARCH 
ORAL: A Theoretically-Principled Sparse, Connected, and Rigid Graph Representation of Molecules
Shih-Hsin Wang, Yuhao Huang, Justin Baker, Yuan-En Sun, Qi Tang, Bao Wang
Abstract
Graph neural networks (GNNs) — learn graph representations by exploiting graph’s sparsity, connectivity, and symmetries — have become indispensable for learning geometric data like molecules. However, the most used graphs (e.g., radial cutoff graphs) in molecular modeling lack theoretical guarantees for achieving connectivity and sparsity simultaneously, which are essential for the performance and scalability of GNNs. Furthermore, existing widely used graph construction methods for molecules lack rigidity, limiting GNNs’ ability to exploit graph nodes’ spatial arrangement. In this paper, we introduce a new hyperparameter-free graph construction of molecules and beyond with sparsity, connectivity, and rigidity guarantees. Remarkably, our method consistently generates connected and sparse graphs with the edge-to-node ratio being bounded above by 3. Our graphs’ rigidity guarantees that edge distances and dihedral angles are sufficient to uniquely determine general spatial arrangements of atoms. We substantiate the effectiveness and efficiency of our proposed graphs in various molecular modeling benchmarks.
ORAL: Booster: Tackling Harmful Fine-tuning for Large Language Models via Attenuating Harmful Perturbation
Tiansheng Huang, Sihao Hu, Fatih Ilhan, Selim Tekin, Ling Liu
Abstract
Harmful fine-tuning attack \citep{qi2023fine} poses serious safety concerns for Large language models’ fine-tuning-as-a-service. While existing defenses have been proposed to mitigate the issue, their performances are still far away from satisfactory, and the root cause of the problem has not been fully recovered. To this end, we in this paper show that \textit{harmful perturbation} over the model weights could be a probable cause of alignment-broken. In order to attenuate the negative impact of harmful perturbation, we propose an alignment-stage solution, dubbed Booster. Technically, along with the original alignment loss, we append a loss regularizer in the alignment stage’s optimization. The regularizer ensures that the model’s harmful loss reduction after the simulated harmful perturbation is attenuated, thereby mitigating the subsequent fine-tuning risk. Empirical results show that Booster can effectively reduce the harmful score of the fine-tuned models while maintaining the performance of downstream tasks. Our code is available at \url{https://anonymous.4open.science/r/Booster-EF18}.
ORAL: Cut Your Losses in Large-Vocabulary Language Models
Erik Wijmans, Brody Huval, Alexander Hertzberg, Vladlen Koltun, Philipp Krähenbühl
Abstract
As language models grow ever larger, so do their vocabularies.This has shifted the memory footprint of LLMs during training disproportionately to one single layer: the cross-entropy in the loss computation.Cross-entropy builds up a logit matrix with entries for each pair of input tokens and vocabulary items and, for small models, consumes an order of magnitude more memory than the rest of the LLM combined.We propose Cut Cross-Entropy (CCE), a method that computes the cross-entropy loss without materializing the logits for all tokens into global memory.Rather, CCE only computes the logit for the correct token and evaluates the log-sum-exp over all logits on the fly.We implement a custom kernel that performs the matrix multiplications and the log-sum-exp reduction over the vocabulary in flash memory, making global memory consumption for the cross-entropy computation negligible. This has a dramatic effect. Taking the Gemma 2 (2B) model as an example, CCE reduces the memory footprint of the loss computation from 24 GB to 1 MB, and the total training-time memory consumption of the classifier head from 28 GB to 1 GB.To improve the throughput of CCE, we leverage the inherent sparsity of softmax and propose to skip elements of the gradient computation that have a negligible (i.e. below numerical precision) contribution to the gradient.Experiments demonstrate that the dramatic reduction in memory consumption is accomplished without sacrificing training speed or convergence.
ORAL: Feedback Schrödinger Bridge Matching
Panagiotis Theodoropoulos, Guan-Horng Liu, Nikolaos Komianos, Vincent Pacelli, Evangelos Theodorou
Abstract
Recent advancements in diffusion bridges for distribution transport problems have heavily relied on matching frameworks, yet existing methods often face a trade-off between scalability and access to optimal pairings during training. Fully unsupervised methods make minimal assumptions but incur high computational costs, limiting their practicality. On the other hand, imposing full supervision of the matching process with optimal pairings improves scalability, however, it can be infeasible in most applications.To strike a balance between scalability and minimal supervision, we introduce Feedback Schrödinger Bridge Matching (FSBM), a novel semi-supervised matching framework that incorporates a small portion ($<8$% of the entire dataset) of pre-aligned pairs as state feedback to guide the transport map of non-coupled samples, thereby significantly improving efficiency. This is achieved by formulating a static Entropic Optimal Transport (EOT) problem with an additional term capturing the semi-supervised guidance. The generalized EOT objective is then recast into a dynamic formulation to leverage the scalability of matching frameworks. Extensive experiments demonstrate that FSBM accelerates training and enhances generalization by leveraging coupled pairs’ guidance, opening new avenues for training matching frameworks with partially aligned datasets.
ORAL: MoDeGPT: Modular Decomposition for Large Language Model Compression
Chi-Heng Lin, Shangqian Gao, James Smith, Abhishek Patel, Shikhar Tuli, Yilin Shen, Hongxia Jin, Yen-Chang Hsu
Abstract
Large Language Models (LLMs) have significantly advanced AI with their exceptional performance across a wide range of tasks. However, their extensive computational requirements restrict their use on devices with limited resources.While recent compression methods based on low-rank matrices show potentialsolutions, they often suffer from significant loss of accuracy or introduce substantialoverhead in parameters and inference time. In this paper, we introduce Modular De-composition (MoDeGPT), a new, efficient, and structured compression frameworkthat overcomes these limitations. MoDeGPT jointly decomposes pairs of consecu-tive subcomponents within Transformer blocks, reduces hidden dimensions throughoutput reconstruction on a larger structural scale than conventional low-rank meth-ods, and repurposes three classical matrix decomposition algorithms—Nyströmapproximation, CR decomposition, and SVD—to ensure bounded errors in ournovel decomposition approach. Our experiments show that MoDeGPT, withoutrelying on backward propagation, consistently matches or surpasses the performance of prior techniques that depend on gradient information, while achieving a98% reduction in compute costs when compressing a 13B-parameter model. OnLLaMA-2/3 and OPT models, MoDeGPT retains 90-95% of zero-shot performancewith compression rates of 25-30%. The compression process can be completed ona single GPU in a few hours, boosting inference throughput by up to 46%.
ORAL: ReGenesis: LLMs can Grow into Reasoning Generalists via Self-Improvement
XIANGYU PENG, Congying Xia, Xinyi Yang, Caiming Xiong, Chien-Sheng Wu, Chen Xing
Abstract
Post-training Large Language Models (LLMs) with explicit reasoning trajectories can enhance their reasoning abilities. However, acquiring such high-quality trajectory data typically demands meticulous supervision from humans or superior models, which can be either expensive or license-constrained. In this paper, we explore how far an LLM can improve its reasoning by self-synthesizing reasoning paths as training data without any additional supervision. Existing self-synthesizing methods, such as STaR, suffer from poor generalization to out-of-domain (OOD) reasoning tasks. We hypothesize it is due to that their self-synthesized reasoning paths are too task-specific, lacking general task-agnostic reasoning guidance. To address this, we propose **Reasoning Generalist via Self-Improvement (ReGenesis)**, a method to *self-synthesize reasoning paths as post-training data by progressing from abstract to concrete*. More specifically, ReGenesis self-synthesizes reasoning paths by converting general reasoning guidelines into task-specific ones, generating reasoning structures, and subsequently transforming these structures into reasoning paths, without the need for human-designed task-specific examples used in existing methods. We show that ReGenesis achieves superior performance on all in-domain and OOD settings tested compared to existing methods. For six OOD tasks specifically, while previous methods exhibited an average performance decrease of approximately 4.6% after post training, ReGenesis delivers around 6.1% performance improvement. We also conduct an in-depth analysis of our framework and show ReGenesis is effective across various language models and design choices.
ORAL: TopoLM: brain-like spatio-functional organization in a topographic language model
Neil Rathi, Johannes Mehrer, Badr AlKhamissi, Taha Binhuraib, Nicholas Blauch, Martin Schrimpf
Abstract
Neurons in the brain are spatially organized such that neighbors on tissue often exhibit similar response profiles. In the human language system, experimental studies have observed clusters for syntactic and semantic categories, but the mechanisms underlying this functional organization remain unclear. Here, building on work from the vision literature, we develop TopoLM, a transformer language model with an explicit two-dimensional spatial representation of model units. By combining a next-token prediction objective with a spatial smoothness loss, representations in this model assemble into clusters that correspond to semantically interpretable groupings of text and closely match the functional organization in the brain’s language system. TopoLM successfully predicts the emergence of the spatio-functional organization of a cortical language system as well as the organization of functional clusters selective for fine-grained linguistic features empirically observed in human cortex. Our results suggest that the functional organization of the human language system is driven by a unified spatial objective, and provide a functionally and spatially aligned model of language processing in the brain.
SPOTLIGHT: Eagle: Exploring The Design Space for Multimodal LLMs with Mixture of Encoders
Min Shi, Fuxiao Liu, Shihao Wang, Shijia Liao, Subhashree Radhakrishnan, Yilin Zhao, De-An Huang, Hongxu Yin, Karan Sapra, Yaser Yacoob, Humphrey Shi, Bryan Catanzaro, Andrew Tao, Jan Kautz, Zhiding Yu, Guilin Liu
Abstract
The ability to accurately interpret complex visual information is a crucial topic of multimodal large language models (MLLMs). Recent work indicates that enhanced visual perception significantly reduces hallucinations and improves performance on resolution-sensitive tasks, such as optical character recognition and document analysis. A number of recent MLLMs achieve this goal using a mixture of vision encoders. Despite their success, there is a lack of systematic comparisons and detailed ablation studies addressing critical aspects, such as expert selection and the integration of multiple vision experts. This study provides an extensive exploration of the design space for MLLMs using a mixture of vision encoders and resolutions. Our findings reveal several underlying principles common to various existing strategies, leading to a streamlined yet effective design approach. We discover that simply concatenating visual tokens from a set of complementary vision encoders is as effective as more complex mixing architectures or strategies. We additionally introduce Pre-Alignment to bridge the gap between vision-focused encoders and language tokens, enhancing model coherence. The resulting family of MLLMs, Eagle, surpasses other leading open-source models on major MLLM benchmarks.
SPOTLIGHT: Grounding Video Models to Actions through Goal Conditioned Exploration
Yunhao Luo, Yilun Du
Abstract
Large video models, pretrained on massive quantities of amount of Internet video, provide a rich source of physical knowledge about the dynamics and motions of objects and tasks.However, video models are not grounded in the embodiment of an agent, and do not describe how to actuate the world to reach the visual states depicted in a video.To tackle this problem, current methods use a separate vision-based inverse dynamic model trained on embodiment-specific data to map image states to actions. Gathering data to train such a model is often expensive and challenging, and this model is limited to visual settings similar to the ones in which data is available.In this paper, we investigate how to directly ground video models to continuous actions through self-exploration in the embodied environment — using generated video states as visual goals for exploration.We propose a framework that uses trajectory level action generation in combination with video guidance toenable an agent to solve complex tasks without any external supervision, e.g., rewards, action labels, or segmentation masks.We validate the proposed approach on 8 tasks in Libero, 6 tasks in MetaWorld, 4 tasks in Calvin, and 12 tasks in iThor Visual Navigation. We show how our approach is on par with or even surpasses multiple behavior cloning baselines trained on expert demonstrations while without requiring any action annotations.
SPOTLIGHT: Hymba: A Hybrid-head Architecture for Small Language Models
Xin Dong, Yonggan Fu, Shizhe Diao, Wonmin Byeon, ZIJIA CHEN, Ameya Mahabaleshwarkar, Shih-Yang Liu, Matthijs Van keirsbilck, Min-Hung Chen, Yoshi Suhara, Yingyan Celine Lin, Jan Kautz, Pavlo Molchanov
Abstract
The transformative capabilities of language models (LMs) have intensified the demand for their deployment on everyday devices, necessitating efficient processing for on-device language tasks. To address this, we propose Hymba, a new family of small language models featuring a hybrid-head architecture that strategically integrates attention mechanisms with state space models (SSMs). This architecture leverages the strengths of both systems: attention heads provide high-resolution recall, akin to snapshot memories in the human brain, while SSM heads offer efficient context summarization, similar to fading memories. To further enhance Hymba’s performance, we introduce learnable meta tokens that are prepended to input sequences and jointly trained with model weights during pretraining. These meta tokens act as a learned cache initialization during inference, modulating all subsequent tokens within the hybrid heads and boosting the model’s focus on salient information, similar to metamemory. Extensive experiments and ablation studies demonstrate that Hymba sets new state-of-the-art results for small LMs across various benchmarks and advances the accuracy-efficiency trade-offs of small LMs. For instance, Hymba-1.5B achieves comparable commonsense reasoning accuracy to LLaMA 3.2 3B while being 3.49x faster and offering a 14.72x reduction in cache size. All codes and models will be released upon acceptance.
SPOTLIGHT: In vivo cell-type and brain region classification via multimodal contrastive learning
Han Yu, Hanrui Lyu, YiXun Xu, Charlie Windolf, Eric Lee, Fan Yang, Andrew Shelton, Olivier Winter, International Brain Laboratory, Eva Dyer, Chandramouli Chandrasekaran, Nicholas Steinmetz, Liam Paninski, Cole Hurwitz
Abstract
Current electrophysiological approaches can track the activity of many neurons, yet it is usually unknown which cell-types or brain areas are being recorded without further molecular or histological analysis. Developing accurate and scalable algorithms for identifying the cell-type and brain region of recorded neurons is thus crucial for improving our understanding of neural computation. In this work, we develop a multimodal contrastive learning approach for neural data that can be fine-tuned for different downstream tasks, including inference of cell-type and brain location. We utilize multimodal contrastive learning to jointly embed the activity autocorrelations and extracellular waveforms of individual neurons. We demonstrate that our embedding approach, Neuronal Embeddings via MultimOdal Contrastive Learning (NEMO), paired with supervised fine-tuning, achieves state-of-the-art cell-type classification for an opto-tagged visual cortex dataset and for brain region classification of the public International Brain Laboratory brain-wide map dataset. Our method represents a promising step towards accurate cell-type and brain region classification from electrophysiological recordings.
SPOTLIGHT: LoRA3D: Low-Rank Self-Calibration of 3D Geometric Foundation models
Ziqi Lu, Heng Yang, Danfei Xu, Boyi Li, Boris Ivanovic, Marco Pavone, Yue Wang
Abstract
Emerging 3D geometric foundation models, such as DUSt3R, offer a promising approach for in-the-wild 3D vision tasks.However, due to the high-dimensional nature of the problem space and scarcity of high-quality 3D data,these pre-trained models still struggle to generalize to many challenging circumstances,such as limited view overlap or low lighting.To address this, we propose LoRA3D, an efficient self-calibration pipeline to *specialize* the pre-trained models to target scenes using their own multi-view predictions.Taking sparse RGB images as input, we leverage robust optimization techniques to refine multi-view predictions and align them into a global coordinate frame.In particular, we incorporate prediction confidence into the geometric optimization process, automatically re-weighting the confidence to better reflect point estimation accuracy. We use the calibrated confidence to generate high-quality pseudo labels for the calibrating views and fine-tune the models using low-rank adaptation (LoRA) on the pseudo-labeled data.Our method does not require any external priors or manual labels. It completes the self-calibration process on a **single standard GPU within just 5 minutes**.Each low-rank adapter requires only **18MB** of storage. We evaluated our method on **more than 160 scenes** from the Replica, TUM and Waymo Open datasets,achieving up to **88\% performance improvement** on 3D reconstruction, multi-view pose estimation and novel-view rendering.
SPOTLIGHT: Multi-session, multi-task neural decoding from distinct cell-types and brain regions
Mehdi Azabou, Krystal Pan, Vinam Arora, Ian Knight, Eva Dyer, Blake A Richards
Abstract
Recent work has shown that scale is important for improved brain decoding, with more data leading to greater decoding accuracy. However, large-scale decoding across many different datasets is challenging because neural circuits are heterogeneous—each brain region contains a unique mix of cellular sub-types, and the responses to different stimuli are diverse across regions and sub-types. It is unknown whether it is possible to pre-train and transfer brain decoding models between distinct tasks, cellular sub-types, and brain regions. To address these questions, we developed a multi-task transformer architecture and trained it on the entirety of the Allen Institute’s Brain Observatory dataset. This dataset contains responses from over 100,000 neurons in 6 areas of the brains of mice, observed with two-photon calcium imaging, recorded while the mice observed different types of visual stimuli. Our results demonstrate that transfer is indeed possible -combining data from different sources is beneficial for a number of downstream decoding tasks. As well, we can transfer the model between regions and sub-types, demonstrating that there is in fact common information in diverse circuits that can be extracted by an appropriately designed model. Interestingly, we found that the model’s latent representations showed clear distinctions between different brain regions and cellular sub-types, even though it was never given any information about these distinctions. Altogether, our work demonstrates that training a large-scale neural decoding model on diverse data is possible, and this provides a means of studying the differences and similarities between heterogeneous neural circuits.
Wuwei Zhang, Ziyu Lu, Trung Le, Hao Wang, Uygar Sümbül, Eric SheaBrown, Lu Mi
Abstract
Neuronal dynamics are highly nonlinear and nonstationary. Traditional methods for extracting the underlying network structure from neuronal activity recordings mainly concentrate on modeling static connectivity, without accounting for key nonstationary aspects of biological neural systems, such as ongoing synaptic plasticity and neuronal modulation. To bridge this gap, we introduce the NetFormer model, an interpretable approach applicable to such systems. In NetFormer, the activity of each neuron across a series of historical time steps is defined as a token. These tokens are then linearly mapped through a query and key mechanism to generate a state- (and hence time-) dependent attention matrix that directly encodes nonstationary connectivity structures. We analyze our formulation from the perspective of nonstationary and nonlinear networked dynamical systems, and show both via an analytical expansion and targeted simulations how it can approximate the underlying ground truth. Next, we demonstrate NetFormer’s ability to model a key feature of biological networks, spike-timing-dependent plasticity, whereby connection strengths continually change in response to local activity patterns. We further demonstrate that NetFormer can capture task-induced connectivity patterns on activity generated by task-trained recurrent neural networks. Thus informed, we apply NetFormer to a multi-modal dataset of real neural recordings, which contains neural activity, cell type, and behavioral state information. We show that the NetFormer effectively predicts neural dynamics and identifies cell-type specific, state-dependent dynamic connectivity that matches patterns measured in separate ground-truth physiology experiments, demonstrating its ability to help decode complex neural interactions based on population activity observations alone.
SPOTLIGHT: Nonlinear Sequence Embedding by Monotone Variational Inequality
Jonathan Zhou, Yao Xie
Abstract
In the wild, we often encounter collections of sequential data such as electrocardiograms, motion capture, genomes, and natural language, and sequences may be multichannel or symbolic with nonlinear dynamics. We introduce a method to learn low-dimensional representations of nonlinear sequence and time-series data without supervision which has provable recovery guarantees. The learned representation can be used for downstream machine-learning tasks such as clustering and classification. The method assumes that the observed sequences arise from a common domain, with each sequence following its own autoregressive model, and these models are related through low-rank regularization. We cast the problem as a convex matrix parameter recovery problem using monotone variational inequalities (VIs) and encode the common domain assumption via low-rank constraint across the learned representations, which can learn a subspace approximately spanning the entire domain as well as faithful representations for the dynamics of each individual sequence incorporating the domain information in totality. We show the competitive performance of our method on real-world time-series data with baselines and demonstrate its effectiveness for symbolic text modeling and RNA sequence clustering.
SPOTLIGHT: Preference Optimization for Reasoning with Pseudo Feedback
Fangkai Jiao, Geyang Guo, Xingxing Zhang, Nancy F Chen, Shafiq Joty, Furu Wei
Abstract
Preference optimization techniques, such as Direct Preference Optimization (DPO), are frequently employed to enhance the reasoning capabilities of large language models (LLMs) in domains like mathematical reasoning and coding, typically following supervised fine-tuning. These methods rely on high-quality labels for reasoning tasks to generate preference pairs; however, the availability of reasoning datasets with human-verified labels is limited.In this study, we introduce a novel approach to generate pseudo feedback for reasoning tasks by framing the labeling of solutions to reason problems as an evaluation against associated \emph{test cases}. We explore two forms of pseudo feedback based on test cases: one generated by frontier LLMs and the other by extending self-consistency to multi-test-case.We conduct experiments on both mathematical reasoning and coding tasks using pseudo feedback for preference optimization, and observe improvements across both tasks. Specifically, using Mathstral-7B as our base model, we improve MATH results from 58.3 to 68.6, surpassing both NuminaMath-72B and GPT-4-Turbo-1106-preview. In GSM8K and College Math, our scores increase from 85.6 to 90.3 and from 34.3 to 42.3, respectively. Building on Deepseek-coder-7B-v1.5, we achieve a score of 24.3 on LiveCodeBench (from 21.1), surpassing Claude-3-Haiku.
SPOTLIGHT: TopoNets: High performing vision and language models with brain-like topography
Mayukh Deb, Mainak Deb, Ratan Murty
Abstract
Neurons in the brain are organized such that nearby cells tend to share similar functions. AI models lack this organization, and past efforts to introduce topography have often led to trade-offs between topography and task performance. In this work, we present *TopoLoss*, a new loss function that promotes spatially organized topographic representations in AI models without significantly sacrificing task performance. TopoLoss is highly adaptable and can be seamlessly integrated into the training of leading model architectures. We validate our method on both vision (ResNet-18, ResNet-50, ViT) and language models (GPT-Neo-125M, NanoGPT), collectively *TopoNets*. TopoNets are the highest performing supervised topographic models to date, exhibiting brain-like properties such as localized feature processing, lower dimensionality, and increased efficiency. TopoNets also predict responses in the brain and replicate the key topographic signatures observed in the brain’s visual and language cortices, further bridging the gap between biological and artificial systems. This work establishes a robust and generalizable framework for integrating topography into AI, advancing the development of high performing models that more closely emulate the computational strategies of the human brain.
BingoGuard: LLM Content Moderation Tools with Risk Levels
Fan Yin, Philippe Laban, XIANGYU PENG, Yilun Zhou, Yixin Mao, Vaibhav Vats, Linnea Ross, Divyansh Agarwal, Caiming Xiong, Chien-Sheng Wu
Abstract
Malicious content generated by large language models (LLMs) can pose varying degrees of harm. Although existing LLM-based moderators can detect harmful content, they struggle to assess risk levels and may miss lower-risk outputs. Accurate risk assessment allows platforms with different safety thresholds to tailor content filtering and rejection. In this paper, we introduce per-topic severity rubrics for 11 harmful topics and build BingoGuard, an LLM-based moderation system designed to predict both binary safety labels and severity levels. To address the lack of annotations on levels of severity, we propose a scalable generate-then-filter framework that first generates responses across different severity levels and then filters out low-quality responses. Using this framework, we create BingoGuardTrain, a training dataset with 54,897 examples covering a variety of topics, response severity, styles, and BingoGuardTest, a test set with 988 examples explicitly labeled based on our severity rubrics that enables fine-grained analysis on model behaviors on different severity levels. Our BingoGuard-8B, trained on BingoGuardTrain, achieves the state-of-the-art performance on several moderation benchmarks, including WildGuardTest and HarmBench, as well as BingoGuardTest, outperforming best public models, WildGuard, by 4.3\%. Our analysis demonstrates that incorporating severity levels into training significantly enhances detection performance and enables the model to effectively gauge the severity of harmful responses. Warning: this paper includes red-teaming examples that may be harmful in nature.
Captured by Captions: On Memorization and its Mitigation in CLIP Models
Wenhao Wang, Adam Dziedzic, Grace Kim, Michael Backes, Franziska Boenisch
Abstract
Multi-modal models, such as CLIP, have demonstrated strong performance in aligning visual and textual representations, excelling in tasks like image retrieval and zero-shot classification. Despite this success, the mechanisms by which these models utilize training data, particularly the role of memorization, remain unclear. In uni-modal models, both supervised and self-supervised, memorization has been shown to be essential for generalization. However, it is not well understood how these findings would apply to CLIP, which incorporates elements from both supervised learning via captions that provide a supervisory signal similar to labels, and from self-supervised learning via the contrastive objective.To bridge this gap in understanding, we propose a formal definition of memorization in CLIP (CLIPMem) and use it to quantify memorization in CLIP models. Our results indicate that CLIP’s memorization behavior falls between the supervised and self-supervised paradigms, with “mis-captioned” samples exhibiting highest levels of memorization. Additionally, we find that the text encoder contributes more to memorization than the image encoder, suggesting that mitigation strategies should focus on the text domain. Building on these insights, we propose multiple strategies to reduce memorization while at the same time improving utility—something that had not been shown before for traditional learning paradigms where reducing memorization typically results in utility decrease.
CHASE-SQL: Multi-Path Reasoning and Preference Optimized Candidate Selection in Text-to-SQL
Mohammadreza Pourreza, Hailong Li, Ruoxi Sun, Yeounoh Chung, Shayan Talaei, Gaurav Tarlok Kakkar, Yu Gan, Amin Saberi, Fatma Ozcan, Sercan Arik
Abstract
In addressing the challenges of improving large language model (LLM) performance for Text-to-SQL tasks, we propose a new framework, CHASE-SQL, that is comprised of innovative strategies that leverage judiciously-designed test-time compute in multi-agent modeling to enhance candidate generation and selection. Our approach leverages LLMs’ intrinsic knowledge to generate diverse and high-quality SQL candidates using different LLM generators with: (1) a divide-and-conquer method that decomposes complex queries into manageable sub-queries in a single LLM call; (2) chain-of-thought reasoning based on query execution plans, reflecting the steps a database engine takes during execution; and (3) a unique instance-aware synthetic example generation technique, which offers specific few-shot demonstrations tailored to test questions. To identify the best candidate, a selection agent is employed to rank the candidates through pairwise comparisons with a fine-tuned binary-candidates selection LLM. This selection approach has been demonstrated more robust over alternatives. The proposed generators-selector framework not only enhances the quality and diversity of SQL queries but also outperforms previous methods. Overall, our proposed CHASE-SQL achieves the state-of-the-art execution accuracy of 73.0 % and 73.01% on the test set and development set of the notable BIRD Text-to-SQL dataset bench-mark, rendering CHASE-SQL the top submission of the leaderboard (at the time of paper submission)
Contextual Self-paced Learning for Weakly Supervised Spatio-Temporal Video Grounding
Akash Kumar, Zsolt Kira, Yogesh S Rawat
Abstract
In this work, we focus on Weakly Supervised Spatio-Temporal Video Grounding (WSTVG). It is a multimodal task aimed at localizing specific subjects spatio-temporally based on textual queries without bounding box supervision. Motivated by recent advancements in multi-modal foundation models for grounding tasks, we first explore the potential of state-of-the-art object detection models for WSTVG. Despite their robust zero-shot capabilities, our adaptation reveals significant limitations, including inconsistent temporal predictions, inadequate understanding of complex queries, and challenges in adapting to difficult scenarios.We propose CoSPaL (Contextual Self-Paced Learning), a novel approach which is designed to overcome these limitations. CoSPaL integrates three core components: (1) Tubelet Phrase Grounding (TPG), which introduces spatio-temporal prediction by linking textual queries to tubelets; (2) Contextual Referral Grounding (CRG), which improves comprehension of complex queries by extracting contextual information to refine object identification over time; and (3) Self-Paced Scene Understanding (SPS), a training paradigm that progressively increases task difficulty, enabling the model to adapt to complex scenarios by transitioning from coarse to fine-grained understanding.
Convergent Privacy Loss of Noisy-SGD without Convexity and Smoothness
Eli Chien, Pan Li
Abstract
We study the Differential Privacy (DP) guarantee of hidden-state Noisy-SGD algorithms over a bounded domain. Standard privacy analysis for Noisy-SGD assumes all internal states are revealed, which leads to a divergent R\’enyi DP bound with respect to the number of iterations. Ye & Shokri (2022) and Altschuler & Talwar (2022) proved convergent bounds for smooth (strongly) convex losses, and raise open questions about whether these assumptions can be relaxed. We provide positive answers by proving convergent R\’enyi DP bound for non-convex non-smooth losses, where we show that requiring losses to have H\”older continuous gradient is sufficient. We also provide a strictly better privacy bound compared to state-of-the-art results for smooth strongly convex losses. Our analysis relies on the improvement of shifted divergence analysis in multiple aspects, including forward Wasserstein distance tracking, identifying the optimal shifts allocation, and the H\”older reduction lemma. Our results further elucidate the benefit of hidden-state analysis for DP and its applicability.
Deep Distributed Optimization for Large-Scale Quadratic Programming
Augustinos Saravanos, Hunter Kuperman, Alex Oshin, Arshiya Taj Abdul, Vincent Pacelli, Evangelos Theodorou
Abstract
Quadratic programming (QP) forms a crucial foundation in optimization, encompassing a broad spectrum of domains and serving as the basis for more advanced algorithms. Consequently, as the scale and complexity of modern applications continue to grow, the development of efficient and reliable QP algorithms becomes increasingly vital. In this context, this paper introduces a novel deep learning-aided distributed optimization architecture designed for tackling large-scale QP problems. First, we combine the state-of-the-art Operator Splitting QP (OSQP) method with a consensus approach to derive DistributedQP, a new method tailored for network-structured problems, with convergence guarantees to optimality. Subsequently, we unfold this optimizer into a deep learning framework, leading to DeepDistributedQP, which leverages learned policies to accelerate reaching to desired accuracy within a restricted amount of iterations. Our approach is also theoretically grounded through Probably Approximately Correct (PAC)-Bayes theory, providing generalization bounds on the expected optimality gap for unseen problems. The proposed framework, as well as its centralized version DeepQP, significantly outperform their standard optimization counterparts on a variety of tasks such as randomly generated problems, optimal control, linear regression, transportation networks and others. Notably, DeepDistributedQP demonstrates strong generalization by training on small problems and scaling to solve much larger ones (up to 50K variables and 150K constraints) using the same policy. Moreover, it achieves orders-of-magnitude improvements in wall-clock time compared to OSQP. The certifiable performance guarantees of our approach are also demonstrated, ensuring higher-quality solutions over traditional optimizers.
Diffusion Generative Modeling for Spatially Resolved Gene Expression Inference from Histology Images
Sichen Zhu, Yuchen Zhu, Molei Tao, Peng Qiu
Abstract
Spatial Transcriptomics (ST) allows a high-resolution measurement of RNA sequence abundance by systematically connecting cell morphology depicted in Hematoxylin and eosin (H\&E) stained histology images to spatially resolved gene expressions. ST is a time-consuming, expensive yet powerful experimental technique that provides new opportunities to understand cancer mechanisms at a fine-grained molecular level, which is critical for uncovering new approaches for disease diagnosis and treatments. Here, we present $\textbf{Stem}$ ($\underline{\textbf{S}}$pa$\underline{\textbf{T}}$ially resolved gene $\underline{\textbf{E}}$xpression inference with diffusion $\underline{\textbf{M}}$odel), a novel computational tool that leverages a conditional diffusion generative model to enable in silico gene expression inference from H&E stained images. Through better capturing the inherent stochasticity and heterogeneity in ST data, $\textbf{Stem}$ achieves state-of-the-art performance on spatial gene expression prediction and generates biologically meaningful gene profiles for new H&E stained images at test time. We evaluate the proposed algorithm on datasets with various tissue sources and sequencing platforms, where it demonstrates clear improvement over existing approaches. $\textbf{Stem}$ generates high-fidelity gene expression predictions that share similar gene variation levels as ground truth data, suggesting that our method preserves the underlying biological heterogeneity. Our proposed pipeline opens up the possibility of analyzing existing, easily accessible H&E stained histology images from a genomics point of view without physically performing gene expression profiling and empowers potential biological discovery from H&E stained histology images.
Diffusion Transformer Captures Spatial-Temporal Dependencies: A Theory for Gaussian Process Data
Hengyu Fu, Zehao Dou, Jiawei Guo, Mengdi Wang, Minshuo Chen
Abstract
Diffusion Transformer, the backbone of Sora for video generation, successfully scales the capacity of diffusion models, pioneering new avenues for high-fidelity sequential data generation. Unlike static data such as images, sequential data consists of consecutive data frames indexed by time, exhibiting rich spatial and temporal dependencies. These dependencies represent the underlying dynamic model and are critical to validate the generated data. In this paper, we make the first theoretical step towards bridging diffusion transformers for capturing spatial-temporal dependencies. Specifically, we establish score approximation and distribution estimation guarantees of diffusion transformers for learning Gaussian process data with covariance functions of various decay patterns. We highlight how the spatial-temporal dependencies are captured and affect learning efficiency. Our study proposes a novel transformer approximation theory, where the transformer acts to unroll an algorithm. We support our theoretical results by numerical experiments, providing strong evidence that spatial-temporal dependencies are captured within attention layers, aligning with our approximation theory.
Directional Gradient Projection for Robust Fine-tuning of Foundation Models
Chengyue Huang, Junjiao Tian, Brisa Maneechotesuwan, Shivang Chopra, Zsolt Kira
Abstract
Robust fine-tuning aims to adapt large foundation models to downstream tasks while preserving their robustness to distribution shifts. Existing methods primarily focus on constraining and projecting current model towards the pre-trained initialization based on the magnitudes between fine-tuned and pre-trained weights, which often require extensive hyper-parameter tuning and can sometimes result in underfitting. In this work, we propose $\textbf{Di}$rectional $\textbf{Gra}$dient $\textbf{P}$rojection (DiGraP), a novel layer-wise trainable method that incorporates directional information from gradients to bridge regularization and multi-objective optimization. Besides demonstrating our method on image classification, as another contribution we generalize this area to the multi-modal evaluation settings for robust fine-tuning. Specifically, we first bridge the uni-modal and multi-modal gap by performing analysis on Image Classification reformulated Visual Question Answering (VQA) benchmarks and further categorize ten out-of-distribution (OOD) VQA datasets by distribution shift types and degree (i.e. near versus far OOD). Experimental results show that DiGraP consistently outperforms existing baselines across Image Classfication and VQA tasks with discriminative and generative backbones, improving both in-distribution (ID) generalization and OOD robustness.
Does Spatial Cognition Emerge in Frontier Models?
Santhosh Kumar Ramakrishnan, Erik Wijmans, Philipp Krähenbühl, Vladlen Koltun
Abstract
Not yet. We present SPACE, a benchmark that systematically evaluates spatial cognition in frontier models. Our benchmark builds on decades of research in cognitive science. It evaluates large-scale mapping abilities that are brought to bear when an organism traverses physical environments, smaller-scale reasoning about object shapes and layouts, and cognitive infrastructure such as spatial attention and memory. For many tasks, we instantiate parallel presentations via text and images, allowing us to benchmark both large language models and large multimodal models. Results suggest that contemporary frontier models fall short of the spatial intelligence of animals, performing near chance level on a number of classic tests of animal cognition.
EC-DIT: Scaling Diffusion Transformers with Adaptive Expert-Choice Routing
Haotian Sun, Bowen Zhang, Yanghao Li, Haoshuo Huang, Tao Lei, Ruoming Pang, Bo Dai, Nan Du
Abstract
Diffusion transformers have been widely adopted for text-to-image synthesis. While scaling these models up to billions of parameters shows promise, the effectiveness of scaling beyond current sizes remains underexplored and challenging. By explicitly exploiting the computational heterogeneity of image generations, we develop a new family of Mixture-of-Experts (MoE) models (EC-DIT) for diffusion transformers with expert-choice routing. EC-DIT learns to adaptively optimize the compute allocated to understand the input texts and generate the respective image patches, enabling heterogeneous computation aligned with varying text-image complexities. This heterogeneity provides an efficient way of scaling EC-DIT up to 97 billion parameters and achieving significant improvements in training convergence, text-to-image alignment, and overall generation quality over dense models and conventional MoE models. Through extensive ablations, we show that EC-DIT demonstrates superior scalability and adaptive compute allocation by recognizing varying textual importance through end-to-end training. Notably, in text-to-image alignment evaluation, our largest models achieve a state-of-the-art GenEval score of 71.68% and still maintain competitive inference speed with intuitive interpretability.
EdgeRunner: Auto-regressive Auto-encoder for Artistic Mesh Generation
Jiaxiang Tang, Max Li, Zekun Hao, Xian Liu, Gang Zeng, Ming-Yu Liu, Qinsheng Zhang
Abstract
Current auto-regressive mesh generation methods suffer from issues such as incompleteness, insufficient detail, and poor generalization. In this paper, we propose an Auto-regressive Auto-encoder (ArAE) model capable of generating high-quality 3D meshes with up to 4,000 faces at a spatial resolution of $512^3$.We introduce a novel mesh tokenization algorithm that efficiently compresses triangular meshes into 1D token sequences, significantly enhancing training efficiency. Furthermore, our model compresses variable-length triangular meshes into a fixed-length latent space, enabling training latent diffusion models for better generalization. Extensive experiments demonstrate the superior quality, diversity, and generalization capabilities of our model in both point cloud and image-conditioned mesh generation tasks.
Efficient Evolutionary Search Over Chemical Space with Large Language Models
Haorui Wang, Marta Skreta, Cher Ser, Wenhao Gao, Lingkai Kong, Felix Strieth-Kalthoff, Chenru Duan, Yuchen Zhuang, Yue Yu, Yanqiao Zhu, Yuanqi Du, Alan Aspuru-Guzik, Kirill Neklyudov, Chao Zhang
Abstract
Molecular discovery, when formulated as an optimization problem, presents significant computational challenges because optimization objectives can be non-differentiable. Evolutionary Algorithms (EAs), often used to optimize black-box objectives in molecular discovery, traverse chemical space by performing random mutations and crossovers, leading to a large number of expensive objective evaluations. In this work, we ameliorate this shortcoming by incorporating chemistry-aware Large Language Models (LLMs) into EAs. Namely, we redesign crossover and mutation operations in EAs using LLMs trained on large corpora of chemical information. We perform extensive empirical studies on both commercial and open-source models on multiple tasks involving property optimization, molecular rediscovery, and structure-based drug design, demonstrating that the joint usage of LLMs with EAs yields superior performance over all baseline models across single- and multi-objective settings. We demonstrate that our algorithm improves both the quality of the final solution and convergence speed, thereby reducing the number of required objective evaluations.
EgoSim: Egocentric Exploration in Virtual Worlds with Multi-modal Conditioning
Wei Yu, Songheng Yin, Steve Easterbrook, Animesh Garg
Abstract
Recent advancements in video diffusion models have established a strong foundation for developing world models with practical applications. The next challenge lies in exploring how an agent can leverage these foundation models to understand, interact with, and plan within observed environments. This requires adding more controllability to the model, transforming it into a versatile game engine capable of dynamic manipulation and control. To address this, we investigated three key conditioning factors: camera, context frame, and text, identifying limitations in current model designs. Specifically, the fusion of camera embeddings with video features leads to camera control being influenced by those features. Additionally, while textual information compensates for necessary spatiotemporal structures, it often intrudes into already observed parts of the scene. To tackle these issues, we designed the Spacetime Epipolar Attention Layer, which ensures that egomotion generated by the model strictly aligns with the camera’s movement through rigid constraints. Moreover, we propose the CI2V-adapter, which uses camera information to better determine whether to prioritize textual or visual embeddings, thereby alleviating the issue of textual intrusion into observed areas. Through extensive experiments, we demonstrate that our new model EgoSim achieves excellent results on both the RealEstate and newly repurposed Epic-Field datasets. For more results, please refer to https://egosim.github.io/EgoSim/.
Ensembles of Low-Rank Expert Adapters
Yinghao Li, Vianne Gao, Chao Zhang, MohamadAli Torkamani
Abstract
The training and fine-tuning of large language models (LLMs) often involve diverse textual data from multiple sources, which poses challenges due to conflicting gradient directions, hindering optimization and specialization. These challenges can undermine model generalization across tasks, resulting in reduced downstream performance. Recent research suggests that fine-tuning LLMs on carefully selected, task-specific subsets of data can match or even surpass the performance of using the entire dataset. Building on these insights, we propose the Ensembles of Low-Rank Expert Adapters (ELREA) framework to improve the model’s capability to handle diverse tasks. ELREA clusters the training instructions based on their gradient directions, representing different areas of expertise and thereby reducing conflicts during optimization. Expert adapters are then trained on these clusters, utilizing the low-rank adaptation (LoRA) technique to ensure training efficiency and model scalability. During inference, ELREA combines predictions from the most relevant expert adapters based on the input data’s gradient similarity to the training clusters, ensuring optimal adapter selection for each task. Experiments show that our method outperforms baseline LoRA adapters trained on the full dataset and other ensemble approaches with similar training and inference complexity across a range of domain-specific tasks.
Federated Granger Causality Learning For Interdependent Clients With State Space Representation
Ayush Mohanty, Nazal Mohamed, Paritosh Ramanan, Nagi Gebraeel
Abstract
Advanced sensors and IoT devices have improved the monitoring and control of complex industrial enterprises. They have also created an interdependent fabric of geographically distributed process operations (clients) across these enterprises. Granger causality is an effective approach to detect and quantify interdependencies by examining how the state of one client affects the states of others over time. Understanding these interdependencies helps capture how localized events, such as faults and disruptions, can propagate throughout the system, potentially leading to widespread operational impacts. However, the large volume and complexity of industrial data present significant challenges in effectively modeling these interdependencies. This paper develops a federated approach to learning Granger causality. We utilize a linear state space system framework that leverages low-dimensional state estimates to analyze interdependencies. This helps address bandwidth limitations and the computational burden commonly associated with centralized data processing. We propose augmenting the client models with the Granger causality information learned by the server through a MachineLearning (ML) function. We examine the co-dependence between the augmented client and server models and reformulate the framework as a standalone ML algorithm providing conditions for its sublinear and linear convergence rates. We also study the convergence of the framework to a centralized oracle model. Moreover, we include a differential privacy analysis to ensure data security while preserving causal insights. Using synthetic data, we conduct comprehensive experiments to demonstrate the robustness of our approach to perturbations in causality, the scalability to the size of communication, number of clients, and the dimensions of raw data. We also evaluate the performance on two real-world industrial control system datasets by reporting the volume of data saved by decentralization.
Generalized Behavior Learning from Diverse Demonstrations
Varshith Sreeramdass, Rohan Paleja, Letian Chen, Sanne van Waveren, Matthew Gombolay
Abstract
Diverse behavior policies are valuable in domains requiring quick test-time adaptation or personalized human-robot interaction. Human demonstrations provide rich information regarding task objectives and factors that govern individual behavior variations, which can be used to characterize \it{useful} diversity and learn diverse performant policies.However, we show that prior work that builds naive representations of demonstration heterogeneity fails in generating successful novel behaviors that generalize over behavior factors.We propose Guided Strategy Discovery (GSD), which introduces a novel diversity formulation based on a learned task-relevance measure that prioritizes behaviors exploring modeled latent factors.We empirically validate across three continuous control benchmarks for generalizing to in-distribution (interpolation) and out-of-distribution (extrapolation) factors that GSD outperforms baselines in novel behavior discovery by $\sim$21\%.Finally, we demonstrate that GSD can generalize striking behaviors for table tennis in a virtual testbed while leveraging human demonstrations collected in the real world.
Generating CAD Code with Vision-Language Models for 3D Designs
Kamel Alrashedy, Pradyumna Tambwekar, Zulfiqar Haider Zaidi, Megan Langwasser, Wei Xu, Matthew Gombolay
Abstract
Generative AI has transformed the fields of Design and Manufacturing by providing efficient and automated methods for generating and modifying 3D objects. One approach involves using Large Language Models (LLMs) to generate Computer-Aided Design (CAD) scripting code, which can then be executed to render a 3Dobject; however, the resulting 3D object may not meet the specified requirements. Testing the correctness of CAD generated code is challenging due to the complexity and structure of 3D objects (e.g., shapes, surfaces, and dimensions) that are not feasible in code. In this paper, we introduce CADCodeVerify, a novel approach toiteratively verify and improve 3D objects generated from CAD code. Our approach works by producing ameliorative feedback by prompting a Vision-Language Model(VLM) to generate and answer a set of validation questions to verify the generated object and prompt the VLM to correct deviations. To evaluate CADCodeVerify, we introduce, CADPrompt, the first benchmark for CAD code generation, consisting of200 natural language prompts paired with expert-annotated scripting code for 3Dobjects to benchmark progress. Our findings show that CADCodeVerify improvesVLM performance by providing visual feedback, enhancing the structure of the 3Dobjects, and increasing the success rate of the compiled program. When applied toGPT-4, CADCodeVerify achieved a 7.30% reduction in Point Cloud distance and a5.0% improvement in success rate compared to prior work.
Group Ligands Docking to Protein Pockets
Jiaqi Guan, Jiahan Li, Xiangxin Zhou, Xingang Peng, Sheng Wang, Yunan Luo, Jian Peng, Jianzhu Ma
Abstract
Molecular docking is a key task in computational biology that has attracted increasing interest from the machine learning community. While existing methods have achieved success, they generally treat each protein-ligand pair in isolation. Inspired by the biochemical observation that ligands binding to the same target protein tend to adopt similar poses, we propose \textsc{GroupBind}, a novel molecular docking framework that simultaneously considers multiple ligands docking to a protein. This is achieved by introducing an interaction layer for the group of ligands and a triangle attention module for embedding protein-ligand and group-ligand pairs. By integrating our approach with diffusion based docking model, we set a new state-of-the-art performance on the PDBBind blind docking benchmark, demonstrating the effectiveness of our paradigm in enhancing molecular docking accuracy.
Has the Deep Neural Network learned the Stochastic Process? An Evaluation Viewpoint
Harshit Kumar, Beomseok Kang, Biswadeep Chakraborty, Saibal Mukhopadhyay
Abstract
This paper presents the first systematic study of evaluating Deep Neural Networks (DNNs) designed to forecast the evolution of stochastic complex systems. We show that traditional evaluation methods like threshold-based classification metrics and error-based scoring rules assess a DNN’s ability to replicate the observed ground truth but fail to measure the DNN’s learning of the underlying stochastic process. To address this gap, we propose a new evaluation criteria called _Fidelity to Stochastic Process (F2SP)_, representing the DNN’s ability to predict the system property _Statistic-GT_—the ground truth of the stochastic process—and introduce an evaluation metric that exclusively assesses F2SP. We formalize F2SP within a stochastic framework and establish criteria for validly measuring it. We formally show that Expected Calibration Error (ECE) satisfies the necessary condition for testing F2SP, unlike traditional evaluation methods. Empirical experiments on synthetic datasets, including wildfire, host-pathogen, and stock market models, demonstrate that ECE uniquely captures F2SP. We further extend our study to real-world wildfire data, highlighting the limitations of conventional evaluation and discuss the practical utility of incorporating F2SP into model assessment. This work offers a new perspective on evaluating DNNs modeling complex systems by emphasizing the importance of capturing underlying the stochastic process.
HelpSteer2-Preference: Complementing Ratings with Preferences
Zhilin Wang, Alexander Bukharin, Olivier Delalleau, Daniel Egert, Gerald Shen, Jiaqi Zeng, Oleksii Kuchaiev, Yi Dong
Abstract
Reward models are critical for aligning models to follow instructions, and are typically trained following one of two popular paradigms: Bradley-Terry style or Regression style. However, there is a lack of evidence that either approach is better than the other, when adequately matched for data. This is primarily because these approaches require data collected in different (but incompatible) formats, meaning that adequately matched data is not available in existing public datasets. To tackle this problem, we release preference annotations (designed for Bradley-Terry training) to complement existing ratings (designed for Regression style training) in the HelpSteer2 dataset. To improve data interpretability, preference annotations are accompanied with human-written justifications. Using this data, we conduct the first head-to-head comparison of Bradley-Terry and Regression models when adequately matched for data. Based on insights derived from such a comparison, we propose a novel approach to combine Bradley-Terry and Regression reward modeling. A Llama-3.1-70B-Instruct model tuned with this approach scores 94.1 on RewardBench, emerging top of more than 140 reward models as of 1 Oct 2024. This reward model can then be used with REINFORCE to align a model to reach 85.0 on Arena Hard, which is No. 1 as of 1 Oct 2024.We open-source this dataset (CC-BY-4.0 license) and openly release the trained reward and aligned models.
High-Quality Joint Image and Video Compression with Causal VAE
Dawit Mureja Argaw, Xian Liu, Qinsheng Zhang, Joon Son Chung, Ming-Yu Liu, Fitsum Reda
Abstract
Generative modeling has seen significant advancements in image and video synthesis. However, the curse of dimensionality remains a significant obstacle, especially for video generation, given its inherently complex and high-dimensional nature. Many existing works rely on low-dimensional latent spaces from pretrained image autoencoders. However, this approach overlooks temporal redundancy in videos and often leads to temporally incoherent decoding. To address this issue, we propose a video compression network that reduces the dimensionality of visual data both spatially and temporally. Our model, based on a variational autoencoder, employs causal 3D convolution to handle images and videos jointly. The key contributions of our work include a scale-agnostic encoder for preserving video fidelity, a novel spatio-temporal down/upsampling block for robust long-sequence modeling, and a flow regularization loss for accurate motion decoding. Our approach outperforms competitors in video quality and compression rates across various datasets. Experimental analyses also highlight its potential as a robust autoencoder for video generation training. Code and models will be open-sourced.
HyperDAS: Towards Automating Mechanistic Interpretability with Hypernetworks
Jiuding Sun, Jing Huang, Sidharth Baskaran, Karel D’Oosterlinck, Christopher Potts, Michael Sklar, Atticus Geiger
Abstract
Mechanistic interpretability has made great strides in identifying neural network features (e.g., directions in hidden activation space) that mediate concepts (e.g., *the birth year of a Nobel laureate*) and enable predictable manipulation. Distributed alignment search (DAS) leverages supervision from counterfactual data to learn concept features within hidden states, but DAS assumes we can afford to conduct a brute force search over potential feature locations. To address this, we present HyperDAS, a transformer-based hypernetwork architecture that (1) automatically locates the token-positions of the residual stream that a concept is realized in and (2) learns features of those residual stream vectors for the concept. In experiments with Llama3-8B, HyperDAS achieves state-of-the-art performance on the RAVEL benchmark for disentangling concepts in hidden states. In addition, we review the design decisions we made to mitigate the concern that HyperDAS (like all powerful interpretabilty methods) might inject new information into the target model rather than faithfully interpreting it.
ImProver: Agent-Based Automated Proof Optimization
Riyaz Ahuja, Jeremy Avigad, Prasad Tetali, Sean Welleck
Abstract
Large language models (LLMs) have been used to generate formal proofs of mathematical theorems in proofs assistants such as Lean.However, we often want to optimize a formal proof with respect to various criteria, depending on its downstream use.For example, we may want a proof to adhere to a certain style, be declaratively structured, or concise. Having suitably optimized proofs is also important for learning tasks, especially since human-written proofs may not optimal for that purpose.To this end, we study a new problem of automated proof optimization: rewriting a proof so that it is correct and optimizes for an arbitrary criterion, such as length or declarativity.As a first method for automated proof optimization, we present ImProver, a large-language-model agent that rewrites proofs to optimize arbitrary user-defined metrics in Lean.We find that naively applying LLMs to proof optimization falls short, and we incorporate various improvements into ImProver, such as the use of symbolic Lean context in a novel Chain-of-States technique, as well as error-correction and retrieval. We test ImProver on rewriting real-world undergraduate, competition, and research-level mathematics theorems, finding that ImProver is capable of rewriting proofs so that they are substantially shorter and more declarative in structure.
Improving Neural Optimal Transport via Displacement Interpolation
Jaemoo Choi, Yongxin Chen, Jaewoong Choi
Abstract
Optimal Transport (OT) theory investigates the cost-minimizing transport map that moves a source distribution to a target distribution. Recently, several approaches have emerged for learning the optimal transport map for a given cost function using neural networks. We refer to these approaches as the OT Map. OT Map provides a powerful tool for diverse machine learning tasks, such as generative modeling and unpaired image-to-image translation. However, existing methods that utilize max-min optimization often experience training instability and sensitivity to hyperparameters. In this paper, we propose a novel method to improve stability and achieve a better approximation of the OT Map by exploiting displacement interpolation, dubbed Displacement Interpolation Optimal Transport Model (DIOTM). We derive the dual formulation of displacement interpolation at specific time $t$ and prove how these dual problems are related across time. This result allows us to utilize the entire trajectory of displacement interpolation in learning the OT Map. Our method improves the training stability and achieves superior results in estimating optimal transport maps. We demonstrate that DIOTM outperforms existing OT-based models on image-to-image translation tasks.
In-context Time Series Predictor
Jiecheng Lu, Yan Sun, Shihao Yang
Abstract
Recent Transformer-based large language models (LLMs) demonstrate in-context learning ability to perform various functions based solely on the provided context, without updating model parameters. To fully utilize the in-context capabilities in time series forecasting (TSF) problems, unlike previous Transformer-based or LLM-based time series forecasting methods, we reformulate “time series forecasting tasks” as input tokens by constructing a series of (lookback, future) pairs within the tokens. This method aligns more closely with the inherent in-context mechanisms and is more parameter-efficient without the need of using pre-trained LLM parameters. Furthermore, it addresses issues such as overfitting in existing Transformer-based TSF models, consistently achieving better performance across full-data, few-shot, and zero-shot settings compared to previous architectures.
Kernel-based Optimally Weighted Conformal Time-Series Prediction
Jonghyeok Lee, Chen Xu, Yao Xie
Abstract
Conformal prediction has been a popular distribution-free framework for uncertainty quantification. In this work, we present a novel conformal prediction method for time-series, which we call Kernel-based Optimally Weighted Conformal Prediction Intervals ($\texttt{KOWCPI}$). Specifically, $\texttt{KOWCPI}$ adapts the classic Reweighted Nadaraya-Watson (RNW) estimator for quantile regression on dependent data and learns optimal data-adaptive weights. Theoretically, we tackle the challenge of establishing a conditional coverage guarantee for non-exchangeable data under strong mixing conditions on the non-conformity scores. We demonstrate the superior performance of $\texttt{KOWCPI}$ on real time-series against state-of-the-art methods, where $\texttt{KOWCPI}$ achieves narrower confidence intervals without losing coverage.
Language Guided Skill Discovery
Seungeun Rho, Laura Smith, Tianyu Li, Sergey Levine, Xue Bin Peng, Sehoon Ha
Abstract
Skill discovery methods enable agents to learn diverse emergent behaviors without explicit rewards. To make learned skills useful for downstream tasks, obtaining a semantically diverse repertoire of skills is crucial. While some approaches use discriminators to acquire distinguishable skills and others focus on increasing state coverage, the direct pursuit of ‘semantic diversity’ in skills remains underexplored. We hypothesize that leveraging the semantic knowledge of large language models (LLM) can lead us to improve semantic diversity of resulting behaviors. In this sense, we introduce Language Guided Skill Discovery (LGSD), a skill discovery framework that aims to directly maximize the semantic diversity between skills. LGSD takes user prompts as input and outputs a set of semantically distinctive skills. The prompts serve as a means to constrain the search space into a semantically desired subspace, and the generated LLM outputs guide the agent to visit semantically diverse states within the subspace. We demonstrate that LGSD enables legged robots to visit different user-intended areas on a plane by simply changing the prompt. Furthermore, we show that language guidance aids in discovering more diverse skills compared to five existing skill discovery methods in robot-arm manipulation environments. Lastly, LGSD provides a simple way of utilizing learned skills via natural language.
Phillip Si, Peng Chen
Abstract
Accurate modeling and prediction of complex physical systems often rely on data assimilation techniques to correct errors inherent in model simulations. Traditional methods like the Ensemble Kalman Filter (EnKF) and its variants as well as the recently developed Ensemble Score Filters (EnSF) face significant challenges when dealing with high-dimensional and nonlinear Bayesian filtering problems with sparse observations, which are ubiquitous in real-world applications. In this paper, we propose a novel data assimilation method, Latent-EnSF, which leverages EnSF with efficient and consistent latent representations of the full states and sparse observations to address the joint challenges of high dimensionlity in states and high sparsity in observations for nonlinear Bayesian filtering. We introduce a coupled Variational Autoencoder (VAE) with two encoders to encode the full states and sparse observations in a consistent way guaranteed by a latent distribution matching and regularization as well as a consistent state reconstruction. With comparison to several methods, we demonstrate the higher accuracy, faster convergence, and higher efficiency of Latent-EnSF for two challenging applications with complex models in shallow water wave propagation and medium-range weather forecasting, for highly sparse observations in both space and time.
LongMamba: Enhancing Mamba’s Long-Context Capabilities via Training-Free Receptive Field Enlargement
Zhifan Ye, Kejing Xia, Yonggan Fu, Xin Dong, Jihoon Hong, Xiangchi Yuan, Shizhe Diao, Jan Kautz, Pavlo Molchanov, Yingyan Celine Lin
Abstract
Mamba models have emerged as an efficient alternative to Transformer models for language modeling tasks, offering linear complexity as context length increases. However, despite their efficiency in handling long contexts, recent studies have demonstrated that Mamba models underperform in understanding extended contexts compared to Transformer models. To address this significant shortfall, we propose “LongMamba”, a training-free technique that significantly enhances the long-context capabilities of Mamba models. Our approach builds upon the discovery that hidden state channels in Mamba models—categorized into \textit{local} and \textit{global channels} based on their receptive field lengths—exhibit distinct functionalities. Specifically, the \textit{global channels} struggle to adaptively extend their effective receptive fields when input lengths far exceed their training sequence length due to exponential decay in their hidden states. We hypothesize this exponential decay is the root cause of Mamba models’ limited performance in extended contexts. LongMamba counters this by effectively expanding the \textit{global channels}’ receptive fields to fully encompass the input sequence length, thus enabling them to capture global information more effectively. Through extensive benchmarking across synthetic and real-world long-context scenarios, LongMamba sets a new standard for state-of-the-art performance in Mamba-based long-context tasks, significantly extending the operational range of Mamba models without requiring additional fine-tuning. All code and models will be released upon acceptance.
Kaiwen Zheng, Yongxin Chen, Hanzi Mao, Ming-Yu Liu, Jun Zhu, Qinsheng Zhang
Abstract
Masked diffusion models (MDMs) have emerged as a popular research topic for generative modeling of discrete data, thanks to their superior performance over other discrete diffusion models, and are rivaling the auto-regressive models (ARMs) for language modeling tasks. The recent effort in simplifying the masked diffusion framework further leads to alignment with continuous-space diffusion models and more principled training and sampling recipes. In this paper, however, we reveal that both training and sampling of MDMs are theoretically free from the time variable, arguably the key signature of diffusion models, and are instead equivalent to masked models. The connection on the sampling aspect is drawn by our proposed first-hitting sampler (FHS). Specifically, we show that the FHS is theoretically equivalent to MDMs’ original generation process while significantly alleviating the time-consuming categorical sampling and achieving a 20$\times$ speedup. In addition, our investigation raises doubts about whether MDMs can truly beat ARMs in text generation. We identify, for the first time, an underlying numerical issue, even with the commonly used 32-bit floating-point precision, which results in inaccurate categorical sampling. We show that it lowers the effective temperature both theoretically and empirically, and the resulting decrease in token diversity makes previous evaluations, which assess the generation quality solely through the incomplete generative perplexity metric, somewhat unfair.
Model merging with SVD to tie the Knots
George Stoica, Pratik Ramesh, Boglarka Ecsedi, Leshem Choshen, Judy Hoffman
Abstract
Recent model merging methods demonstrate that the parameters of fully-finetuned models specializing in distinct tasks can be combined into one model capable of solving all tasks without retraining. Yet, this success does not transfer well when merging LoRA finetuned models. We study this phenomenon and observe that the weights of LoRA finetuned models showcase a lower degree of alignment compared to their fully-finetuned counterparts. We hypothesize that improving this alignment is key to obtaining better LoRA model merges, and propose KnOTS to address this problem. KnOTS uses the SVD to jointly transform the weights of different LoRA models into an aligned space, where existing merging methods can be applied. In addition, we introduce a new benchmark that explicitly evaluates whether merged models are general models. Notably, KnOTS consistently improves LoRA merging by up to 4.3% across several vision and language benchmarks, including our new setting.
On Statistical Rates of Conditional Diffusion Transformer: Approximation and Estimation
Jerry Hu, Weimin Wu, Yi-Chen Lee, Yu-Chao Huang, Minshuo Chen, Han Liu
Abstract
We investigate the approximation and estimation rates of conditional diffusion transformers (DiTs) with classifier-free guidance.We present a comprehensive analysis for “in-context” conditional DiTs under four common data assumptions. We show that both conditional DiTs and their latent variants lead to the minimax optimality of unconditional DiTs under identified settings. Specifically, we discretize the input domains into infinitesimal grids and then perform a term-by-term Taylor expansion on the conditional diffusion score function under Hölder smooth data assumption. This enables fine-grained use of transformers’ universal approximation through a more detailed piecewise constant approximation, and hence obtains tighter bounds.Additionally, we extend our analysis to the latent setting under the linear latent subspace assumption.We not only show that latent conditional DiTs achieve lower bounds than conditional DiTs both in approximation and estimation, but also show the minimax optimality of latent unconditional DiTs.Our findings establish statistical limits for conditional and unconditional DiTs, and offer practical guidance toward developing more efficient and accurate DiT models.
PARTNR: A Benchmark for Planning and Reasoning in Embodied Multi-agent Tasks
Matthew Chang, Gunjan Chhablani, Alexander Clegg, Mikael Dallaire Cote, Ruta Desai, Michal Hlavac, Vladimir Karashchuk, Jacob Krantz, Roozbeh Mottaghi, Priyam Parashar, Siddharth Patki, Ishita Prasad, Xavier Puig, Akshara Rai, Ram Ramrakhya, Daniel Tran, Joanne Truong, John Turner, Eric Undersander, Tsung-Yen Yang
Abstract
We present a benchmark for Planning And Reasoning Tasks in humaN-Robot collaboration (PARTNR) designed to study human-robot coordination in household activities. PARTNR tasks exhibit characteristics of everyday tasks, such as spatial, temporal, and heterogeneous agent capability constraints. We employ a semi-automated task generation pipeline using Large Language Models (LLMs), incorporating simulation-in-the-loop for the grounding and verification. PARTNR stands as the largest benchmark of its kind, comprising 100,000 natural language tasks, spanning 60 houses and 5,819 unique objects. We analyze state-of-the-art LLMs on PARTNR tasks, across the axes of planning, perception and skill execution. The analysis reveals significant limitations in SoTA models, such as poor coordination and failures in task tracking and recovery from errors. When LLMs are paired with ‘real’ humans, they require 1.5x as many steps as two humans collaborating and 1.1x more steps than a single human, underscoring the potential for improvement in these models. We further show that fine-tuning smaller LLMs with planning data can achieve performance on par with models 9 times larger, while being 8.6x faster at inference. Overall, PARTNR highlights significant challenges facing collaborative embodied agents and aims to drive research in this direction.
Provable Benefit of Annealed Langevin Monte Carlo for Non-log-concave Sampling
Wei Guo, Molei Tao, Yongxin Chen
Abstract
We consider the outstanding problem of sampling from an unnormalized density that may be non-log-concave and multimodal. To enhance the performance of simple Markov chain Monte Carlo (MCMC) methods, techniques of annealing type have been widely used. However, quantitative theoretical guarantees of these techniques are under-explored. This study takes a first step toward providing a non-asymptotic analysis of annealed MCMC. Specifically, we establish, for the first time, an oracle complexity of $\widetilde{O}\left(\frac{d\beta^2{\cal A}^2}{\varepsilon^6}\right)$ for the simple annealed Langevin Monte Carlo algorithm to achieve $\varepsilon^2$ accuracy in Kullback-Leibler divergence to the target distribution $\pi\propto{\rm e}^{-V}$ on $\mathbb{R}^d$ with $\beta$-smooth potential $V$. Here, ${\cal A}$ represents the action of a curve of probability measures interpolating the target distribution $\pi$ and a readily sampleable distribution.
PWM: Policy Learning with Multi-Task World Models
Ignat Georgiev, Varun Giridhar, Nicklas Hansen, Animesh Garg
Abstract
Reinforcement Learning (RL) has made significant strides in complex tasks but struggles in multi-task settings with different embodiments. World models methods offer scalability by learning a simulation of the environment, but often rely on inefficient gradient-free optimization methods for policy extraction. In contrast, gradient-based methods exhibit lower variance but fail to handle discontinuities. Our work reveals that well-regularized world models can generate smoother optimization landscapes than the actual dynamics, facilitating more effective first-order optimization. We introduce Policy learning with multi-task World Models (PWM), a novel model-based RL algorithm for continuous control. Initially, the world model is pre-trained on offline data, and then policies are extracted from it using first-order optimization in less than 10 minutes per task. PWM effectively solves tasks with up to 152 action dimensions and outperforms methods that use ground-truth dynamics. Additionally, PWM scales to an 80-task setting, achieving up to 27% higher rewards than existing baselines, without relying on costly online planning. Visualizations and code available at [https://policy-world-model.github.io/](https://policy-world-model.github.io/).
Mufei Li, Siqi Miao, Pan Li
Abstract
Large Language Models (LLMs) demonstrate strong reasoning abilities but face limitations such as hallucinations and outdated knowledge. Knowledge Graph (KG)-based Retrieval-Augmented Generation (RAG) addresses these issues by grounding LLM outputs in structured external knowledge from KGs. However, current KG-based RAG frameworks still struggle to optimize the trade-off between retrieval accuracy and efficiency in identifying a suitable amount of relevant graph information for the LLM to digest. We introduce SubgraphRAG, extending the KG-based RAG framework that retrieves subgraphs centered on query/topic entities and leverages LLMs for reasoning. Our approach innovatively integrates a lightweight multilayer perceptron (MLP) with a parallel triple-scoring mechanism for efficient subgraph retrieval while encoding directional structural distances to enhance retrieval accuracy. The size of retrieved subgraphs can be flexibly adjusted to match the query’s need and the downstream LLM’s reasoning capacity. This design strikes a balance between model complexity and reasoning power, enabling scalable and generalizable retrieval processes. Notably, based on our retrieved subgraphs, smaller models like Llama3.1-8B deliver competitive results with explainable reasoning, while larger models like GPT-4o achieve comparable or better state-of-the-art accuracy compared with previous baselines—all without fine-tuning. Extensive evaluations on the WebQSP and CWQ benchmarks highlight SubgraphRAG’s strengths in efficiency, accuracy, and reliability by reducing hallucinations and improving response grounding.
Robust Barycenter Estimation using Semi-Unbalanced Neural Optimal Transport
Milena Gazdieva, Jaemoo Choi, Alexander Kolesov, Jaewoong Choi, Petr Mokrov, Alexander Korotin
Abstract
Aggregating data from multiple sources can be formalized as an *Optimal Transport* (OT) barycenter problem, which seeks to compute the average of probability distributions with respect to OT discrepancies. However, in real-world scenarios, the presence of outliers and noise in the data measures can significantly hinder the performance of traditional statistical methods for estimating OT barycenters. To address this issue, we propose a novel, scalable approach for estimating the *robust* continuous barycenter, leveraging the dual formulation of the *(semi-)unbalanced* OT problem. To the best of our knowledge, this paper is the first attempt to develop an algorithm for robust barycenters under the continuous distribution setup. Our method is framed as a $\min$-$\max$ optimization problem and is adaptable to *general* cost function. We rigorously establish the theoretical underpinnings of the proposed method and demonstrate its robustness to outliers and class imbalance through a number of illustrative experiments.
Scalable Discrete Diffusion Samplers: Combinatorial Optimization and Statistical Physics
Sebastian Sanokowski, Wilhelm Berghammer, Haoyu Wang, Martin Ennemoser, Sepp Hochreiter, Sebastian Lehner
Abstract
Learning to sample from complex unnormalized distributions over discrete domains emerged as a promising research direction with applications in statistical physics, variational inference, and combinatorial optimization. Recent work has demonstrated the potential of diffusion models in this domain. However, existing methods face limitations in memory scaling and thus the number of attainable diffusion steps since they require backpropagation through the entire generative process. To overcome these limitations we introduce two novel training methods for discrete diffusion samplers, one grounded in the policy gradient theorem and the other one leveraging Self-Normalized Neural Importance Sampling (SN-NIS). These methods yield memory-efficient training and achieve state-of-the-art results in unsupervised combinatorial optimization.Numerous scientific applications additionally require the ability of unbiased sampling. We introduce adaptations of SN-NIS and Neural Markov Chain Monte Carlo that enable for the first time the application of discrete diffusion models to this problem. We validate our methods on Ising model benchmarks and find that they outperform popular autoregressive approaches. Our work opens new avenues for applying diffusion models to a wide range of scientific applications in discrete domains that were hitherto restricted to exact likelihood models.
Self-MoE: Towards Compositional Large Language Models with Self-Specialized Experts
Junmo Kang, Leonid Karlinsky, Hongyin Luo, Zhen Wang, Jacob Hansen, James R Glass, David Cox, Rameswar Panda, Rogerio Feris, Alan Ritter
Abstract
We present Self-MoE, an approach that transforms a monolithic LLM into a compositional, modular system of self-specialized experts, named MiXSE (MiXture of Self-specialized Experts). Our approach leverages self-specialization, which constructs expert modules using self-generated synthetic data, each equipping a shared base LLM with distinct domain-specific capabilities, activated via self-optimized routing. This allows for dynamic and capability-specific handling of various target tasks, enhancing overall capabilities, without extensive human-labeled data and added parameters. Our empirical results reveal that specializing LLMs may exhibit potential trade-offs in performances on non-specialized tasks. On the other hand, our Self-MoE demonstrates substantial improvements (6.5%p on average) over the base LLM across diverse benchmarks such as knowledge, reasoning, math, and coding. It also consistently outperforms other methods, including instance merging and weight merging, while offering better flexibility and interpretability by design with semantic experts and routing. Our findings highlight the critical role of modularity, the applicability of Self-MoE to multiple base LLMs, and the potential of self-improvement in achieving efficient, scalable, and adaptable systems.
Size-Generalizable RNA Structure Evaluation by Exploring Hierarchical Geometries
Zongzhao Li, Jiacheng Cen, Wenbing Huang, Taifeng Wang, Le Song
Abstract
Understanding the 3D structure of RNA is essential for deciphering its function and developing RNA-based therapeutics. Geometric Graph Neural Networks (GeoGNNs) that conform to the $\mathrm{E}(3)$-symmetry have advanced RNA structure evaluation, a crucial step toward RNA structure prediction. However, existing GeoGNNs are still defective in two aspects: 1. inefficient or incapable of capturing the full geometries of RNA; 2. limited generalization ability when the size of RNA significantly differs between training and test datasets. In this paper, we propose EquiRNA, a novel equivariant GNN model by exploring the three-level hierarchical geometries of RNA. At its core, EquiRNA effectively addresses the size generalization challenge by reusing the representation of nucleotide, the common building block shared across RNAs of varying sizes. Moreover, by adopting a scalarization-based equivariant GNN as the backbone, our model maintains directional information while offering higher computational efficiency compared to existing GeoGNNs. Additionally, we propose a size-insensitive $K$-nearest neighbor sampling strategy to enhance the model’s robustness to RNA size shifts. We test our approach on our created benchmark as well as an existing dataset. The results show that our method significantly outperforms other state-of-the-art methods, providing a robust baseline for RNA 3D structure modeling and evaluation.
STORM: Spatio-TempOral Reconstruction Model For Large-Scale Outdoor Scenes
Jiawei Yang, Jiahui Huang, Boris Ivanovic, Yuxiao Chen, Yan Wang, Boyi Li, Yurong You, Apoorva Sharma, Maximilian Igl, Peter Karkus, Danfei Xu, Yue Wang, Marco Pavone
Abstract
We present STORM, a spatio-temporal reconstruction model designed to reconstruct in-the-wild dynamic outdoor scenes from sparse observations. Existing dynamic reconstruction methods rely heavily on dense observations across space and time and strong motion supervision, therefore suffering from lengthy optimization time, limited generalizability to novel views or scenes, and degenerated quality caused by noisy pseudo-labels. To bridge the gap, STORM introduces a data-driven Transformer architecture that jointly infers 3D scenes and their dynamics in a single forward pass. A key design of our scene representation is to aggregate 3D Gaussians and their motion predicted from all frames, which are later transformed to the target timestep for a more complete (i.e. “amodal”) reconstruction at any given time from any viewpoint. As an emergent property, STORM can automatically capture dynamic instances and their high-quality masks using just the reconstruction loss. Extensive experiments show that STORM accurately reconstructs dynamic scenes and outperforms other per-scene optimization (+3.7 PSNR) or feed-forward approaches (+1.5 PSNR), it can reconstruct large-scale outdoor scenes within just 200ms and render in real-time. Beyond reconstruction, we qualitatively demonstrate four additional applications of our model, demonstrating the potential of self-supervised learning for advancing dynamic scene understanding. Our code and model will be released.
Kangrui Du, Yuhang Wu, Shikuang Deng, Shi Gu
Abstract
Spiking Neural Networks (SNNs), models inspired by neural mechanisms in the brain, allow for energy-efficient implementation on neuromorphic hardware. However, SNNs trained with current direct training approaches are constrained to a specific time step. This “temporal inflexibility” 1) hinders SNNs’ deployment on time-step-free fully event-driven chips and 2) prevents energy-performance balance based on dynamic inference time steps. In this study, we first explore the feasibility of training SNNs that generalize across different time steps. We then introduce Mixed Time-step Training (MTT), a novel method that improves the temporal flexibility of SNNs, making SNNs adaptive to diverse temporal structures. During each iteration of MTT, random time steps are assigned to different SNN stages, with spikes transmitted between stages via communication modules. After training, the weights are deployed and evaluated on both time-stepped and fully event-driven platforms. Experimental results show that models trained by MTT gain remarkable temporal flexibility, friendliness for both event-driven and clock-driven deployment (nearly lossless on N-MNIST and 10.1\% higher than standard methods on CIFAR10-DVS), enhanced network generalization, and near SOTA performance. To the best of our knowledge, this is the first work to report the results of large-scale SNN deployment on fully event-driven scenarios.
Towards Domain Adaptive Neural Contextual Bandits
Ziyan Wang, Xiaoming Huo, Hao Wang
Abstract
Contextual bandit algorithms are essential for solving real-world decision making problems. In practice, collecting a contextual bandit’s feedback from different domains may involve different costs. For example, measuring drug reaction from mice (as a source domain) and humans (as a target domain). Unfortunately, adapting a contextual bandit algorithm from a source domain to a target domain with distribution shift still remains a major challenge and largely unexplored. In this paper, we introduce the first general domain adaptation method for contextual bandits. Our approach learns a bandit model for the target domain by collecting feedback from the source domain. Our theoretical analysis shows that our algorithm maintains a sub-linear regret bound even adapting across domains. Empirical results show that our approach outperforms the state-of-the-art contextual bandit algorithms on real-world datasets.
Tengfei Ma, Xiang song, Wen Tao, Mufei Li, Jiani Zhang, Xiaoqin Pan, Yijun Wang, Bosheng Song, Xiangxiang Zeng
Abstract
Knowledge graph completion (KGC) aims to alleviate the inherent incompleteness of knowledge graphs (KGs), a crucial task for numerous applications such as recommendation systems and drug repurposing. The success of knowledge graph embedding (KGE) models provokes the question about the explainability: “\textit{Which the patterns of the input KG are most determinant to the prediction}?” Particularly, path-based explainers prevail in existing methods because of their strong capability for human understanding. In this paper, based on the observation that a fact is usually determined by the synergy of multiple reasoning chains, we propose a novel explainable framework, dubbed KGExplainer, to explore synergistic pathways. KGExplainer is a model-agnostic approach that employs a perturbation-based greedy search algorithm to identify the most crucial synergistic paths as explanations within the local structure of target predictions. To evaluate the quality of these explanations, KGExplainer distills an evaluator from the target KGE model, allowing for the examination of their fidelity. We experimentally demonstrate that the distilled evaluator has comparable predictive performance to the target KGE. Experimental results on benchmark datasets demonstrate the effectiveness of KGExplainer, achieving a human evaluation accuracy of 83.3\% and showing promising improvements in explainability. Code is available at \url{https://anonymous.4open.science/r/KGExplainer-33A0}
Training-free LLM-generated Text Detection by Mining Token Probability Sequences
Yihuai Xu, Yongwei Wang, YIFEI BI, Huangsen Cao, Zhouhan Lin, Yu Zhao, Fei Wu
Abstract
Large language models (LLMs) have demonstrated remarkable capabilities in generating high-quality texts across diverse domains. However, the potential misuse of LLMs has raised significant concerns, underscoring the urgent need for reliable detection of LLM-generated texts. Conventional training-based detectors often struggle with generalization, particularly in cross-domain and cross-model scenarios. In contrast, training-free methods, which focus on inherent discrepancies through carefully designed statistical features, offer improved generalization and interpretability. Despite this, existing training-free detection methods typically rely on global text sequence statistics, neglecting the modeling of local discriminative features, thereby limiting their detection efficacy. In this work, we introduce a novel training-free detector, termed \textbf{Lastde} that synergizes local and global statistics for enhanced detection. For the first time, we introduce time series analysis to LLM-generated text detection, capturing the temporal dynamics of token probability sequences. By integrating these local statistics with global ones, our detector reveals significant disparities between human and LLM-generated texts. We also propose an efficient alternative, \textbf{Lastde++} to enable real-time detection. Extensive experiments on six datasets involving cross-domain, cross-model, and cross-lingual detection scenarios, under both white-box and black-box settings, demonstrated that our method consistently achieves state-of-the-art performance. Furthermore, our approach exhibits greater robustness against paraphrasing attacks compared to existing baseline methods. {Our codes are available at \url{https://anonymous.4open.science/r/Lastde-5DBC} anonymously}.
Trivialized Momentum Facilitates Diffusion Generative Modeling on Lie Groups
Yuchen Zhu, Tianrong Chen, Lingkai Kong, Evangelos Theodorou, Molei Tao
Abstract
The generative modeling of data on manifold is an important task, for which diffusion models in flat spaces typically need nontrivial adaptations. This article demonstrates how a technique called `trivialization’ can transfer the effectiveness of diffusion models in Euclidean spaces to Lie groups. In particular, an auxiliary momentum variable was algorithmically introduced to help transport the position variable between data distribution and a fixed, easy-to-sample distribution. Normally, this would incur further difficulty for manifold data because momentum lives in a space that changes with the position. However, our trivialization technique creates a new momentum variable that stays in a simple $\textbf{fixed vector space}$. This design, together with a manifold preserving integrator, simplifies implementation and avoids inaccuracies created by approximations such as projections to tangent space and manifold, which were typically used in prior work, hence facilitating generation with high-fidelity and efficiency. The resulting method achieves state-of-the-art performance on protein and RNA torsion angle generation and sophisticated torus datasets. We also, arguably for the first time, tackle the generation of data on high-dimensional Special Orthogonal and Unitary groups, the latter essential for quantum problems.
Understanding Bottlenecks of State Space Models through the Lens of Recency and Over-smoothing
Peihao Wang, Ruisi Cai, Yuehao Wang, Jiajun Zhu, Pragya Srivastava, Zhangyang Wang, Pan Li
Abstract
Structured State Space Models (SSMs) have emerged as alternatives to transformers, addressing the challenges of processing long sequences.While SSMs are often regarded as effective in capturing long-term dependencies, we theoretically demonstrate that they suffer from a strong recency bias.Our empirical findings reveal that this bias impairs the models’ ability to recall distant information and introduces robustness issues.We conducted scaling experiments and discovered that deeper structures in SSMs facilitate the learning of long contexts.However, our theoretical analysis reveal that as SSMs increase in depth, they exhibit a tendency toward over-smoothing, resulting in token representations becoming increasingly indistinguishable.This over-smoothing phenomenon ultimately constrains the scalability of SSMs to achieve improved performance.Collectively, these findings highlight important limitations of SSMs and underscore the need for further research to address these challenges in long-range sequence modeling.
What Are Good Positional Encodings for Directed Graphs?
Yinan Huang, Haoyu Wang, Pan Li
Abstract
Positional encodings (PEs) are essential for building powerful and expressive graph neural networks and graph transformers, as they effectively capture the relative spatial relationships between nodes. Although extensive research has been devoted to PEs in undirected graphs, PEs for directed graphs remain relatively unexplored. This work seeks to address this gap. We first introduce the notion of *Walk Profile*, a generalization of walk-counting sequences for directed graphs. A walk profile encompasses numerous structural features crucial for directed graph-relevant applications, such as program analysis and circuit performance prediction. We identify the limitations of existing PE methods in representing walk profiles and propose a novel *Multi-q Magnetic Laplacian PE*, which extends the Magnetic Laplacian eigenvector-based PE by incorporating multiple potential factors. The new PE can provably express walk profiles. Furthermore, we generalize prior basis-invariant neural networks to enable the stable use of the new PE in the complex domain. Our numerical experiments validate the expressiveness of the proposed PEs and demonstrate their effectiveness in solving sorting network satisfiability and performing well on general circuit benchmarks.
What Matters in Learning from Large-Scale Datasets for Robot Manipulation
Vaibhav Saxena, Matthew Bronars, Nadun Ranawaka Arachchige, Kuancheng Wang, Woo Shin, Soroush Nasiriany, Ajay Mandlekar, Danfei Xu
Abstract
Imitation learning from large multi-task demonstration datasets has emerged as a promising path for building generally-capable robots. As a result, 1000s of hours have been spent on building such large-scale datasets around the globe. Despite the continuous growth of such efforts, we still lack a systematic understanding of what data should be collected to improve the utility of a robotics dataset and facilitate downstream policy learning. In this work, we conduct a large-scale dataset composition study to answer this question. We develop a data generation framework to procedurally emulate common sources of diversity in existing datasets (such as sensor placements and object types and arrangements), and use it to generate large-scale robot datasets with controlled compositions, enabling a suite of dataset composition studies that would be prohibitively expensive in the real world. We focus on two practical settings: (1) what types of diversity should be emphasized when future researchers collect large-scale datasets for robotics, and (2) how should current practitioners retrieve relevant demonstrations from existing datasets to maximize downstream policy performance on tasks of interest. Our study yields several critical insights — for example, we find that camera poses and spatial arrangements are crucial dimensions for both diversity in collection and alignment in retrieval. In real-world robot learning settings, we find that not only do our insights from simulation carry over, but our retrieval strategies on existing datasets such as DROID allow us to consistently outperform existing training strategies by up to 70\%.
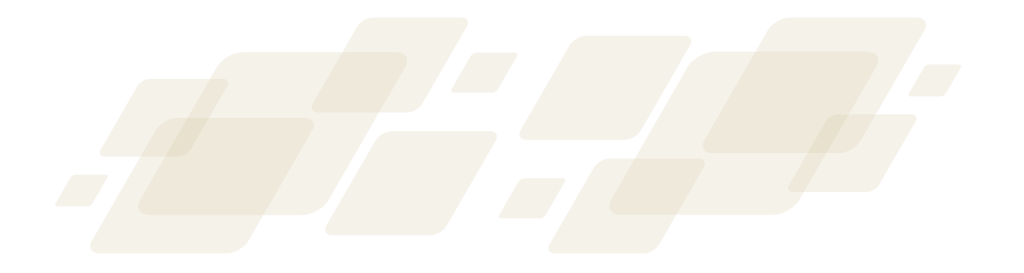
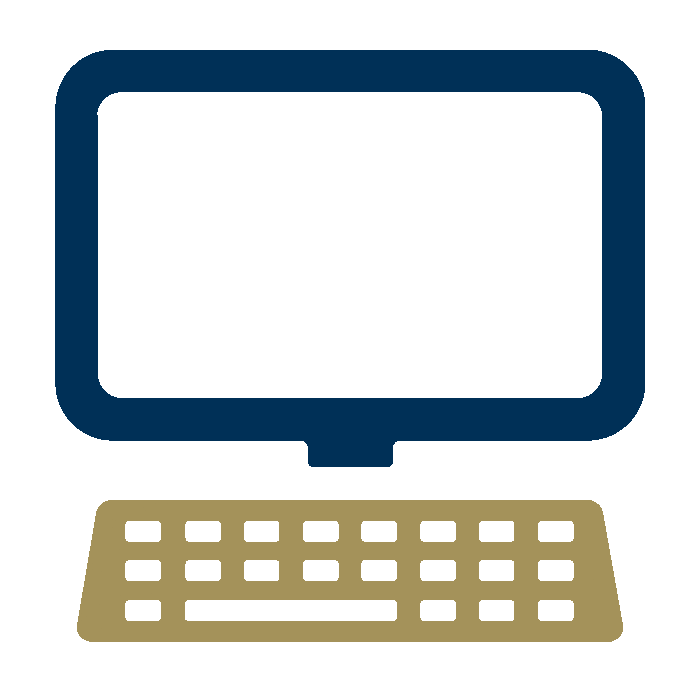
See you in Singapore!
Development: College of Computing
Project and Web Lead/Data Graphics: Joshua Preston
News: Joshua Preston and Bryant Wine
Featured Photography: Bryant Wine Profile Photos: Kevin Beasley, Terence Rushin, and submitted photos
Data: https://iclr.cc/ Additional data collection/formatting: Joni Isbell